M.S. in Data Science students are required to complete a capstone project. Capstone projects challenge students to acquire and analyze data to solve real-world problems. Project teams consist of two to four students and a faculty advisor. Teams select their capstone project at the beginning of the year and work on the project over the course of two semesters.
Most projects are sponsored by an organization—academic, commercial, non-profit, and government—seeking valuable recommendations to address strategic and operational issues. Depending on the needs of the sponsor, teams may develop web-based applications that can support ongoing decision-making. The capstone project concludes with a paper and presentation.
Key takeaways:
- Synthesizing the concepts you have learned throughout the program in various courses (this requires that the question posed by the project be complex enough to require the application of appropriate analytical approaches learned in the program and that the available data be of sufficient size to qualify as ‘big’)
- Experience working with ‘raw’ data exposing you to the data pipeline process you are likely to encounter in the ‘real world’
- Demonstrating oral and written communication skills through a formal paper and presentation of project outcomes
- Acquisition of team building skills on a long-term, complex, data science project
- Addressing an actual client’s need by building a data product that can be shared with the client
Capstone projects have been sponsored by a variety of organizations and industries, including: Capital One, City of Charlottesville, Deloitte Consulting LLP, Metropolitan Museum of Art, MITRE Corporation, a multinational banking firm, The Public Library of Science, S&P Global Market Intelligence, UVA Brain Institute, UVA Center for Diabetes Technology, UVA Health System, U.S. Army Research Laboratory, Virginia Department of Health, Virginia Department of Motor Vehicles, Virginia Office of the Governor, Wikipedia, and more.
View previous examples of capstone projects and check out answers to frequently asked questions.
What does the process look like?
- The School of Data Science periodically puts out a Call for Proposals. Prospective project sponsors submit official proposals, vetted by the Associate Director for Research Development, Capstone Director, and faculty.
- Sponsors present their projects to students at “Pitch Day” near the start of the Fall term, where students have the opportunity to ask questions.
- Students individually rank their top project choices. An algorithm sorts students into capstone groups of approximately 3 to 4 students per group.
- Adjustments are made by hand as necessary to finalize groups.
- Each group is assigned a faculty mentor, who will meet groups each week in a seminar-style format.
What is the seminar approach to mentoring capstones?
We utilize a seminar approach to managing capstones to provide faculty mentorship and streamlined logistics. This approach involves one mentor supervising three to four loosely related projects and meeting with these groups on a regular basis. Project teams often encounter similar roadblocks and issues so meeting together to share information and report on progress toward key milestones is highly beneficial.
Do all capstone projects have corporate sponsors?
Not necessarily. Generally, each group works with a sponsor from outside the School of Data Science. Some sponsors are corporations, some are from nonprofit and governmental organizations, and some are from in other departments at UVA.
One of the challenges we continue to encounter when curating capstone projects with external sponsors is appropriately scoping and defining a question that is of sufficient depth for our students, obtaining data of sufficient size, obtaining access to the data in sufficient time for adequate analysis to be performed and navigating a myriad of legal issues (including conflicts of interest). While we continue to strive to use sponsored projects and work to solve these issues, we also look for ways to leverage openly available data to solve interesting societal problems which allow students to apply the skills learned throughout the program. While not all capstones have sponsors, all capstones have clients. That is, the work is being done for someone who cares and has investment in the outcome.
Why do we have to work in groups?
Because data science is a team sport!
All capstone projects are completed by group work. While this requires additional coordination , this collaborative component of the program reflects the way companies expect their employees to work. Building this skill is one of our core learning objectives for the program.
I didn’t get my first choice of capstone project from the algorithm matching. What can I do?
Remember that the point of the capstone projects isn’t the subject matter; it’s the data science. Professional data scientists may find themselves in positions in which they work on topics assigned to them, but they use methods they enjoy and still learn much through the process. That said, there are many ways to tackle a subject, and we are more than happy to work with you to find an approach to the work that most aligns with your interests.
Your ability to influence which project you work on is in the ranking process after “pitch day” and in encouraging your company or department to submit a proposal during the Call for Proposal process. At a minimum it takes several months to work with a sponsor to adequately scope a project, confirm access to the data and put the appropriate legal agreements into place. Before you ever see a project presented on pitch day, a lot of work has taken place to get it to that point!
Can I work on a project for my current employer?
Each spring, we put forward a public call for capstone projects. You are encouraged to share this call widely with your community, including your employer, non-profit organizations, or any entity that might have a big data problem that we can help solve. As a reminder, capstone projects are group projects so the project would require sufficient student interest after ‘pitch day’. In addition, you (the student) cannot serve as the project sponsor (someone else within your employer organization must serve in that capacity).
If my project doesn’t have a corporate sponsor, am I losing out on a career opportunity?
The capstone project will provide you with the opportunity to do relevant, high-quality work which can be included on a resume and discussed during job interviews. The project paper and your code on Github will provide more career opportunities than the sponsor of the project. Although it does happen from time to time, it is rare that capstones lead to a direct job offer with the capstone sponsor's company. Capstone projects are just one networking opportunity available to you in the program.
Capstone Project Reflections From Alumni
"For my Capstone project, I used Python to train machine learning models for visual analysis – also known as computer vision. Computer vision helped my Capstone team analyze the ergonomic posture of workers at risk of developing musculoskeletal injuries. We automated the process, and hope our work further protects the health and safety of people working in the United States.”
— Theophilus Braimoh, MSDS Online Program 2023, Admissions Student Ambassador
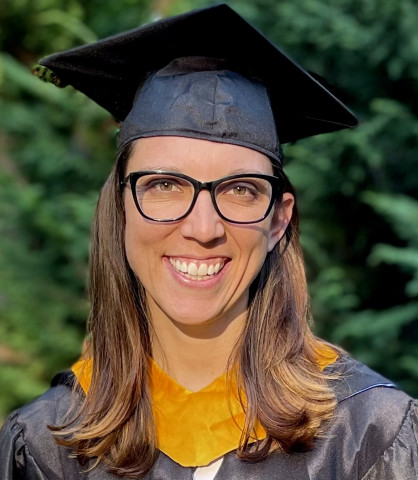
“My Capstone experience with the ALMA Observatory and NRAO was a pivotal chapter in my UVA Master’s in Data Science journey. It fostered profound growth in my data science expertise and instilled a confidence that I'm ready to make meaningful contributions in the professional realm.”
— Haley Egan, MSDS Online Program 2023, Admissions Student Ambassador
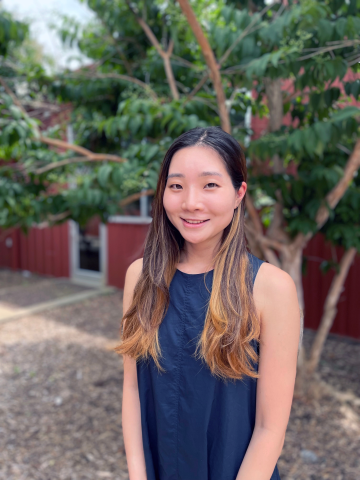
“Our Capstone projects gave us the opportunity to gain new domain knowledge and answer big data questions beyond the classroom setting.”
— Mina Kim, MSDS Residential Program 2023, Ph.D. in Psychology Candidate
Capstone Project Reflections From Sponsors
“For us, the level of expertise, and special expertise, of the capstone students gives us ‘extra legs’ and an extra push to move a project forward. The team was asked to provide a replicable prototype air quality sensor that connected to the Cville Things Network, a free and community supported IoT network in Charlottesville. Their final product was a fantastic example that included clear circuit diagrams for replication by citizen scientists.”
— Lucas Ames, Founder, Smart Cville
“Working with students on an exploratory project allowed us to focus on the data part of the problem rather than the business part, while testing with little risk. If our hypothesis falls flat, we gain valuable information; if it is validated or exceeded, we gain valuable information and are a few steps closer to a new product offering than when we started.”
— Ellen Loeshelle, Senior Director of Product Management, Clarabridge