Master’s Students Strengthen Ability of LLM to Recommend Scholarly Works
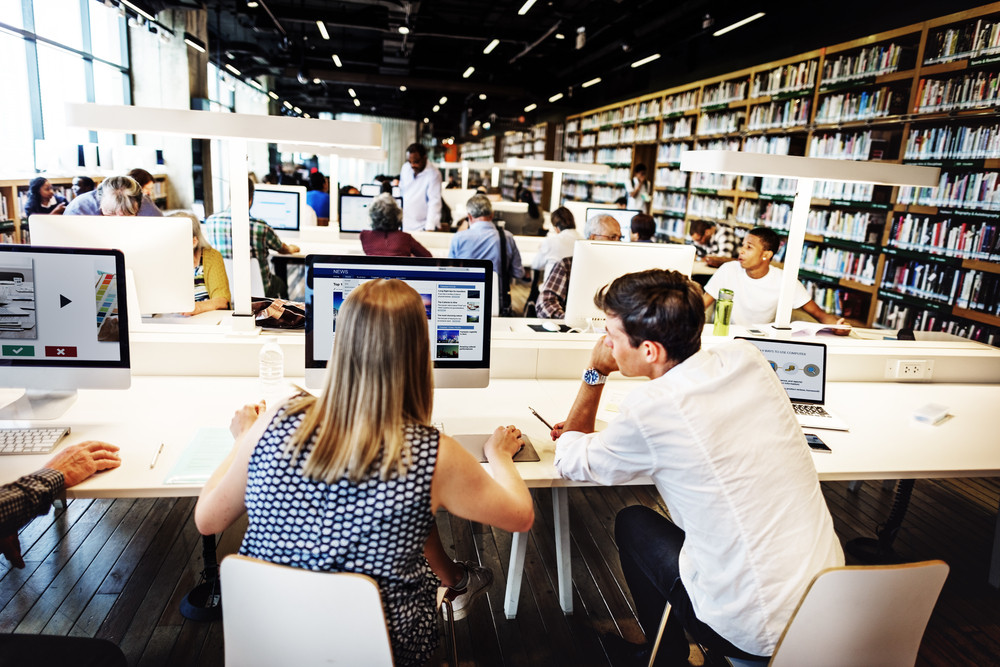
Like other content consumers, readers of scholarly articles sometimes need guidance to find works that best match their interests.
Hum, a company that uses artificial intelligence and data to help publishers better serve their clients, developed a large language model, which they called Lodestone, capable of interpreting lengthy academic texts and generating user recommendations.
The continual evolution of LLMs, though, requires even high-performing models to be fine-tuned. To that end, Hum enlisted the help of four UVA data science master’s students — Brook Tarekegn Assefa, Kyler Halat-Shafer, Kristen Rose, and Dominic Scerbo — to take on this task as part of their capstone project.
Capstone projects have long been an integral component of both the residential and online data science master’s programs. In them, small groups of students work with a faculty mentor and an external sponsor to tackle a real-world data challenge — then present their findings to fellow students and faculty shortly before graduation.
“We enjoyed working with these students and the UVA program as a whole. Helping them place their technical skills into a business context is rewarding and a really fun growth phase to watch,” said Will Fortin, lead data scientist at HUM.
Under the guidance of Philip Waggoner from the School of Data Science, Assefa, Halat-Shafer, Rose, and Scerbo set out to enhance Hum’s existing model, given the constant improvements to LLM technology.
“Large language models have really boomed,” Rose said during the group’s presentation. “New and better models are coming out every day.”
The group used information from OpenAlex, a catalog of global research that is fully accessible to the public. They focused on the data that would be key to completing their project: article titles, abstracts, and topics.
Scerbo explained how the group employed a process where an interpretive AI model transformed scholarly research and third-party data into a mathematical vector representation, while also using a fine-tuning process to make further improvements. The team introduced training pairs to strengthen their model's capabilities to understand academic language.
“Using a state-of-the-art model, this allows us to increase the precision of the contextualized word embeddings, thus improving the relevancy of the predictive capability within Hum’s platform,” Scerbo said.
The group developed a new benchmark, tested the model against internal and industry-standard benchmarks, and outlined their results during their presentation. One key metric illustrated the potential of their model. The group looked at the difference between what their fine-tuned model recommended for users and the content they consumed.
While the actual percentage improvement from the use of their model may have been small, the impact could be profound.
“For every fraction of percentage improvement, it’s an improved result for thousands or even tens of thousands of users daily,” said Assefa.
Not long after successfully presenting their findings, the group members all graduated with data science master’s degrees. Their work on the project will undoubtedly prove beneficial to both readers of scholarly articles and to themselves as they take the next step in their data science careers.
“Overall, each of us are walking away from this experience with a treasure trove of knowledge,” said Halat-Shafer.