Student Capstone Project Looks To Improve Electrolarynx Speech-to-Text
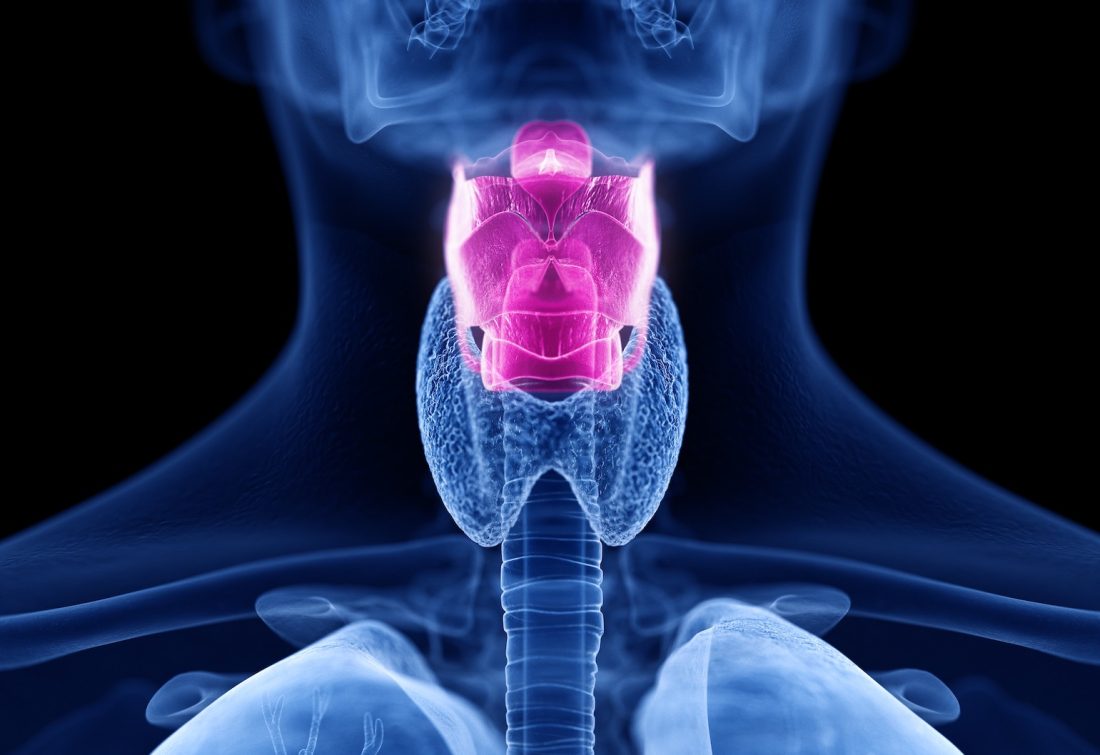
A team of M.S. in Data Science Online students partnered with the University of Virginia Otolaryngology Department on a capstone project to explore a deep-learning model that could convert electrolarynx audio to readable text reliably and quickly, improving communication across telemedicine and video for patients and their health providers.
Why is this important? More than 3,000 people in the United States each year undergo a laryngectomy, often due to life-threatening conditions brought on by cancer. The loss of speech makes it especially difficult for patients to communicate, especially with their medical teams during crucial stages in their treatment.
Voice restoration therapies do exist, and an electrolarynx is a common solution—a non-invasive and relatively inexpensive handheld device pressed against the throat that emulates vocal cords using vibrations. The electrolarynx is not without its flaws as it is difficult to manipulate and understand due to its monotone output. Usage over the phone or video puts it at an even greater disadvantage.
“This project is a first step in a larger effort towards helping these patients,” said Will Johnson, one of the students working on the project. “If it’s hard to understand someone’s words with [an electrolarynx] in person, imagine the challenges they face with a Teladoc appointment.”
The capstone project sponsored by the UVA Otolaryngology Department was a continuation of research done last year by students in the engineering school who reduced video data to four points on the lip to predict patient speech. “As data scientists, we explored what we could do with the data itself,” said another student, Chris Lee. “With the guidance of our mentor Yuri Malitsky, we manipulated existing and synthesized data to improve a deep learning model that could improve speech-to-text performance.
Extensive research exists on speech-to-text (STT) models using machine learning and artificial intelligence, but little has been done for patients who have lost their larynx. The capstone team was challenged to research STT models for patients using an electrolarynx (EL) and was given over 300 video files which they parsed into 477 audio samples. They supplemented the data with more than 13,000 audio clips from the open-source LJ speech dataset.
“EL speech is very different from normal speech,” the team wrote in their capstone research paper. “Its unique acoustic properties make it difficult to develop effective STT models. The lack of airflow required to speak means users have challenges reproducing distinctions between consonants and vowel sounds.”
They pointed out that the characteristics of EL speech that humans find difficult to understand make it even more difficult for computers to understand. “Given how impressive recent advancements in AI have been,” said Johnson, “one might think this task should have been accomplished by now.”
The student team, which also included Mani Shanmugavel, compared several data sets against their benchmark STT model trained and run on normal speech. They looked at the following:
Out of the Box – Run a pre-trained STT model on EL data
EL Dataset – Train a new STT model using only EL data
Denoised Data – Train a denoiser and run on EL data before STT training
Synthetic Dataset – Emulate EL data on a larger dataset
EL + Synthetic Dataset – Train on both EL and synthetic data, validate on EL alone
The conclusion? The team reported that while their research does not return a practical model for EL transcription, they believed the results suggested that “additional data collection, design changes, and experimentation may yield a model that can work for patients.
According to Johnson, the problem isn’t AI’s design but rather the lack of data. “More inclusive data mining practices will improve upon the work we started.”
Lee agrees. “It would be great if more data could be collected so these models could improve enough to be used in real-time, in real patient interactions.”
The team is optimistic. Given more data, real breakthroughs using AI to improve electrolarynx speech-to-text could be made and would vastly improve patients' experience and therefore the care they receive.
Capstone projects like this one are an integral and required part of the M.S. in Data Science experience. They challenge students in the residential or online program to acquire and analyze data to solve real-world problems. Most projects are sponsored by an organization—academic, commercial, non-profit, and government—seeking valuable recommendations to address strategic and operational issues. Students work closely with the sponsor and faculty lead to deliver actionable recommendations to the project challenge in the form of a paper and presentation.