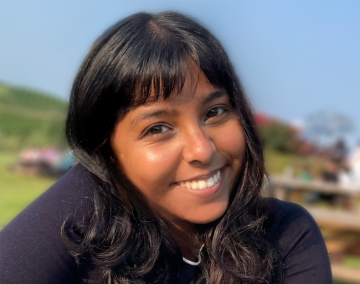
Data Science Across the Globe: Ph.D. Student Shares Insights from Uzbekistan Conference
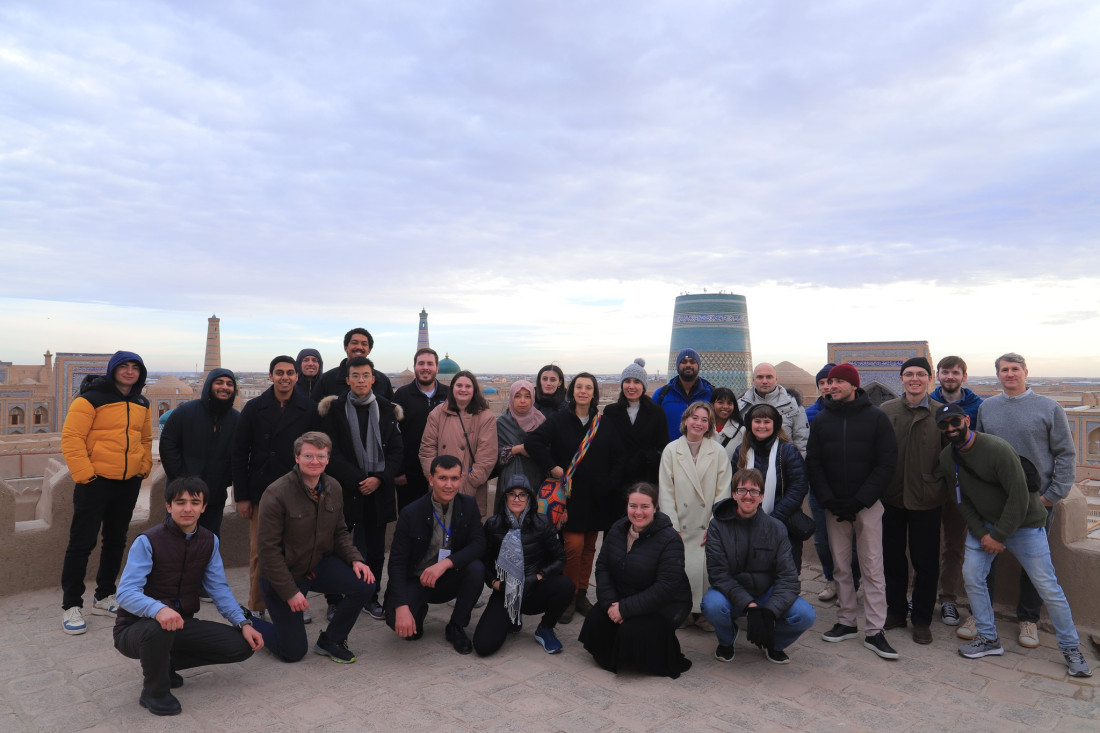
I recently was part of a select group of students and lecturers to attend the Advanced Studies Institute in Mathematics of Data Science & Machine Learning international conference at Urgench State University in Uzbekistan. I learned about the experience from an open call for student applicants through the National Science Foundation.
Organized by the USA-Uzbekistan Collaboration for Research, Education, and Student Training, one emphasis of the conference was on formalizing mathematical definitions in data science and connecting them to present-day research, which sparked my interest in attending.
My favorite lecture was one that introduced a concept known as “benign overfitting” as a positive characteristic of machine learning. The traditional attitude toward model overfitting — which is very closely related to the bias-variance tradeoff — is that it is viewed negatively.
The reason for that perception is that while it may generalize well on training data, model overfitting usually performs poorly on unseen data. However, recent research shows that this is not always the case. In fact, you can implement overparameterization — which occurs when you purposely include more training features than number samples — to have good performance both on training and new data.
This lecture provided a great reminder to consider the exceptions to a rule, even when that rule is the classical approach to prediction.
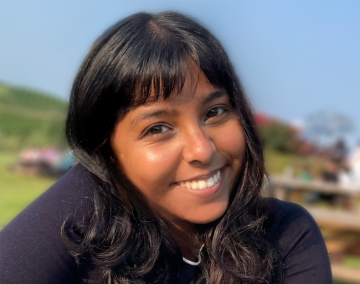
Another takeaway from the conference was how sobering it was to see how critical currents of information and research are carried across borders. We had lecturers and students from institutions around the world and an equally wide breadth of technical fields represented, from robotics to geospatial methods.
Data science and its methods were our common language, but no two participants applied it in the same way or area. It was fitting to have a data science and machine learning conference against the backdrop of Uzbekistan and the Silk Road since, historically, it was a critical juncture for cultural and idea exchange.
Throughout our trip, we were reminded of the rich history of scientific heritage in Central Asia. For instance, the terms “algebra” and “algorithm” derive from Arabic and treatises of mathematics written in Uzbekistan.
Finally, to students who might consider attending a conference like this, I would say what you learn outside the conference hall can be just as important as what you learn inside it. Conferences are unique in that you gain firsthand exposure to those central to your field’s trajectory. You can not only expand on what was previously discussed or lectured on, but also get a feel for where entire disciplines are moving toward — or away from.
This knowledge can be priceless in formulating relevant and well-structured research questions, especially in a field like data science with broad applicability.
Navya Annapareddy is a Ph.D. student at the School of Data Science. She previously received a master’s degree in data science from UVA as well as a bachelor’s degree in systems engineering from the University.