The Art of Data: Visualizing Different Data Problems
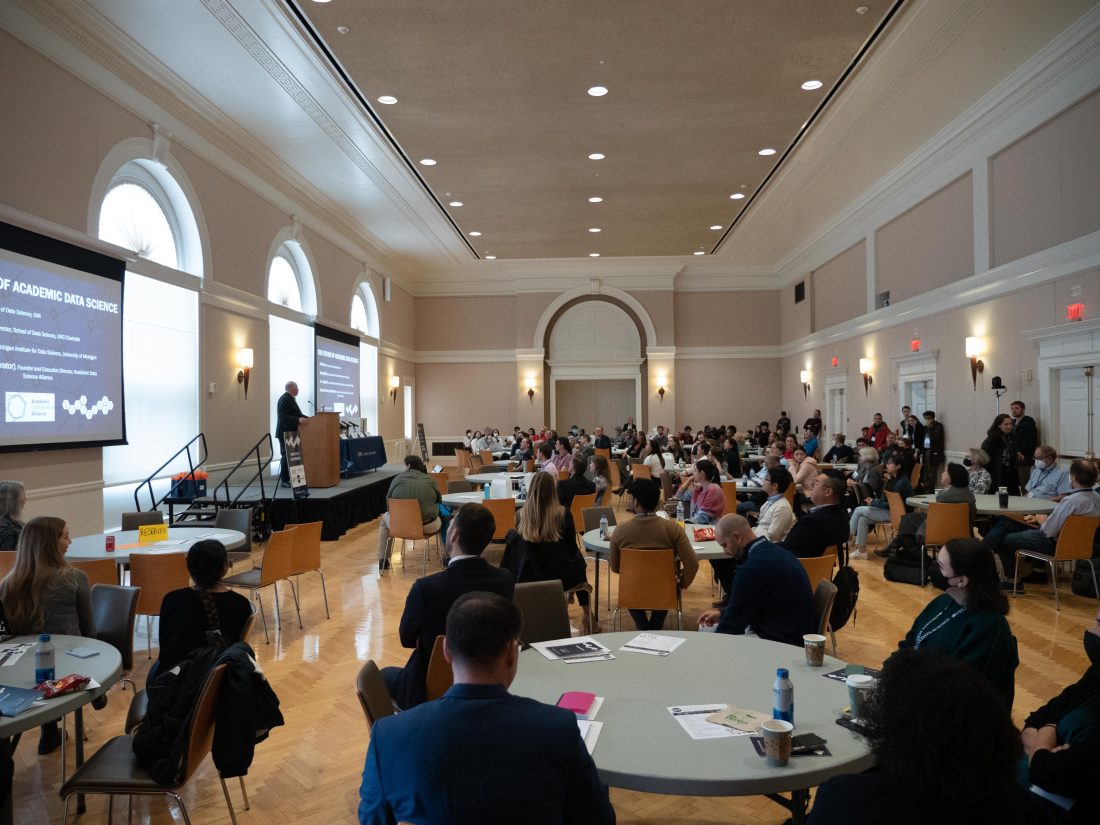
The Data of Design and Visualization Lightning talk at Datapalooza 2022 focused on how data can be represented in different contexts. There were a variety of topics discussed in the presentations that made the talk especially interesting to me. I personally enjoyed Alvitta Ottley’s presentation on different human interactions, and how those can be tracked. Alex Gates and Sara Giggs did an excellent job of discussing their topics of Networks and Human-Computer Interaction.
Assistant Professor at The School of Data Science Alex Gates started the lightning session off with a presentation on Networks. Gates explained that networks are all around us through most of our lives. Whether it was a social media network, a research network, or a road network. Professor Gates continued the discussion of how he and his lab started to investigate the interdisciplinarity of research during the grant writing process. He did this through assessing different research papers that were written and what topics those papers belonged to most. He then plotted the papers based on what other papers cited the first paper. This created a co-citation network, and the viewer was able to see what topics were cited in different disciplines. The color of the node indicated which subject the paper belonged to. The higher the concentration of a color near another color, the more interdisciplinarity these topics were to one another.
Next, Alvitta Ottley, Assistant Professor of Computer Science and Engineering at the University of Washington in St. Louis, discussed how their team’s work could try and predict which individuals would go to which webpages. Ottley elaborated on how human computer interaction needs to be seen as a spectrum where some tasks can be more human oriented, and some tasks are more computer oriented. I really liked this way of thinking because it seemed so realistic to what happens in different tasks in data science. She then continued to on how humans have been able to begin to predict human behavior, such as authenticating a user based off their keystrokes. Also, there has been research predicting someone’s personality based on the websites they visited. I also thought that these two discoveries were interesting because these two different things feel very different. Finally, Ottley stressed the importance of, when making algorithm recommendations, understanding that humans can bring their own biases into these algorithms.
Lastly, Sara Riggs, Associate Professor in the Engineering School at the University of Virginia, discussed how better context and explanation is necessary for AI algorithms. Riggs discussed how different industries or different fields of study might need more explanation as to how an algorithm decided to take the course of action it did. There are certain contexts in which many of the fields need further explanation, such as the government and medicine, as to why an algorithm decided to choose the course of action that it did. There are cases in which the effects of an automated decision by an algorithm are vastly different such as a recommendation algorithm on a social media outlet vs. an algorithm that decides what a UAV should target. Thus, the two cases require very different explanations, and the magnitude of the explanation is very different.
Overall, all three presentations were very engaging and enlightening. I appreciated the time for questions at the end, and the presenters were thoughtful and responsive.