Credit card fraud costs billions of dollars annually, increasing the incentive among financial institutions to develop fast, effective and dynamic fraud detection systems.
Researchers Navin Kasa, Andrew Dahbura, and Charishma Ravoori undertook a capstone project—part of the Master of Science in Data Science program—that addresses credit card fraud detection through a semi-supervised approach, in which clusters of account profiles are created and used for modeling classifiers. Accounts are profiled based on their behavioral trends and clustered into similar groups. Groups are further identified as distinct customer segments based on purchase characteristics such as amount, frequency or distance.
The primary question of this research investigates whether clustering helps improve the predictive performance of credit card fraud.
By engineering useful and descriptive features at the account level, the researchers hypothesized that clustering would be able to separate accounts into meaningful clusters that will improve prediction capabilities. Two baseline models without clustering were generated for comparison against cluster specific models.
Results highlight the potential for optimal classifiers to vary by cluster, suggesting that these classifiers may boost overall fraud detection performance when evaluated using clustering. Additionally, account and transaction characteristics of each cluster should be investigated further to help understand what features are useful in dividing customers. Specifically, clusters that cannot be differentiated must be investigated further to better understand their customer behaviors.
If banks can understand groups of consumers where models perform better or worse, they can begin to investigate and engineer new features that may be more useful.
Further research could investigate whether reassigning accounts in underperforming clusters helps improve performance. It is possible that accounts on the fringe of two customer groups share characteristics that may be useful in predicting fraud when looked at jointly, but are missed by the current model. This also presents the task of determining when accounts should be assigned to new clusters as their behavioral patterns change over time.
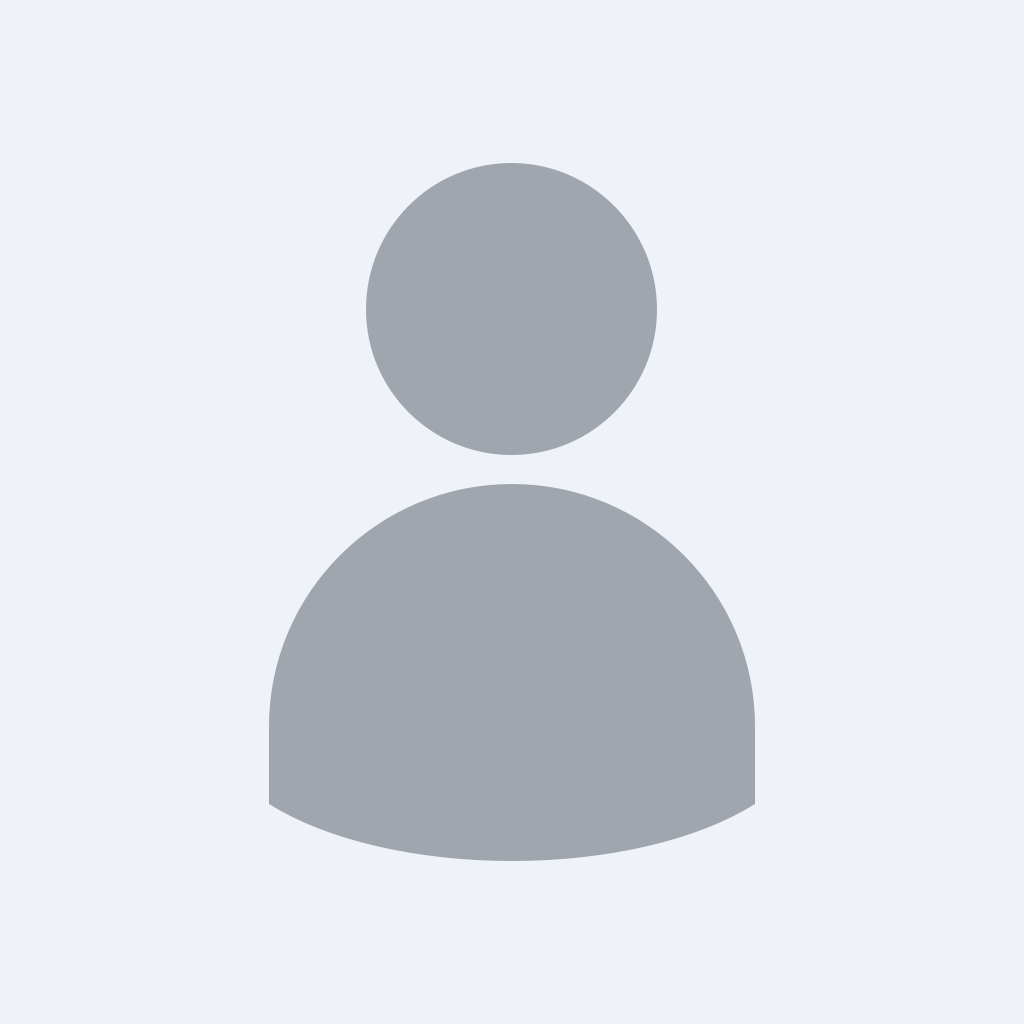