The Market Intelligence division of S&P Global provides annual multiyear forecasts of U.S. subscribers for the video industry, which is comprised of cable, satellite, and telecommunication service providers.
The current forecasts leave room for improvement: They are labor-intensive to generate and can be influenced by biases of subject-matter experts.
The focus of this project is to explore the application of machine learning methods to these forecasts. MSDS students Jordan Baker, Andrew Pomykalski and Kaley Hanrahan used demographic and subscriber data to assess the accuracy of different models, splitting it into training, validation, and testing sets.
This initial exploration into machine learning shows promise and indicates it can be used as a tool to enhance S&P’s current forecasting techniques. The industry is continuing to change as new technologies create disruption in the marketplace, which necessitates continued research into forecasting within this field.
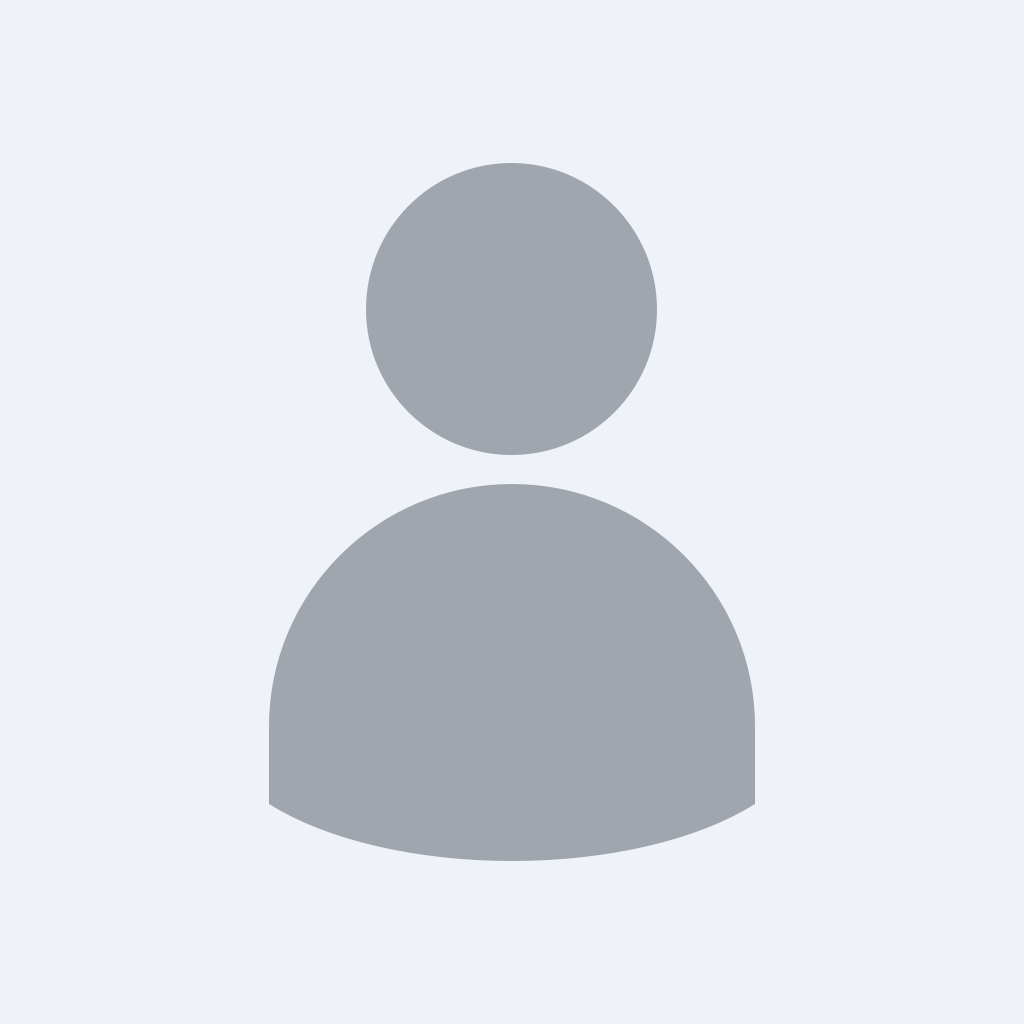
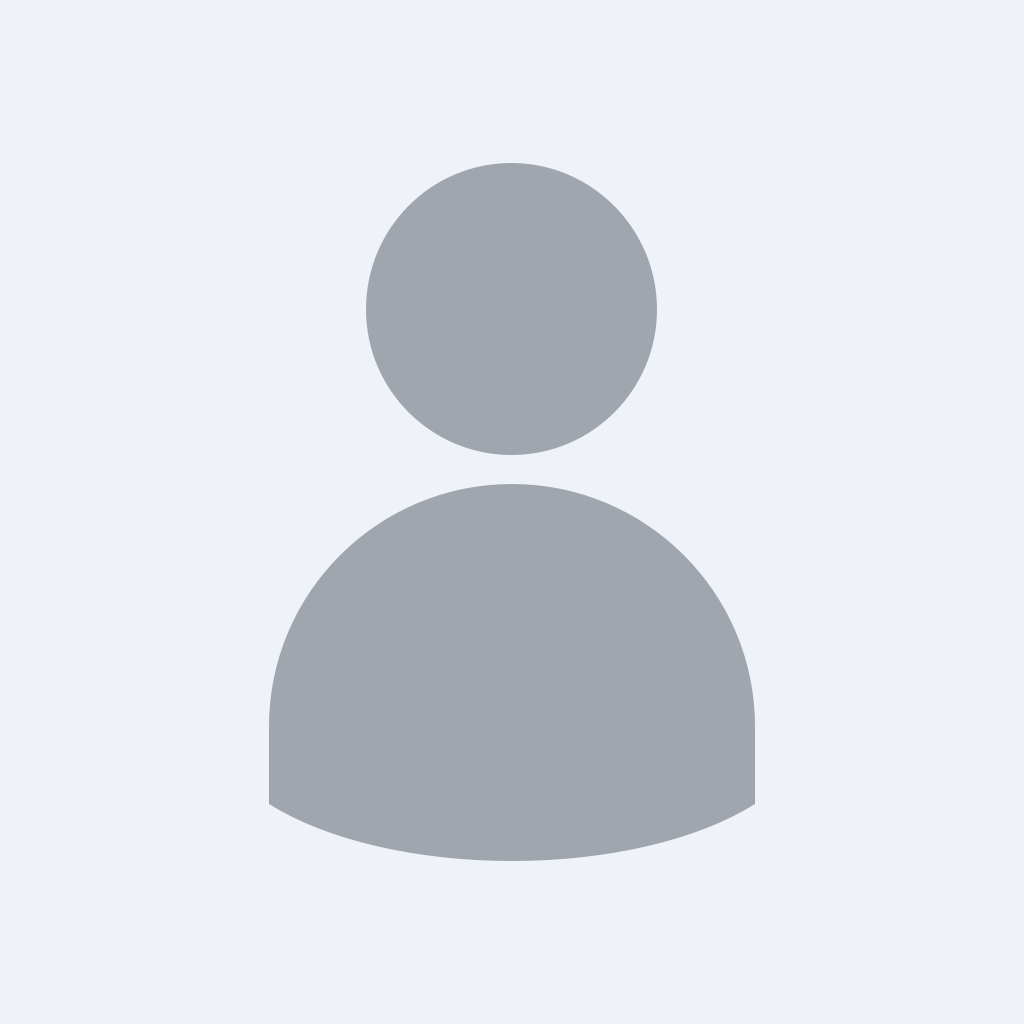
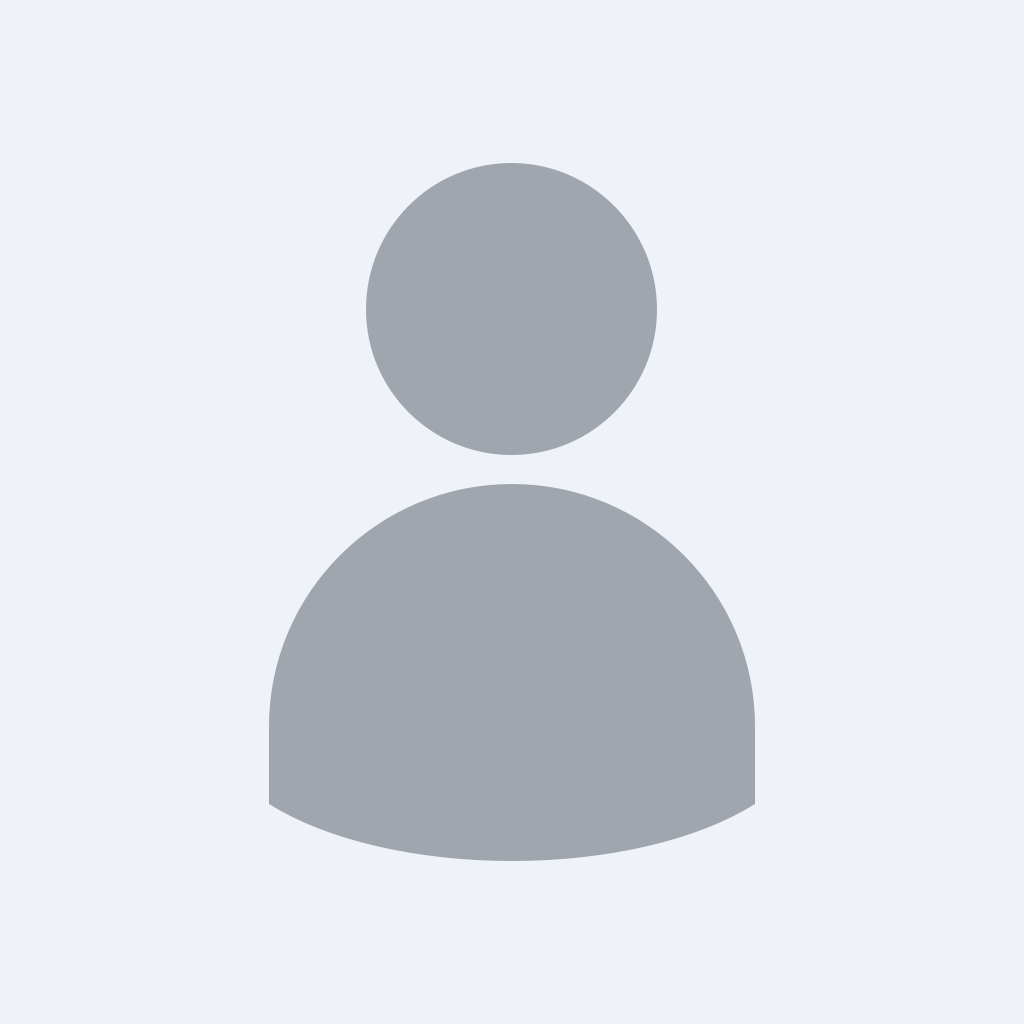