Bicycle use in cities has clear social and environmental benefits, but encouraging bike-riding requires good city planning. Good planning, in turn, requires continuous observation of ridership patterns, which are difficult to collect.
This project aimed to find out how data on bicycle use can best be collected and analyzed, using existing technology. Modern traffic signals detect vehicles using video, but most cameras are trained only to spot large vehicles (cars and trucks).
Video data from traffic surveillance cameras to monitor bicycles via a simultaneous detection and tracking algorithm was gathered. The algorithm tracks bicycle movement by detecting “informative” subcomponents of bicycles and modeling their interactions and relationships using a robust machine learning algorithm. This helps overcome difficulties associated with moving/self-occluding parts and changes in view perspective.
The model the researchers have created enables detection and tracking of more generic objects and is applicable to more generic object detection and tracking.
The design and aspirations of this project have opened up a world of possibilities for better city planning, enabling tracking of all traffic on city streets and thus providing the data city planners need to design bike-friendly cities.
Emmanuel Denlove-Ito is a MS student in the Department of Electrical and Computer Engineering.
Alec Gosse is a PhD student in the Department of Civil and Environmental Engineering.
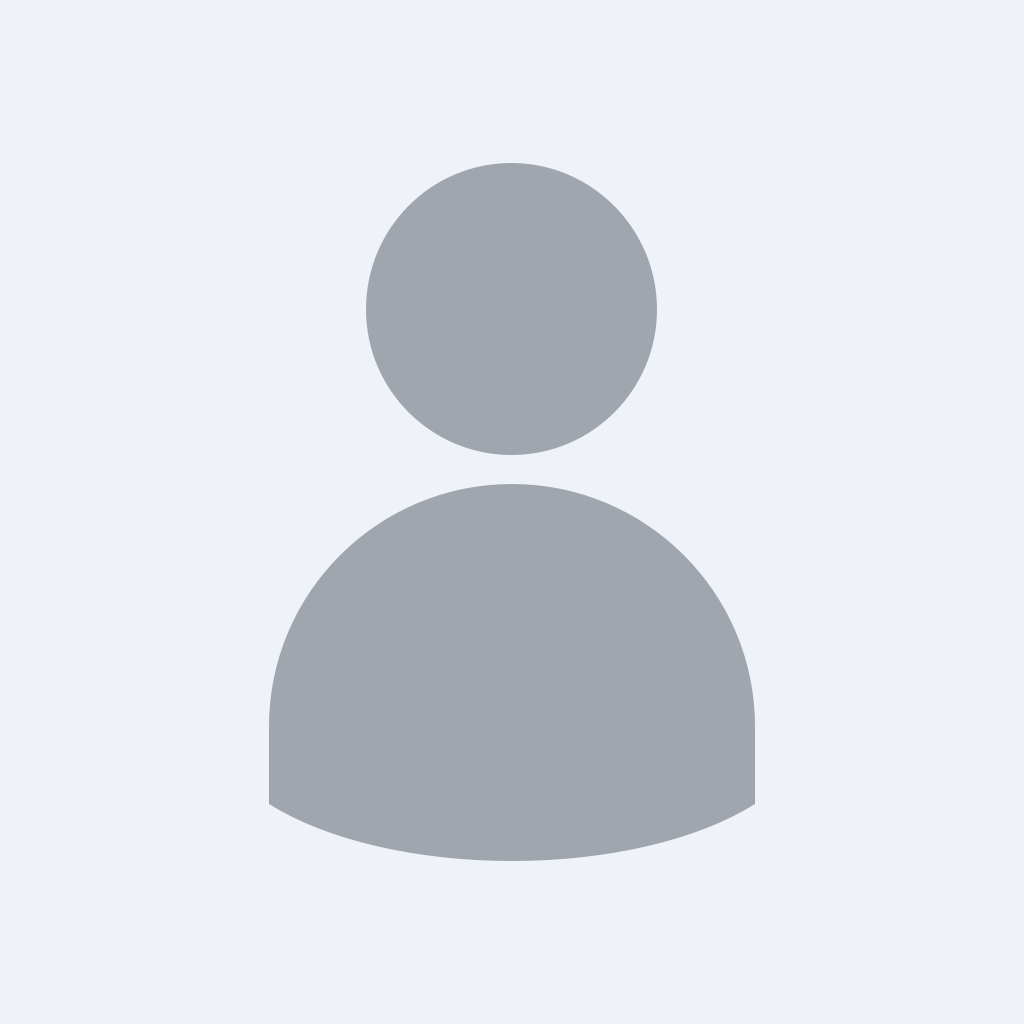
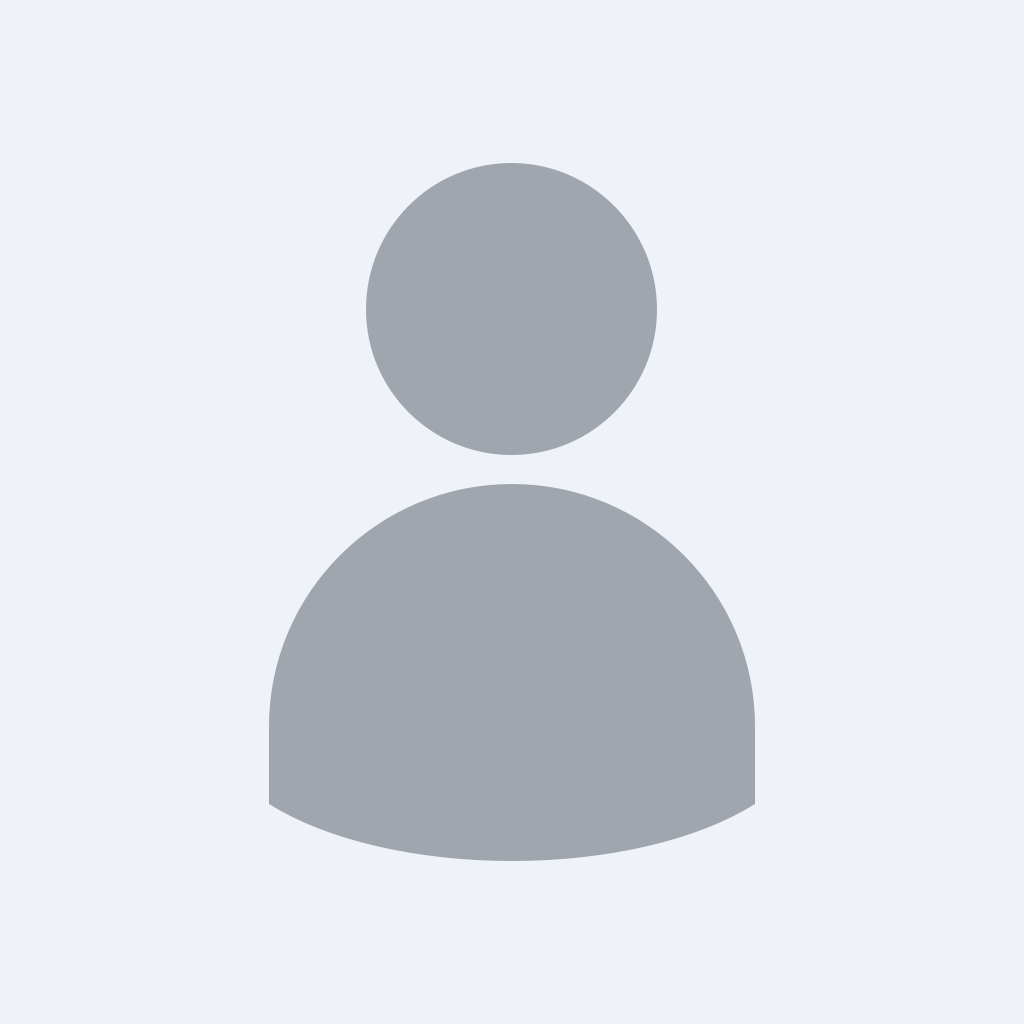