Knowing the exact three-dimensional position of each atom in a protein, known as its 3D structure, opens the door to a wealth of information—from deciphering evolution to designing better drugs.
Determining such 3D structures via experimental means, like X-ray crystallography, is quite costly in terms of time, money, and human resources—many PhDs have gone towards this task. Therefore, Master of Science in Data Science students Sean Mullane, Ruoyan Chen and Sri Vaishnavi Vemulapalli were motivated to apply data science tools and techniques to the problem, and see if protein structures can be quantitatively described, compared and otherwise analyzed in a more robust, efficient and automated manner. Potential applications include more effectively designed drugs to inhibit disease-related proteins, or even newly engineered ones.
The researchers received the award for Best Paper in the Data Science for Health category at the 2019 Systems & Information Design Symposium (SIEDS) meeting. Their project, "Machine Learning for Classification of Protein Helix Capping Motifs," focused on small segments of a protein called secondary structural elements. These structural elements are the basic molecular-scale building blocks that all proteins—and therefore life—build upon.
Protein segments are typically classified into discrete “secondary structures,” such as helices and sheets; historically, irregular structural patterns, such as ‘loops’, have been harder to study. Mullane, Chen and Vemulapalli devised and implemented a Deep Learning approach to classify the loop-like “end cap” structures which delimit helices (essentially, as start and stop signals). The team found that helix caps can be learned purely from a protein’s amino acid sequence (the string of chemical units that defines a protein chain), along with the specific geometric angles of the protein backbone, using a state-of-the-art deep neural network architecture known as a “bidirectional long short-term memory” (or BiLSTM) model.
In future work, they will focus on developing a related method (using ‘autoencoders’) to improve their model and design unsupervised models that might be able to ‘correct’ malformed predicted protein structures.
DeepMind’s recent AlphaFold success is a compelling example of the power of combining Deep Learning and protein structure analysis. In the near future, protein structures may well be more easily predicted from their simple sequences (versus laborious experimental determination). The Capstone Team’s success with helix caps illuminates what more might be possible in the years to come, including the use of Deep Learning to elucidate the detailed physical principles that underlie the structures of all proteins. Because protein function stems from 3D structure, such efforts hold the promise of deepening our knowledge of both normal and aberrant protein function, with ramifications for human health and disease.
The student team was supported by faculty advisors Phil Bourne, Cam Mura and Ke Wang, and PhD student Eli Draizen.
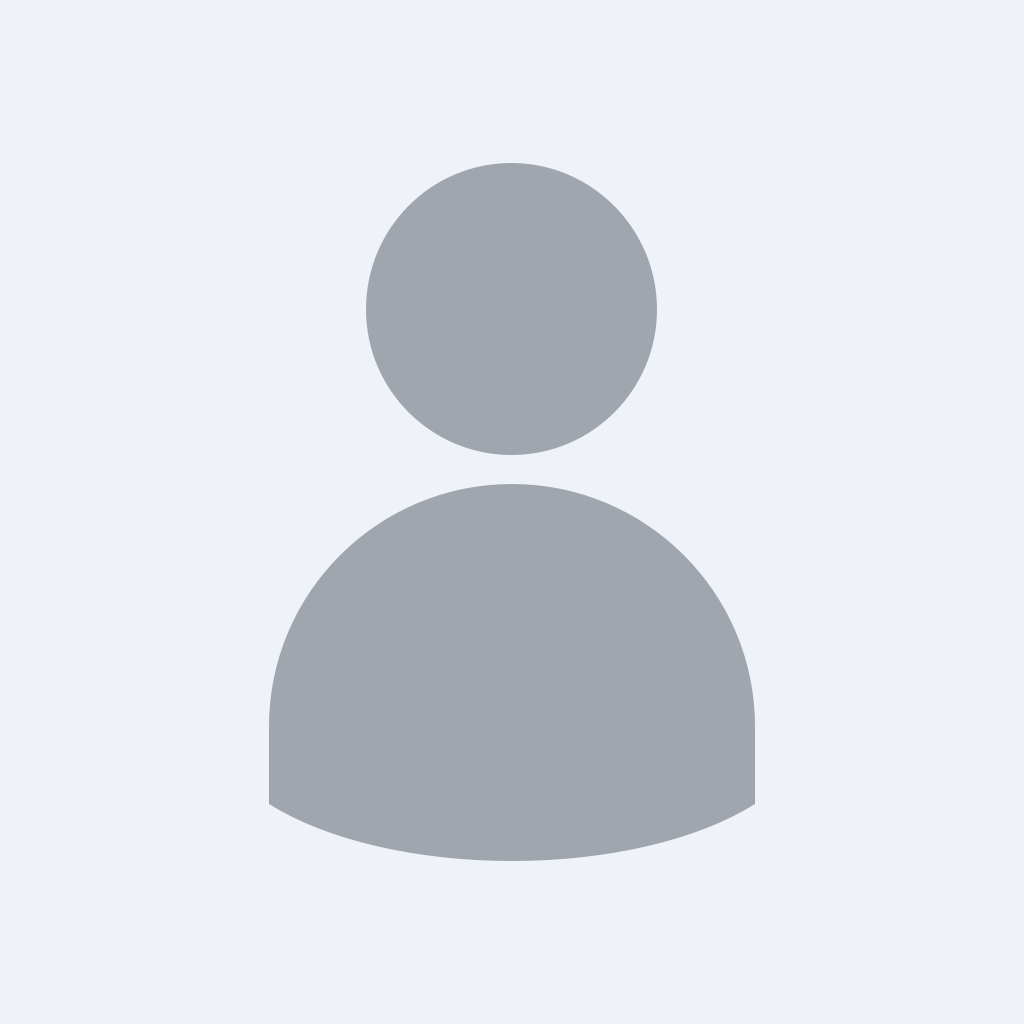
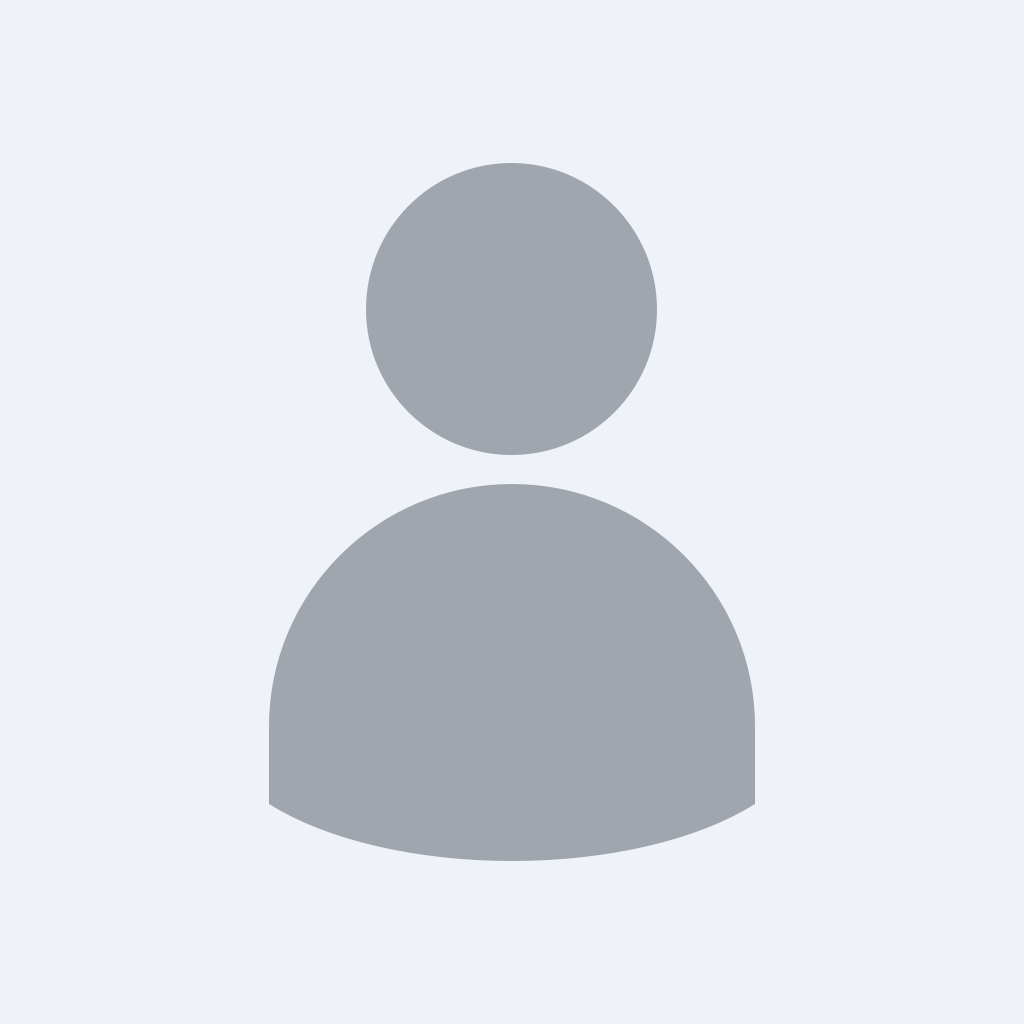
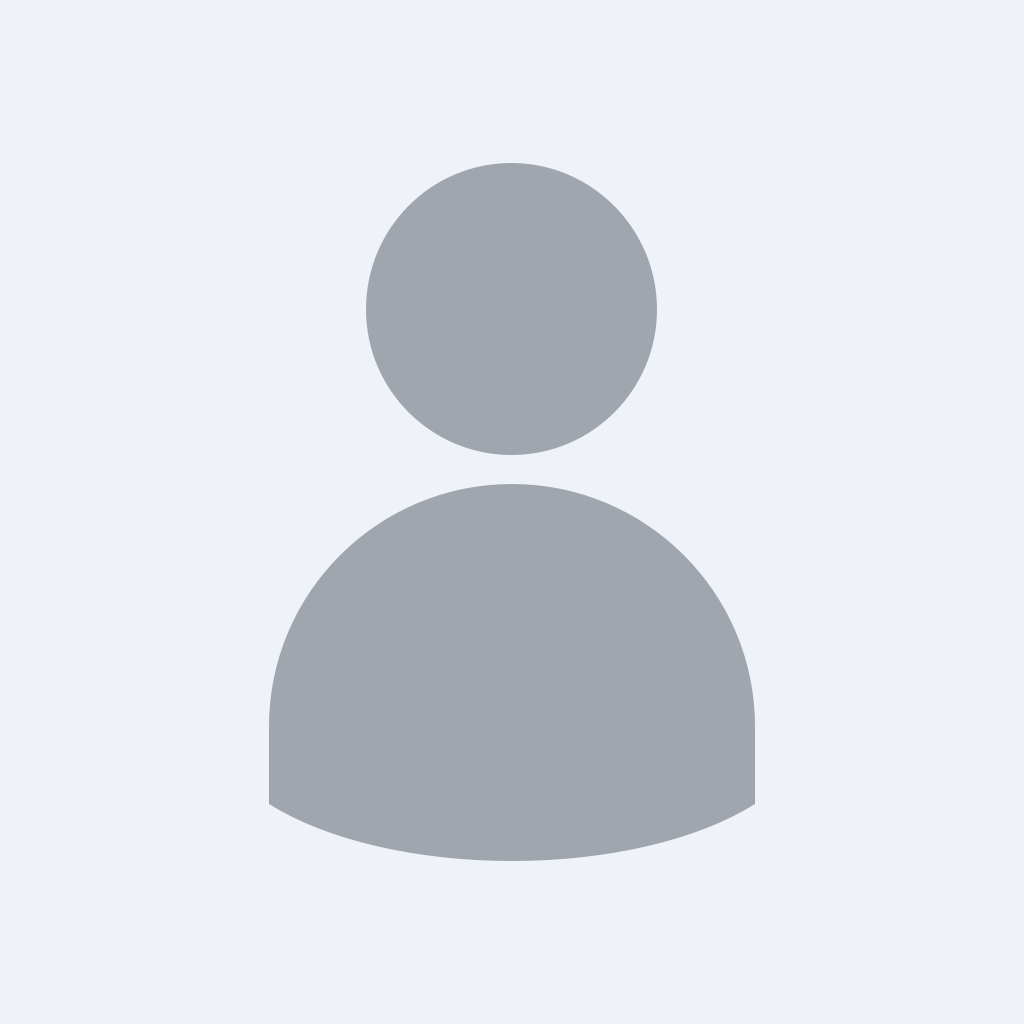
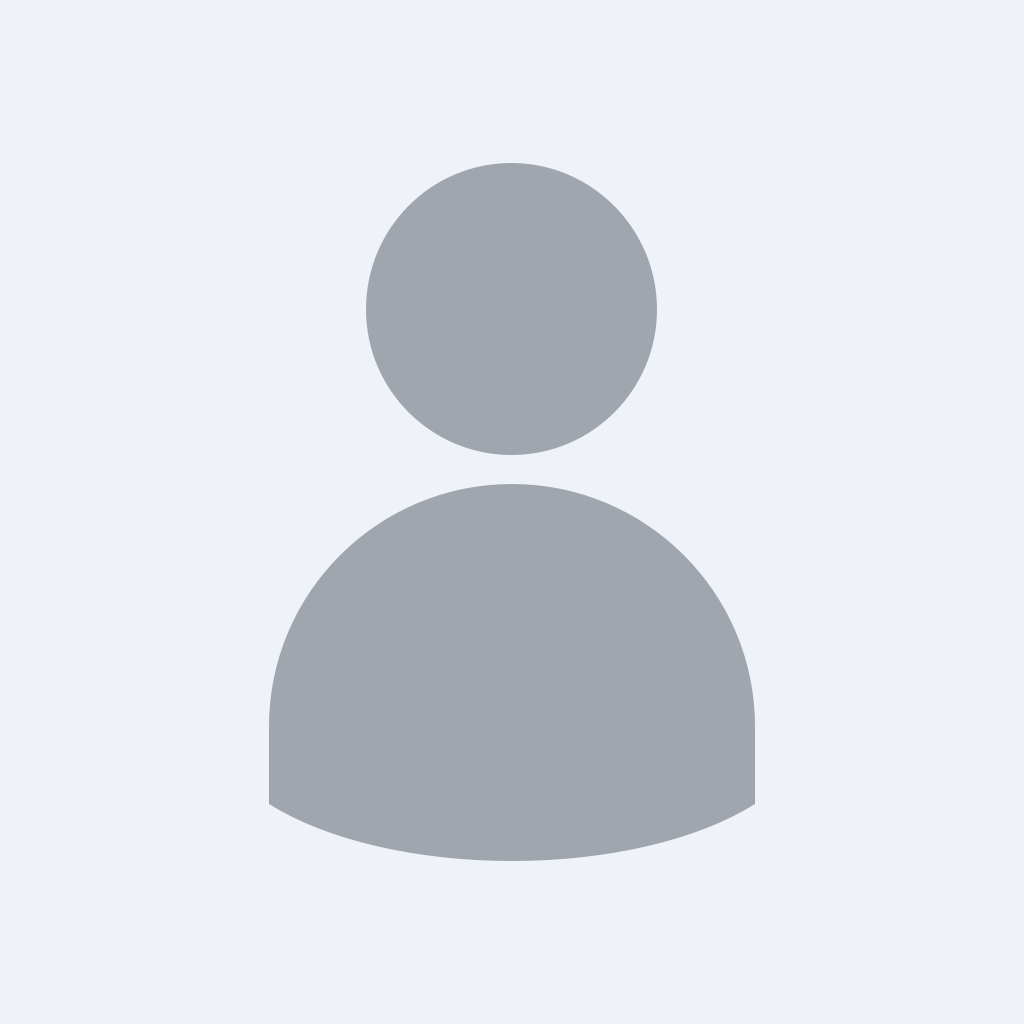