Robots are usually thought of as tools with purely functional movements. However, all movements contain expression, which also enhances function.
Lin Bai and Jon Bellona’s research was motivated by improving the perception of qualitative, expressive robotic movement. By capturing and analyzing human movement and sound data and studying the correspondences that articulate how humans move, they developed robotic movements synchronized with perceptually designed sounds as a way to make robotic movements more expressive and thus increase the level of human perception of quality in robotic movement. The improved feature variation of robotic movement makes robots more functional as well.
The project combined the fields of engineering and music to accomplish this task. The “timing” and “quality” features will be extracted from synchronized sound and movement data with control algorithms and signal analysis tools. Bai and Bellona studied how sonic features map in accordance with features of movement. By improving the available variation that is algorithmically defined in a robot’s movement while supplementing the movement with perceptually driven sounds, the project will enhance the quality and function of robotic movement.
These advancements will make robotic movements more expressive and relatable to human perception, enabling the possibility for robots to carry out more functions in more diverse fields, thus, making robots better tools.
Lin Bai is a PhD student in the Robotics, Automation, and Dance Lab and the Department of Electrical and Computer Engineering. Her research interests include control algorithm designs to ensure desired system performances, system modeling, optimization and estimation methods.
Jon Bellona is an intermedia artist and composer and a PhD student in composition and computer technologies in the Department of Music. His research includes data-driven control of electronic music performance, the construction of musical spaces for deep listening and collaboration with other disciplines to inform how we create musical experiences.
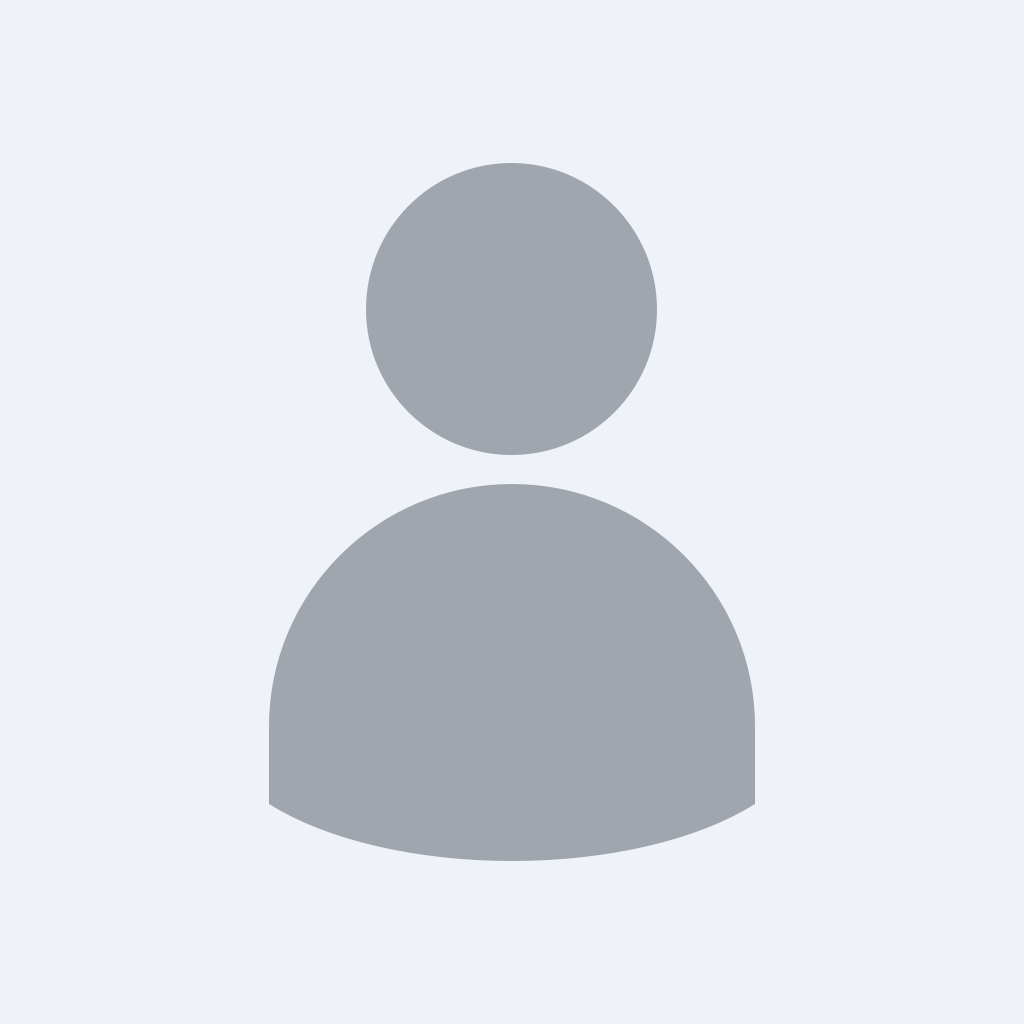
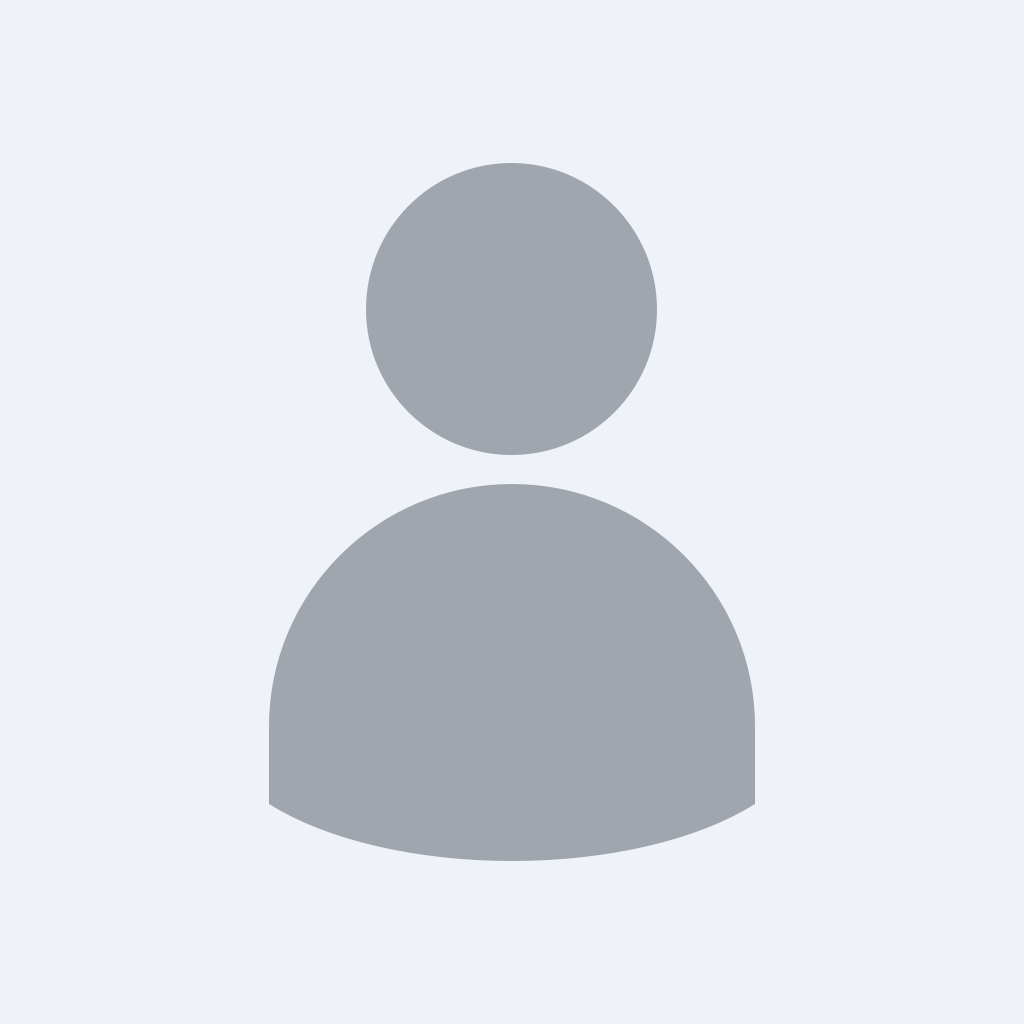
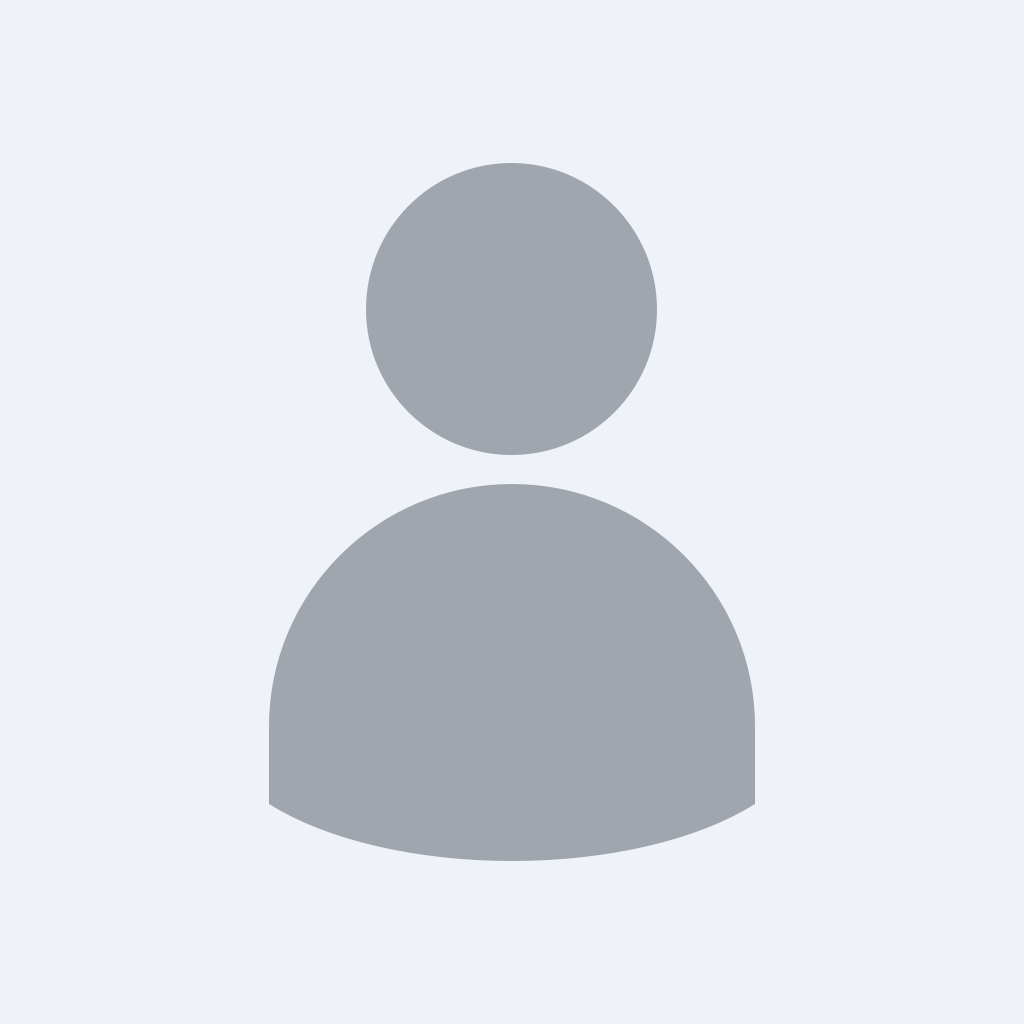