Suicide is a serious and ongoing public health issue. However, the primary method for assessing acute suicide risk relies on patients’ self-report and clinicians’ judgments, both of which have been shown to be rather inaccurate.
In this study, Jeffrey Glenn and Alicia Nobles applied text mining techniques to social media and personal communications to identify features indicative of heightened suicide risk. Their research is novel because it applied text analytic approaches to communications temporally, allowing not only for prediction of suicide risk, but also for insight into how these predictive attributes change as an individual draws closer to their suicide attempt.
Such knowledge may lead to passive monitoring tools that can be utilized by patients and clinicians to more objectively assess level of risk and ultimately reduce rates of suicide.
Jeffrey Glenn is a fourth-year PhD student in clinical psychology. He is interested in understanding how cognitive and emotional processes contribute to psychopathology, with a specific interest in the roles of future thinking, decision making and implicit cognition in suicide.
Alicia Nobles is a second-year PhD student in the Department of Systems and Information Engineering. She is interested in using computational health informatics, specifically machine learning and data mining, to create novel interventions and advance health policies.
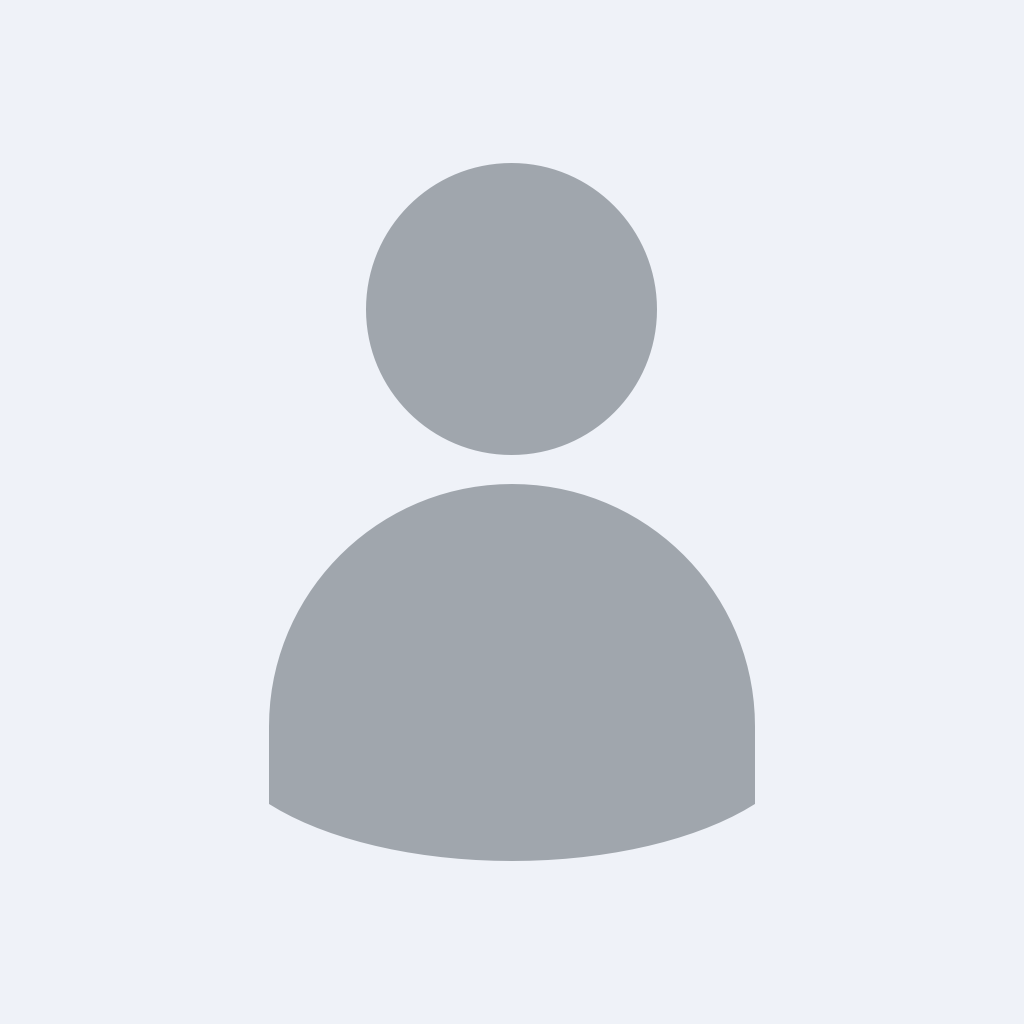
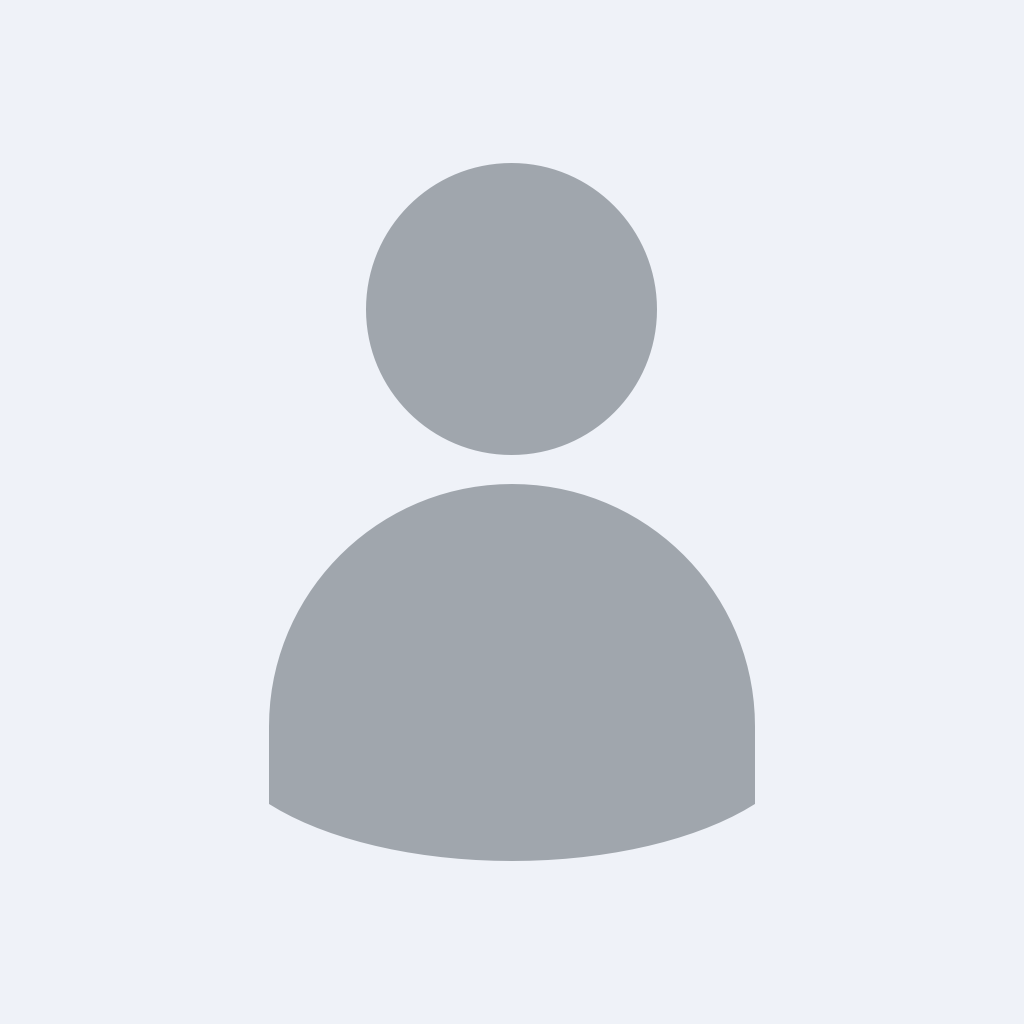