Dean’s Blog: Parallel Universes
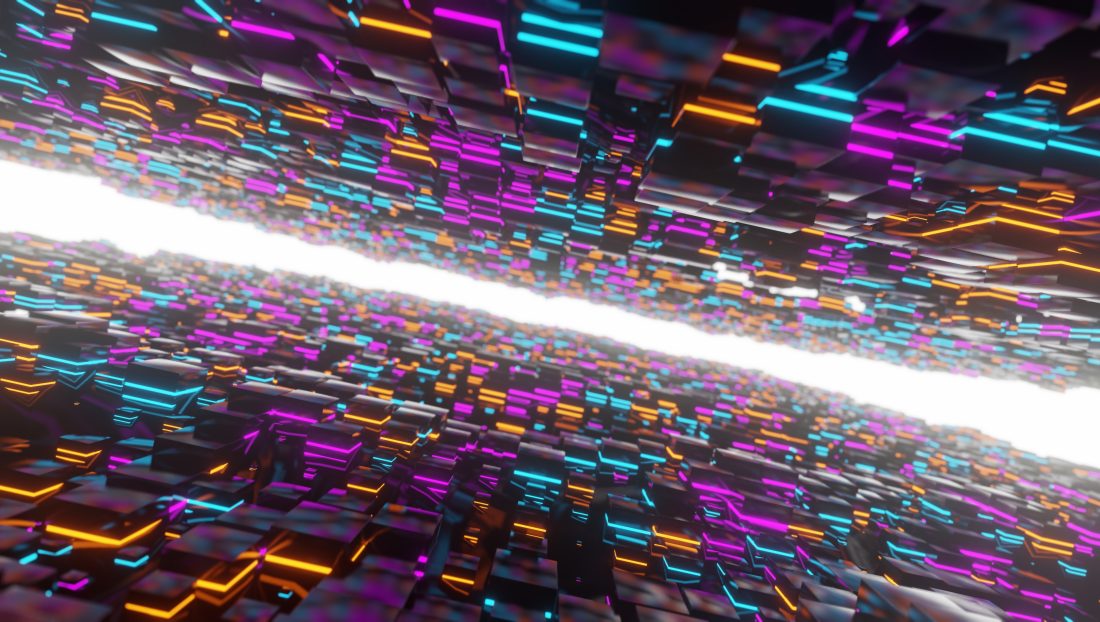
There are at least two universes associated with data science and they seem to operate in parallel. This makes no sense to me. We are not talking about antimatter and matter, where contact would lead to annihilation. We are talking about two universes with a commonly shared matter (aka data) and energy (aka scientists). Let me explain.
There is the universe of data oversight exemplified by CODATA and RDA. According to ChatGPT in response to “What is the CODATA/RDA mission statement?”:
The Committee on Data (CODATA) {formed in 1966} is an interdisciplinary scientific committee of the International Council for Science (ICSU), which was later incorporated into the International Science Council (ISC). CODATA’s mission is to strengthen international science by improving the accessibility, reliability, and sustainability of scientific data {….} Overall, CODATA’s goal is to advance scientific research by enabling effective data sharing, management, and reuse, ultimately contributing to global efforts to address complex societal challenges such as climate change, public health, and sustainable development.
The Research Data Alliance (RDA) is a global organization focused on developing infrastructure and best practices for data sharing and management across different disciplines, technologies, and countries. Its mission is to accelerate research data sharing and exchange by building the social and technical bridges that enable open data sharing.
According to me – In short a universe of international organizations with a mandate of international cooperation around interdisciplinary data sharing and use. We will save the debate for why there is more than one for another day.
The other universe, that has emerged in the last 10 years, is the exploding field of data science of which our School of Data Science at the University of Virginia is just one bright light. That field is driven as much by the private sector as academia – think DeepMind or OpenAI. And when I say academia I do not just mean the traditional digital data-rich disciplines, astronomy, physics, biology, medicine, etc. I mean all disciplines from A-architecture to R-religious studies to Z-zoology and everything in between. Data science – the science of data has become a driver for many of our industries and it is where an ever increasing number of students see their futures. Our definition of data science, which serves the purpose here, is embodied in a 4+1 model. 4 fundamental areas of data science applied to disciplines (the +1). The 4 being systems, design (human-computer interaction), analysis and value (the ethics, policy and law associated with data).
But here’s the thing, in my opinion, there is little connection between these two universes. Thus far they have had little influence on each other. If I asked our undergraduate and graduate students, of which there are many, most if not all, would never have heard of CODATA or RDA. Even sadder, while some of our faculty are aware of these organizations, none are engaged with them to my knowledge. If this is true more broadly, as I believe it is, then this is a huge missed opportunity for both universes.
Before we delve into what we might do about it, let’s understand more why it might be so. Academic data science as a field is relatively new, while CODATA and RDA are older, so perhaps it’s just a matter of timing. CODATA and RDA focus on data governance and establishing good data practices whereas, at the masters level, which is where the majority of data science degree programs exist, the emphasis is on getting the most from messy data in the shortest period of time and then joining the workforce. With Ph.D. programs and students emerging who have interests in policy, ethics, and governance, opportunities for engagement emerge. Much more could be said as to why the two universes have not intersected to date, but let’s focus on what to do about it. Here are a few thoughts that I pose to CODATA.
Student engagement. Students are where the energy and future lie. There are student bodies in both universes. Let’s bring them together. They will figure out the rest if there is something to be done, particularly if it will further their blossoming careers through career development opportunities etc. It will require governing bodies in both universes to respect the role of students in changing their respective universes.
Leadership engagement. Leaders in both universes need to engage with each other. In the data science universe, a good place to start would be the Academic Data Science Alliance (ADSA). They have a leadership summit which would be a beginning. Increasing engagement is a mandate so the opportunity exists.
Beyond engagement. Engagement is a first step, but what are the drivers for continued collaboration? Both universes must see value in spending time together. Perhaps a series of symposia to kick things off. Examples:
• Implications of the latest developments in data science for data sharing and governance
• Data science and national sovereignty
• Implications of generative language models as data generators
So much needs to be done together. To quote ChatGPT:
Generative language models have the potential to be powerful data generators, as they can generate realistic and diverse synthetic data that can be used to augment or replace real-world data. However, the use of generative language models as data generators also raises several important implications and considerations: {…} privacy, fairness and bias, quality and representation, and ethics.
What are your ideas?
Acknowledgments: Thanks to Emma Candelier (UVA) Sarah Nusser (CODATA), Micaela Parker (ADSA), Mark Parsons (CODATA), Steve van Tuyl (ADSA) for their input.
Disclaimer: I have had a passing affiliation with RDA, having given a talk at the first plenary in Gothenberg in 2013 and attended a couple of later meetings while at the University of California San Diego and the National Institutes of Health. I just joined the US National Committee for CODATA to make just these points.