Data Science vs Data Engineering
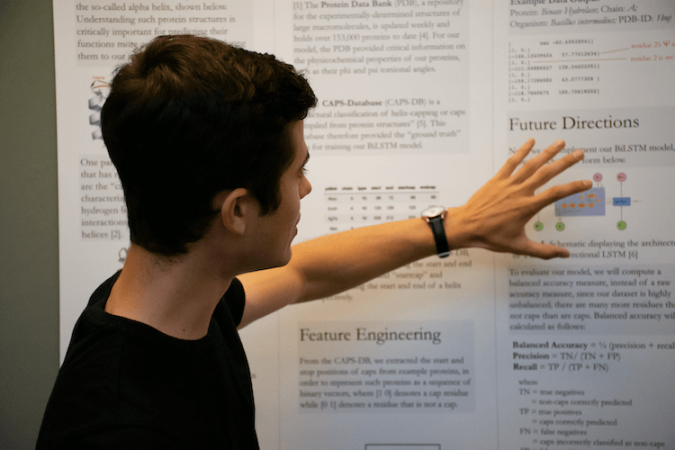
For years, business and technology leaders like McKinsey and Gartner have reported the urgency for companies to implement data science and analytics to improve business strategies. In 2017, Burning Glass Technologies partnered with IBM and the Business Higher Education Forum to quantify the need for data science and analytics professionals. They found an enormous demand, and evidence that artificial intelligence, machine learning, deep learning, and programming skills remain overwhelmingly relevant in business.
However, for those interested in a career path within data and analytics, choosing between the various related roles can be daunting: data scientist, data engineer, data analyst, data architect-data science job trends are ever-evolving.
Below we highlight one potentially confusing comparison, the role of a data scientist versus a data engineer. How do they differ in duties, skills, job outlook, and salary? And which career path is right for you?
Differences between data science and data engineering
If you read through job postings for data scientists and data engineers, you will find overlap in the required knowledge, skills, and education. In fact, a company’s goals for the two positions can sound similar. Sometimes a job posting presents confusion over a) what a data scientist does, or b) exactly what the company needs to solve its data problems. Job posting qualifications (like Python and SQL) can also appear for both data science and data engineering. Because of how these two roles are continually used and molded, the line distinguishing data scientist and data engineer is often blurred.
At the end of the day, though, a data scientist is different from a data engineer. A data scientist cleans and analyzes data, answers questions, and provides metrics to solve business problems. A data engineer, on the other hand, develops, tests, and maintains data pipelines and architectures, which the data scientist uses for analysis. The data engineer does the legwork to help the data scientist provide accurate metrics.
Below is an “at a glance” comparison of a data scientist and a data engineer. This is a general view as you will likely find data scientists and data engineers with varying profiles. Job descriptions often depend on the organization’s size and industry, especially if the available resources are limited. That said, here are the typical characteristics of a data scientist and a data engineer.
Pros and cons of data science versus data engineering
Data scientists are often expected to do the work of both a data scientist and a data engineer. One benefit of studying data science instead of data engineering is that the training for a data scientist is broad and, therefore, versatile. Consider a company that does not distinguish between data engineers and data scientists. In this scenario, a data scientist can perform all of the required tasks, but may not have the requisite depth of knowledge to perform some tasks at an appropriately high level.
Of course, both data science and data engineering advanced degrees have significant benefits. The pros and cons of each hinge on individual backgrounds and career goals. For example, an aspiring data scientist with a bachelor’s degree in psychology might consider a master’s degree in data science to acquire the necessary programming skills and hands-on experience. For an aspiring data engineer already possessing a bachelor’s degree in computer science, an advanced degree may not be required to begin their career because they are already able to perform entry-level data engineering duties.
Below is an outline of common educational backgrounds for data professionals, as well as pros and cons of pursuing advanced education in data science or data engineering.
How to choose between data science and data engineering
Data scientists and data engineers work in tandem. Each skill set is equally important and has a future in tech. However, it was recently reported that only 1 in 10 data science projects make it to production. Why? Because data projects are enormously complex and time-consuming, they require the full contribution of both data scientists and data engineers. Projects fall through the cracks for a number of reasons, but often the data team cannot get past the production pipeline phase due to cumbersome data sets. Without data engineers to build strong and reliable architecture, data scientists cannot efficiently analyze data. Projects become delayed and sometimes dropped altogether when teams run out of time and money.
Concentrating on the day-to-day work of each role, as opposed to job titles, lets you focus on learning the specific skills needed for the role you choose. Your career choice is clearer when you know which part of the data machine interests you most. Not sure? Ask yourself these questions:
- What skills make a good data scientist or data engineer?
- Which skills are better suited to me?
- Can I hone these skills and be successful in one of these areas?
- Will I thrive and be motivated to perform the tasks in this area?
- What do I want to contribute?
Here is a breakout of typical responsibilities for data scientists and data engineers:
Career outlook for data science versus data engineering
While there are important distinctions between data science and data engineering, the top priority is to determine how you want to spend your time every day. Once you have done that, there are other considerations, including job outlook, demand, and salary.
The career outlook for both data scientists and data engineers is promising. Data scientists have been in demand for some time, but recruiters are slowing their search for data unicorns. While it is nice to have a single data professional with ideal communication skills, business acumen, and creativity on top of SQL (Structured Query Language), a more realistic division of labor is more efficient. Companies now focus recruitment efforts on building data teams; these are composed of professionals with different, complementary skill sets and allow more room for specialized data roles, such as data engineers. As enterprises desire to move data to the Cloud, they will need improved data flow and nuanced data storage. The focus on recruiting data engineers will only increase.
According to Google Trends, data jobs have grown over the past decade and are only projected to increase in the future. The graph below shows the growing interest in data jobs since 2010.
There are data science and data engineering job opportunities across a variety of industries. Whether in government or healthcare, companies understand the need for data science in any discipline.
The chart below provides an overview of the job potential in data science and data engineering, including annual income potential, experience required, top hiring cities, and the projected job growth.
Data science or data engineering? Be prepared for both with a Master’s in Data Science from the University of Virginia
Whether you want to pursue a career in data science or data engineering, pursue an online Master of Science in Data Science (MSDS) from the University of Virginia’s School of Data Science. UVA’s MSDS program offers foundational and advanced courses in a variety of in-demand disciplines:
- Programming and Systems
- Linear Models
- Data Mining
- Text Analytics
- Data Visualization
- Ethics of Big Data
- Machine Learning
The program also ensures graduate candidates apply theory to real-world experiences by offering practice and applied courses, as well as a capstone project. To complete the capstone project, students work with peers, advisors, and clients through hands-on learning.
Led by expert faculty, the online MSDS program is committed to providing career services which help graduates land competitive, exciting jobs. The graduating class of 2017 received employment offers from top employers such as:
- Amazon
- Capital One
- Deloitte
- IBM
- Morgan, Lewis & Bockius
- National Institutes of Health
- University of Virginia Health System
- Procter & Gamble Company
- KPMG Global
UVA’s online MSDS program meets you where you are. This means graduate students have the flexibility needed to meet the demands of life and a career via engaging online curriculum.
Now more than ever, data is shaping the future. Are you ready to contribute your curiosity, imagination, and energy to the world of data science?