Credit card fraud is an expensive problem for many financial institutions, costing billions of dollars to companies annually. Many adversaries still evade fraud detection systems because these systems often do not include information about the adversary’s knowledge of the fraud detection mechanism.
This project, conducted by MSDS students Frankie Zeager, Aksheetha Sridhar and Nathan Fogal, aims to include information about the “fraudster’s” motivations and knowledge base into an adaptive fraud detection system. They used a game theoretical adversarial learning approach in order to model the fraudster’s best strategy and pre-emptively adapt the fraud detection system to better classify these future fraudulent transactions.
Using a logistic regression classifier as the fraud detection mechanism, they initially identified the best strategy for the adversary based on the number of fraudulent transactions that went undetected and assumed that the adversary used this strategy for future transactions in order to improve our classifier.
Prior research has used game theoretic models for adversarial learning in the domains of credit card fraud and email spam, but this project adds to the literature by extending these frameworks to a practical, real-world data set.
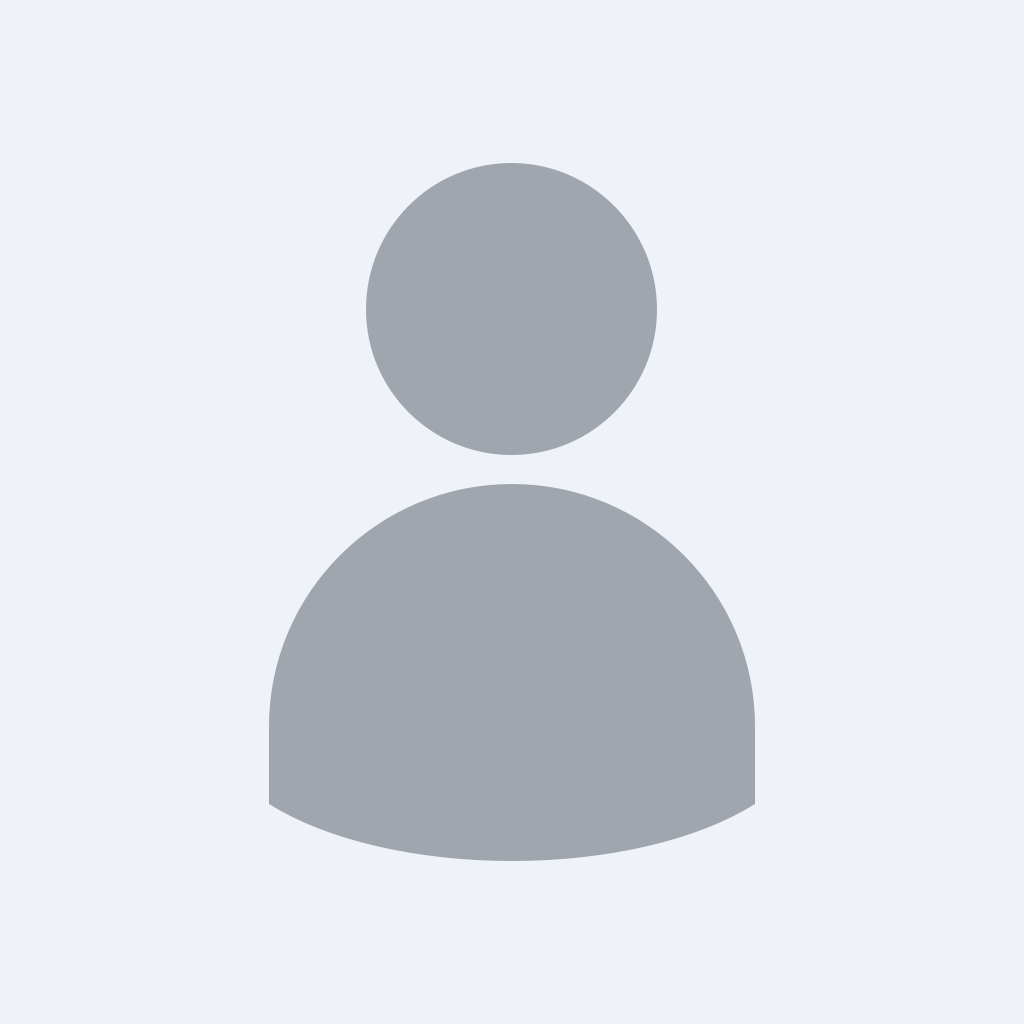
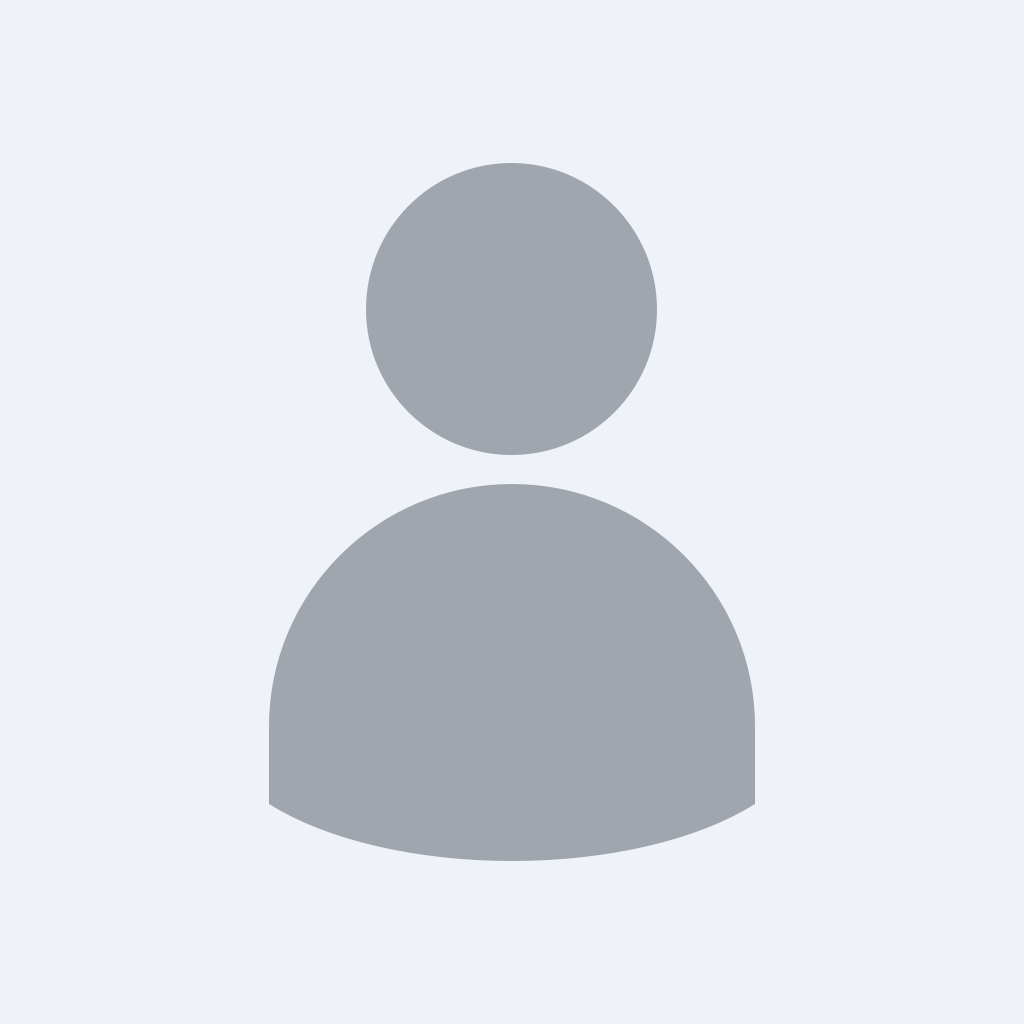
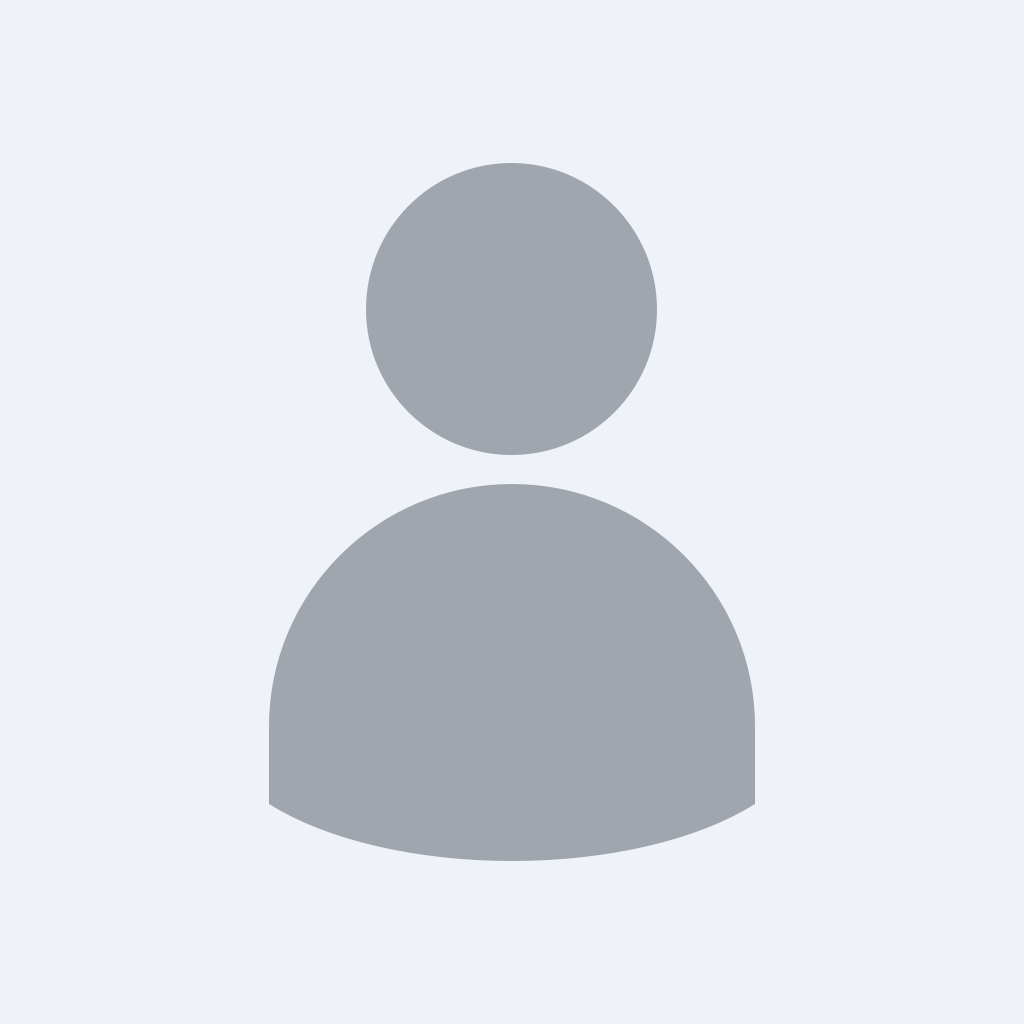