The U.S. military is both a producer and consumer of vast quantities of data. The data come from a variety of sensors, including radars, hyperspectral imagery and receivers across the electromagnetic spectrum. At an abstract level, data can be viewed as the outputs from point or vector processes in space and time.
With extensive research programs funded by the U.S. Department of Defense, University of Virginia researchers Maïté Brandt-Pearce, Donald Brown, Stephanie Guerlain and Barry Horowitz could produce significant results with signal processing, data fusion, visualization, human factors and cyber-security.
The fusion processes developed in the Predictive Technology Laboratory took these data from multiple sources and combined them using hierarchical models. The models have components that represent the different sources of data and enable the estimation of dependencies between the components. For example, multiple layers of remotely sensed data about an area (such as slope, vegetation, surface materials, roads and man-made obstacles) can be combined, resulting in an integrated model to predict land use.
Dependency structures make it possible to predict evolving or changing processes. The methods developed by this team can exploit massive amounts of contextual data and can also use other aspects of the dynamic environment, such as movements by objects or the changing characteristics of objects in an area.
This overall hierarchical modeling framework has broad applicability. It is being implemented in high-performance environments for use on large, multi-type data integration problems.
Going forward, the key research to support this project will focus on
- the translation of this framework to XSEDE and other CI elements, and
- the use and extension to more data types found in a broader range of interdisciplinary problems.
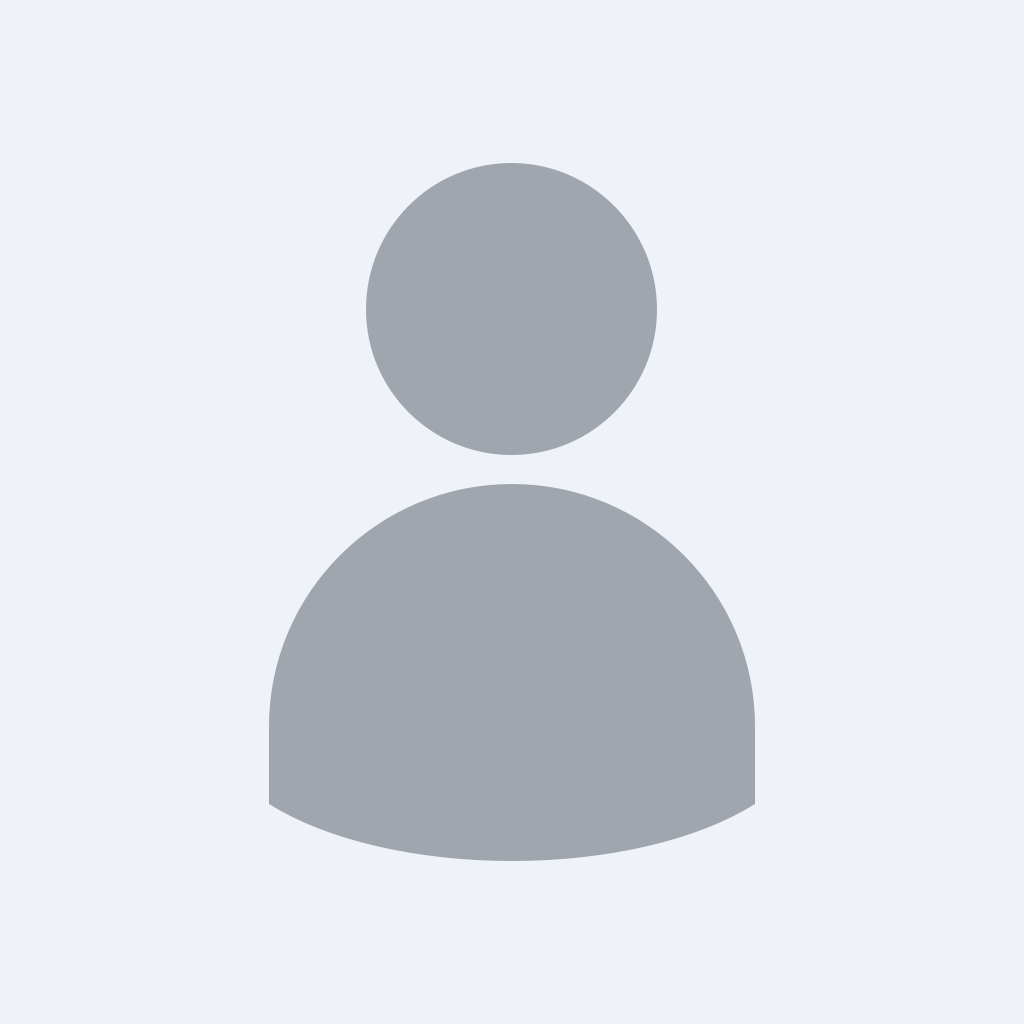
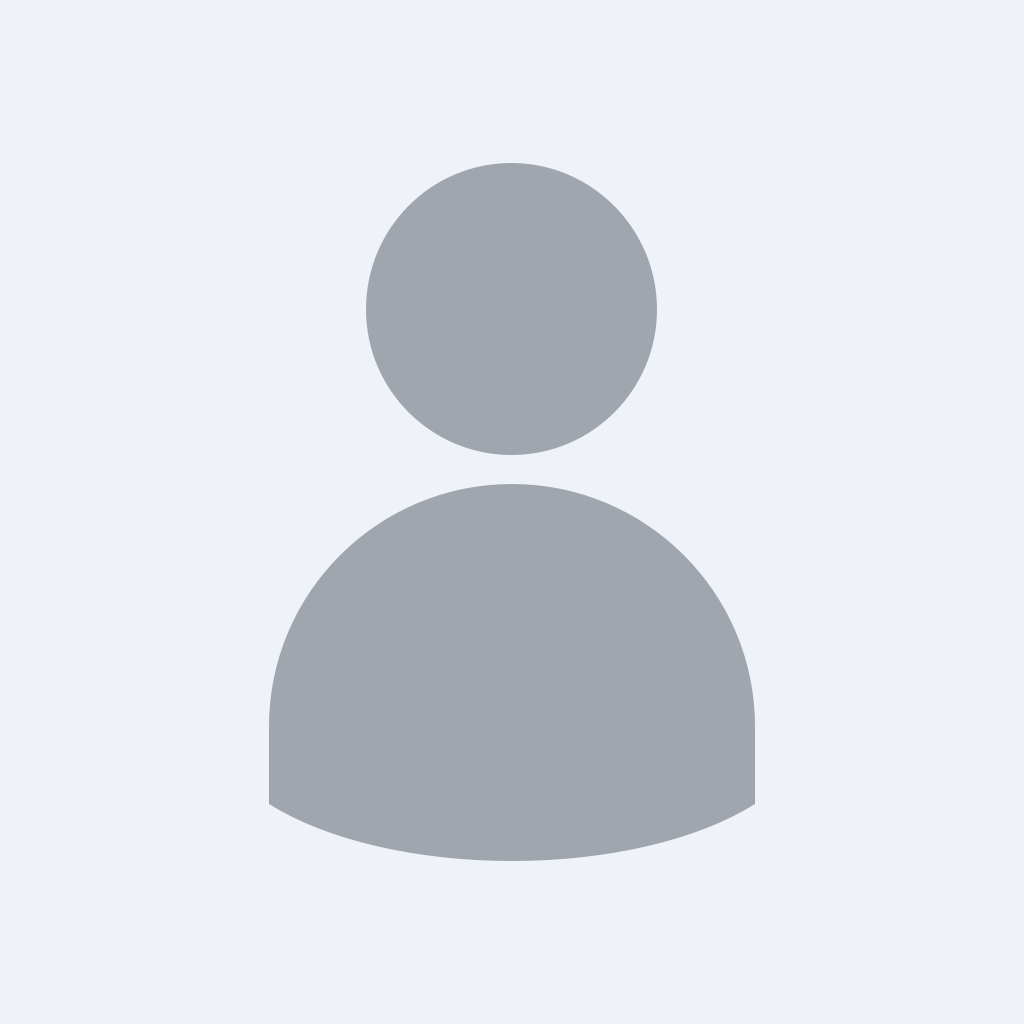
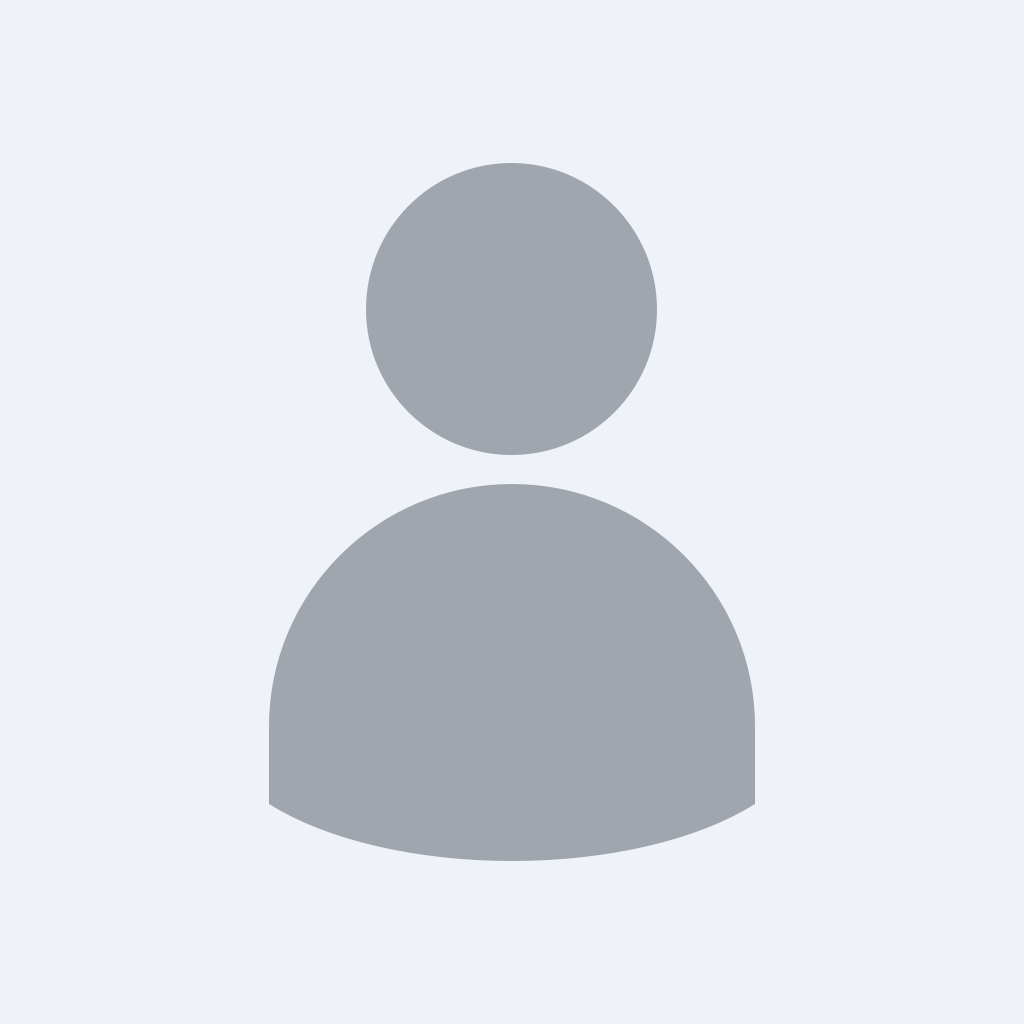
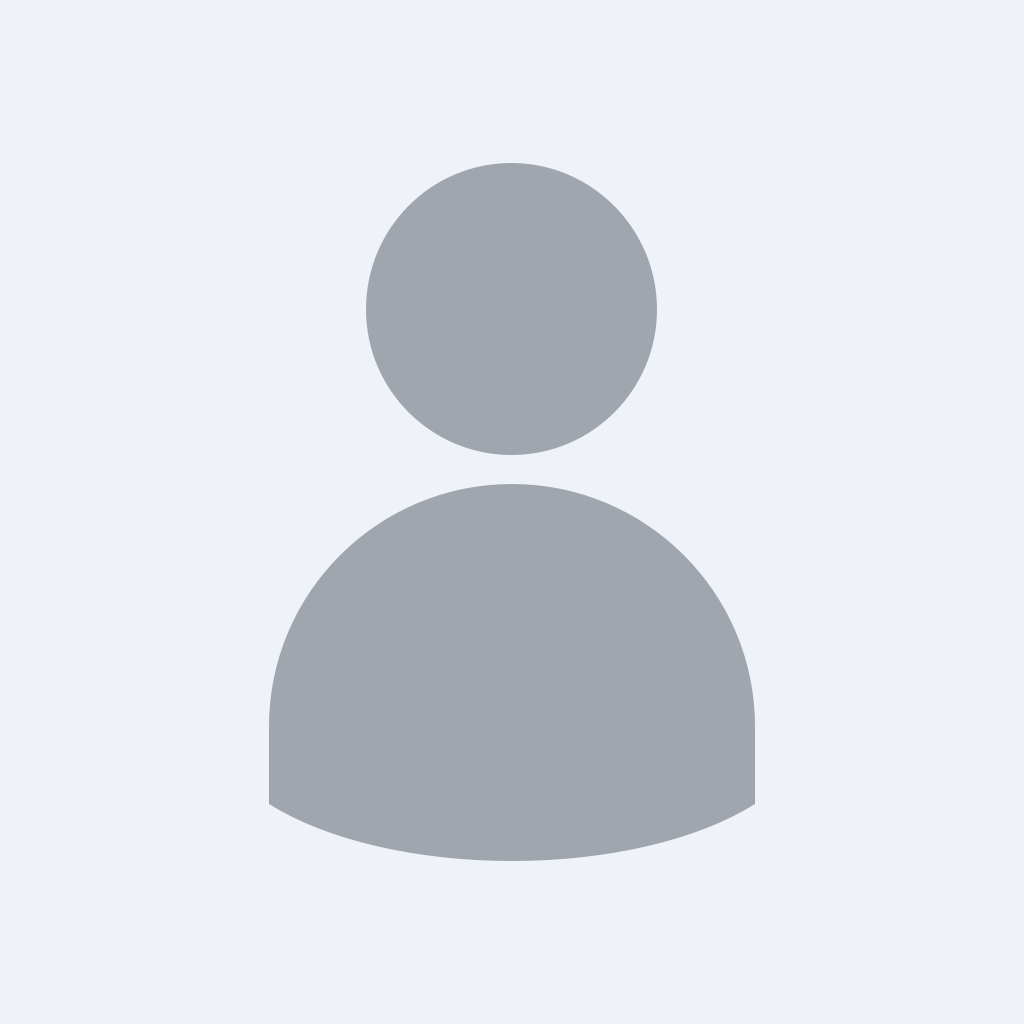