Networks are vulnerable to costly attacks. Thus, the ability to detect these intrusions early on and minimize their impact is imperative to the financial security and reputation of an institution.
There are two mainstream systems of intrusion detection (IDS), signature-based and anomaly-based IDS. Signature-based IDS identify intrusions by referencing a database of known identity, or signature, for each of the previous intrusion events. Anomaly-based IDS attempt to identify intrusions by referencing a baseline or learned patterns of normal behavior. Under this approach, deviations from the baseline are considered intrusions.
The project, conducted by MSDS students Julina Zhang, Kerry Jones and Tianye Song, investigated unsupervised techniques for anomaly-based network intrusion detection. For this research, they used real-time traffic data from University of Virginia network.
The team evaluated the performance of Local Outlier Factor (LOF) and Isolation Forest (iForest) by probing the similarities and differences between the result of each approach. They found that iForest performed well in identifying anomalies compared with LOF.
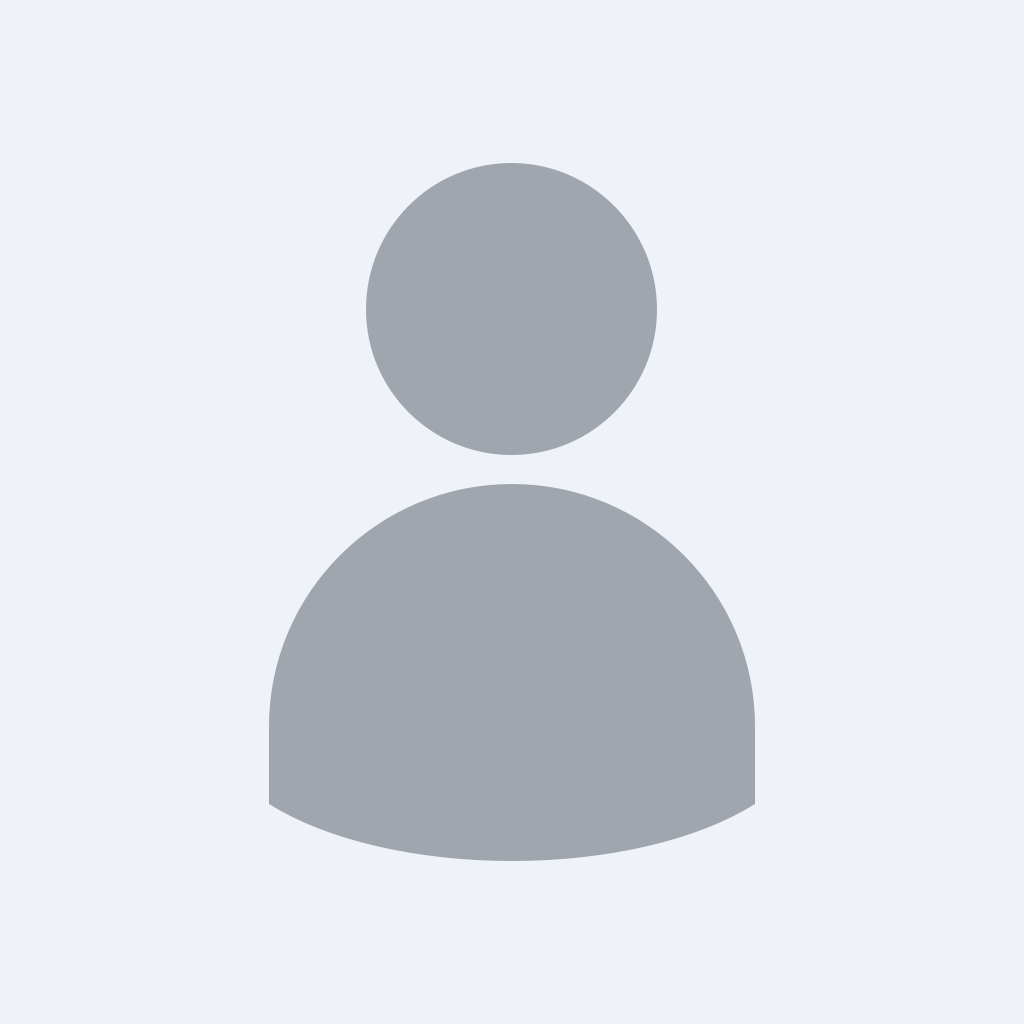
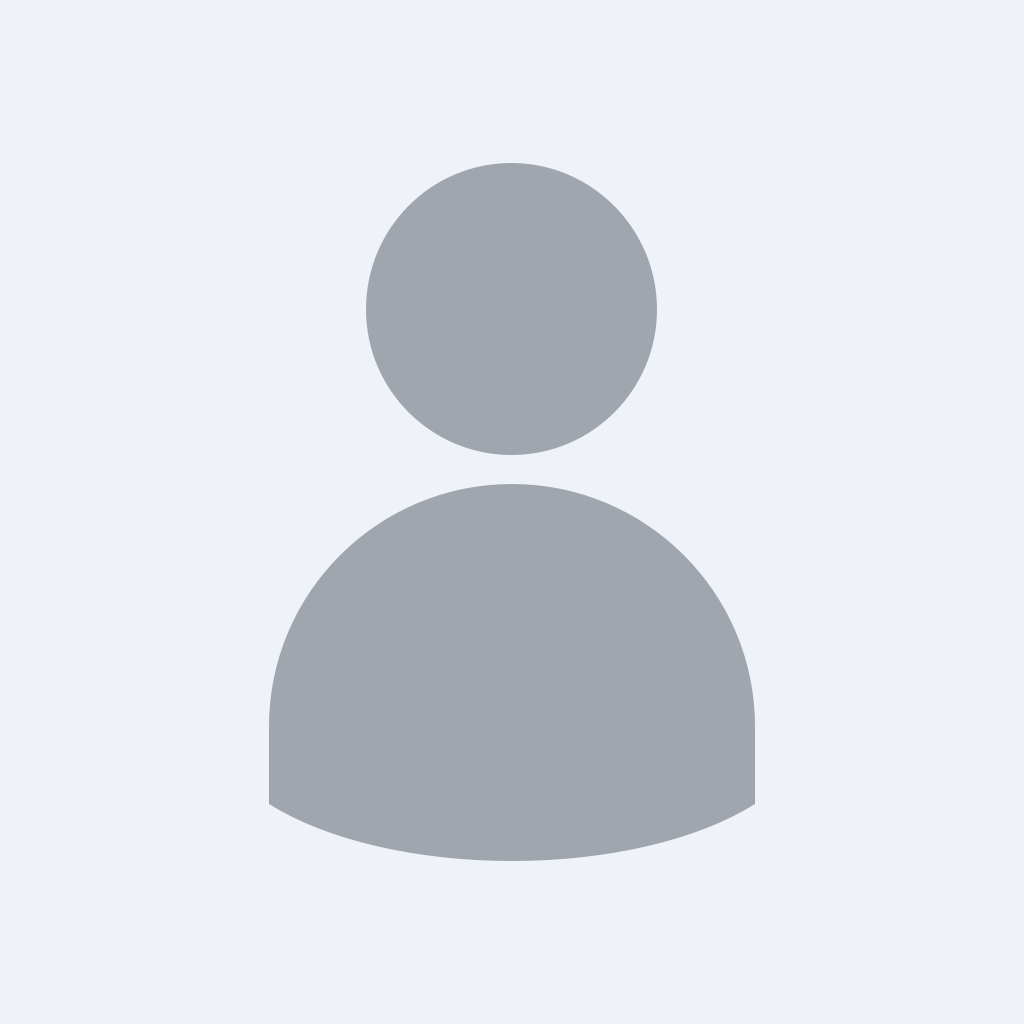
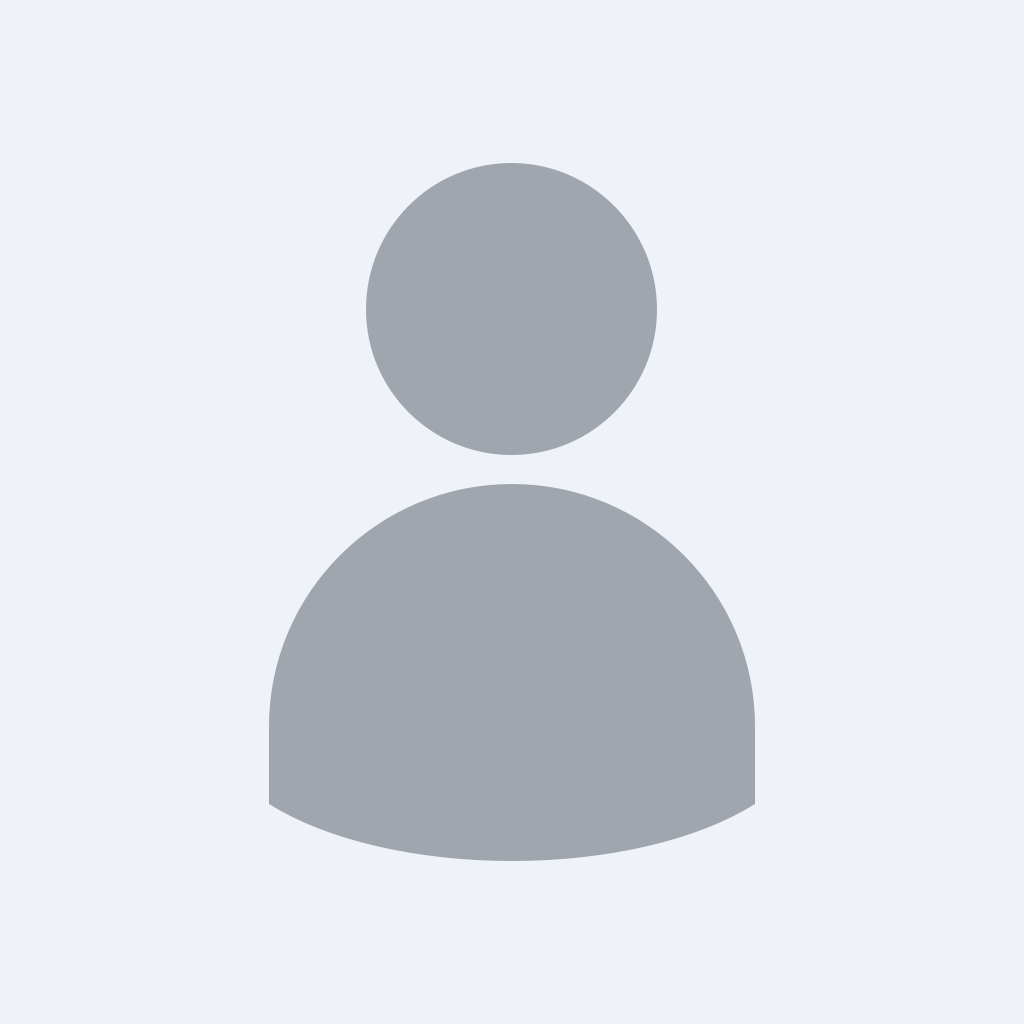
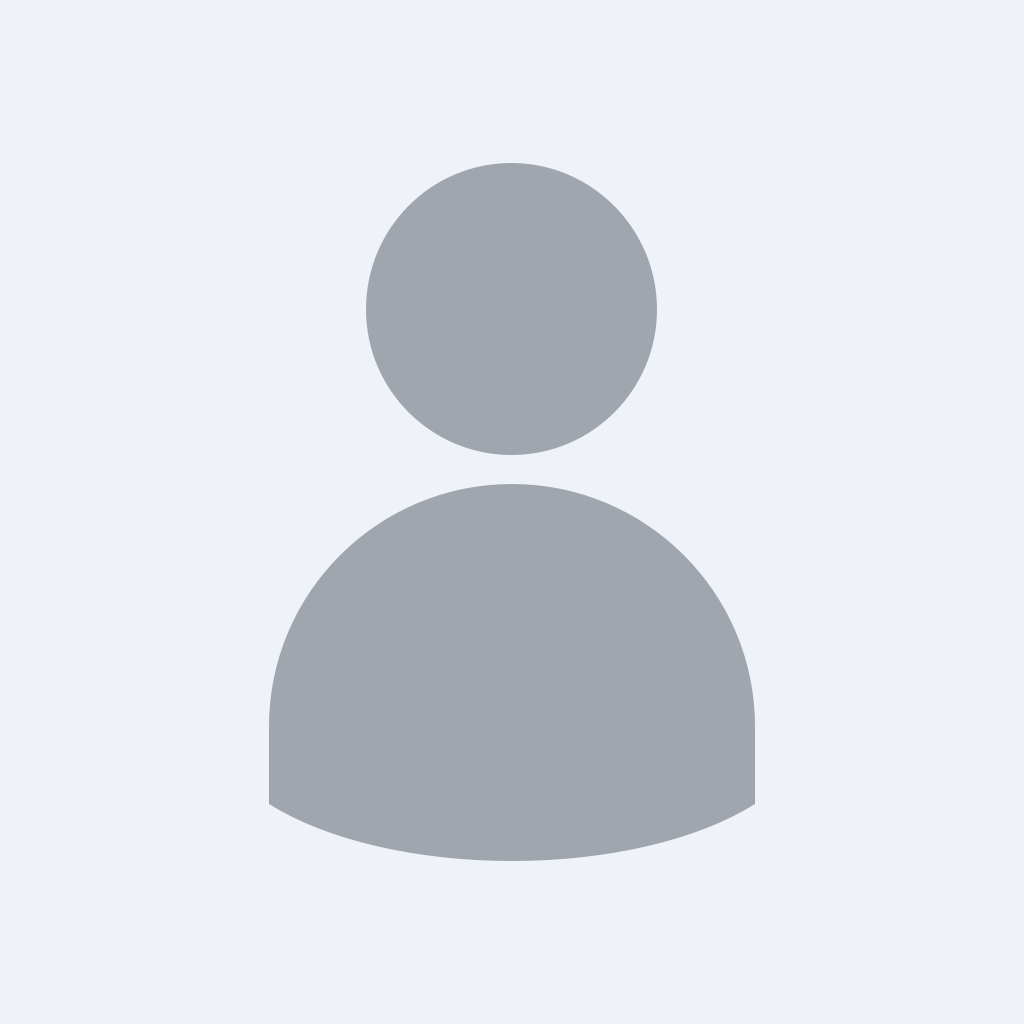