Increased interaction among people with distinct perspectives can lead to increased conflict.
The multiplicity of available data can alleviate this burden by offering an informed avenue for comprehension. Parsing these data sources for relevant information is a time intensive and academically challenging task.
The research, conducted by MSDS students Colin Cassady, Fandi Lin and Thomas Molinari, looks to explore the practicality of parsing these sources of data by attempting to surface latent cultural structures from organized texts. By analyzing sources that heavily rely on nationalistic subtexts, our research looks to find non-obvious sentiment on latent cultural events and topics that would typically require dedicated research to discover and dissect.
The two data sources this work considers are nationalist journalistic efforts: An Phoblacht and TamilNet. The team explored different paradigms for latent variable discovery in textual data, including topic modeling and word embedding, and synthesized them with sentiment analysis to produce a composite model for discovering and classifying unseen cultural context within the text.
Cassady, Lin and Molinari paid additional consideration to evaluating how these composite models shift over time and what implications this holds for identifying patterns of reaction for these populations.
Their exploratory results show predicted latent cultural structures within the text, while their time series analysis indicates several outliers in the usage of language that indicate potential latent cultural perspectives.
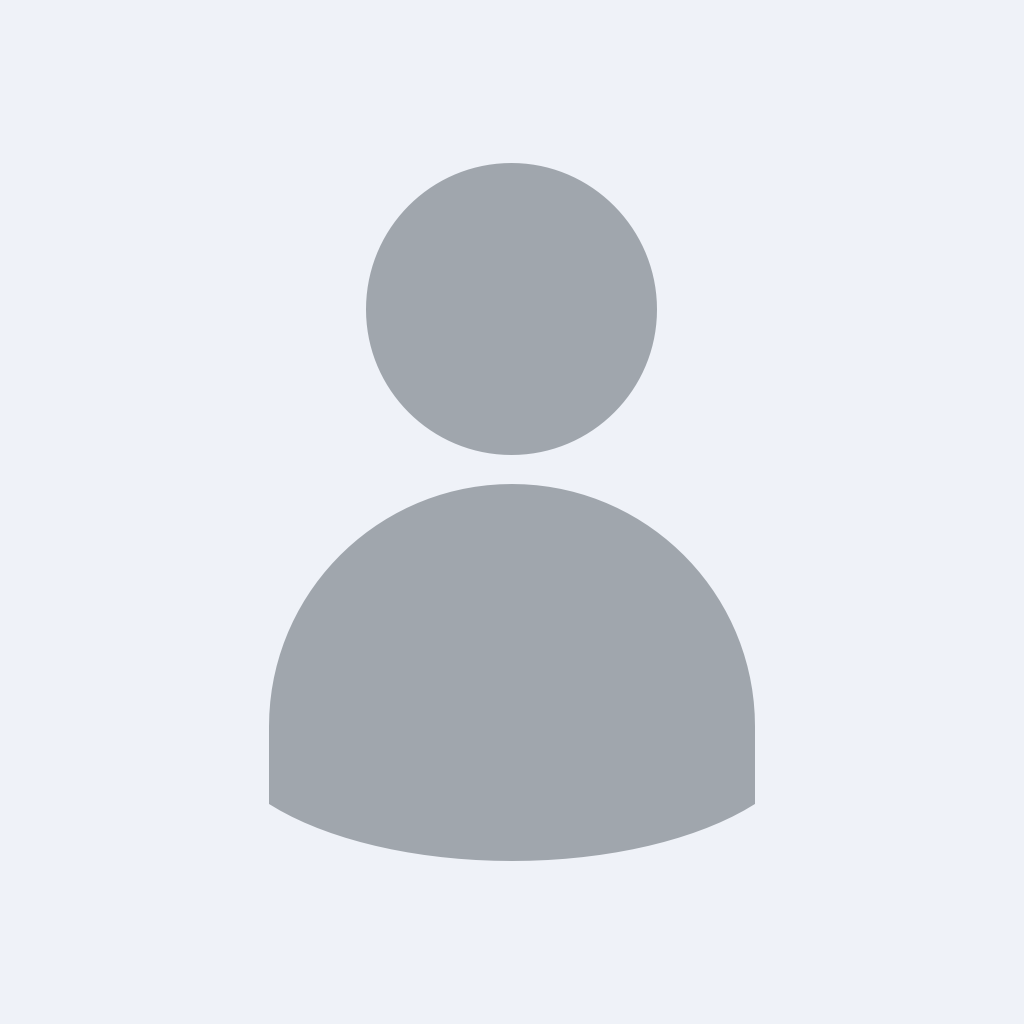
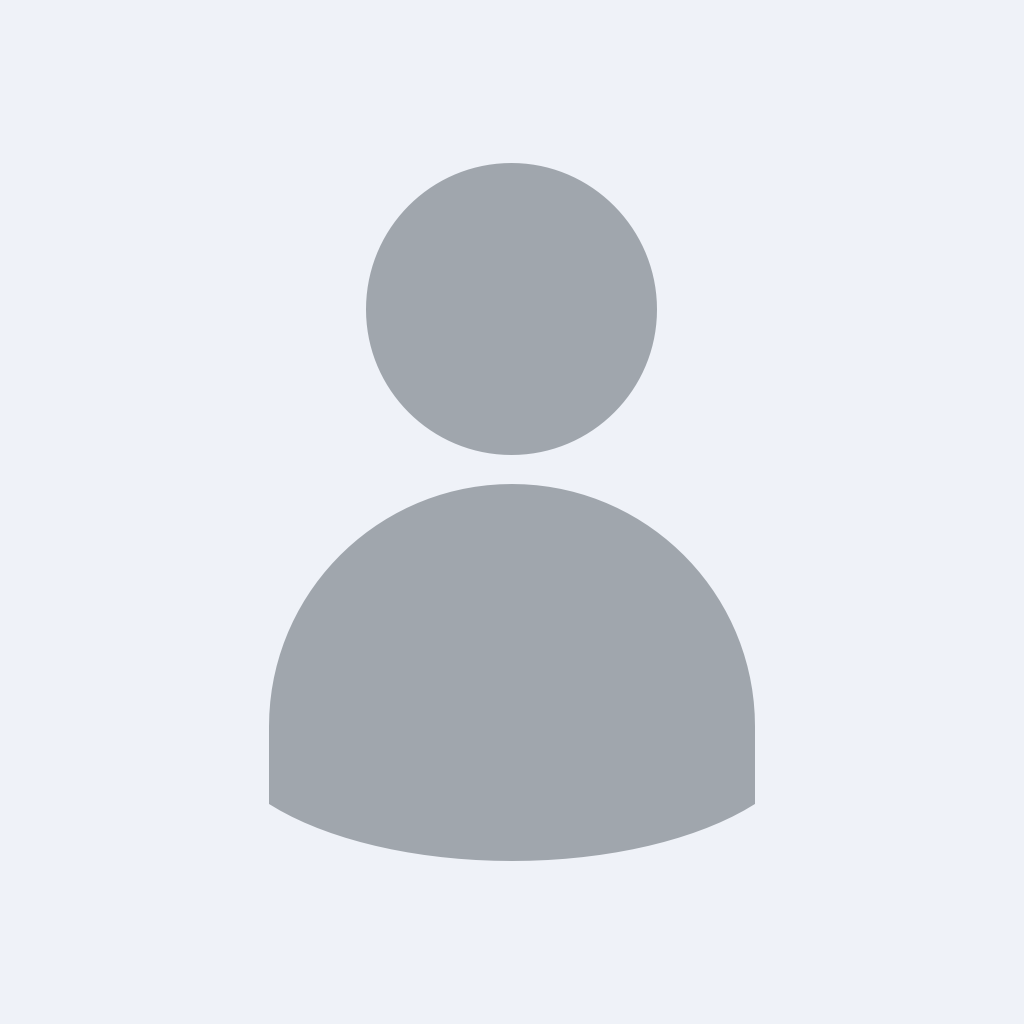
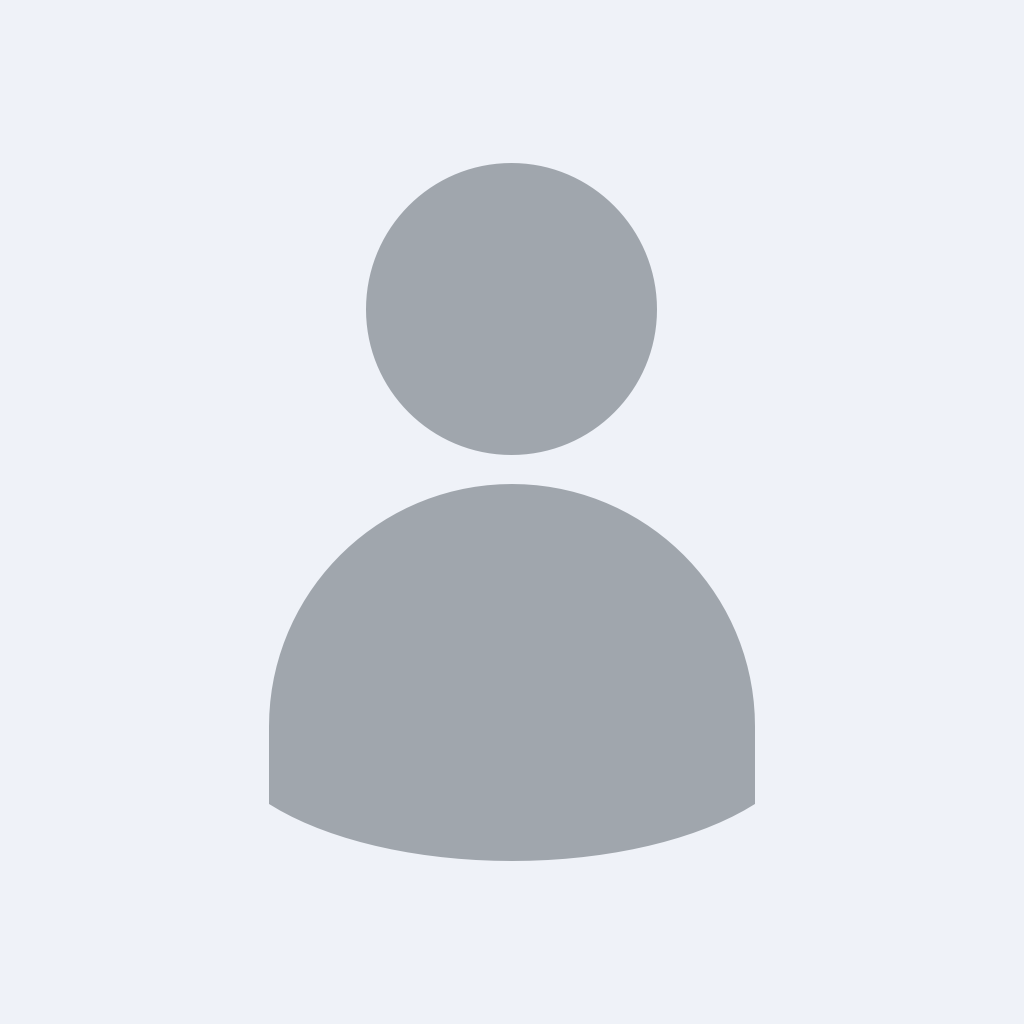