Will Steph Curry’s Next Shot Be a Swish? These Data Science Students May Know
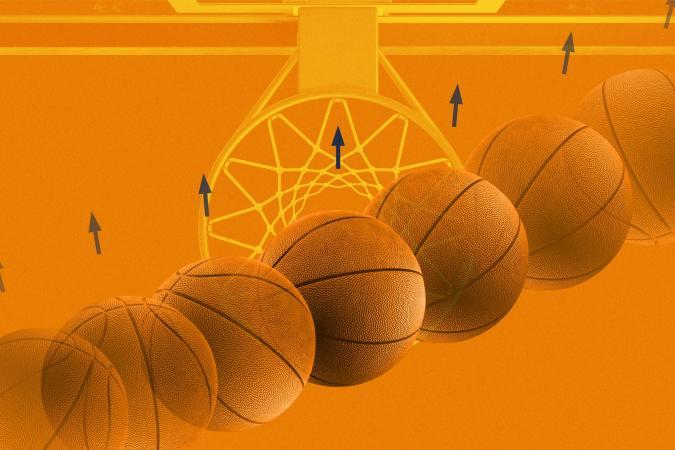
Published Feb. 17, 2022 by UVA Today
NBA superstar Steph Curry is one of the best shooters the world has ever seen. But as great as he is, he still misses roughly 53% of his attempts. (The average shooter in the NBA misses about 55% of his shots.)
With so much of a player’s success based on taking optimal shots – such as ones from the best locations on the court, as well as other factors – four UVA students from the University of Virginia’s School of Data Science set out to build a model that could predict whether a field goal attempt will be a make or a miss.
Using a dataset from the 2016-17 season, which tracked more than 210,000 shots, the team of Kristy Bell, Abhi Dommalapati, Jack Peele and Spencer Bozsik created a model that won the School of Data Science Sports Analytics Club’s first-ever Hackathon last semester.
“I was proud of my team and how we worked together to effectively use the data science pipeline to answer our question of interest,” Bell said. “More than anything, winning the competition gave our group confidence that we were able to apply what we were learning in our program to a real sports dataset.”
UVA Today caught up with Bell, a Pennsylvania native who graduated last spring from UVA with an undergraduate degree in statistics and economics – and who is now pursuing her master’s degree here in data science – to learn more about the team’s model and methodology.
Q. Can you tell UVA Today readers a little more about the team’s objective?
A. We were provided with a dataset that listed several attributes for every shot in the 2016-17 NBA season, including player, home team, away team, shot type, shot location, time left in the game and the player’s last shot outcome. The data required a bit of data wrangling prior to model building (e.g. changing shot location to distance from the net, simplifying shot type).
Using a subset of the provided features we sought to construct a model to predict whether a shot was a make or a miss. … Our ultimate goal was to have a model that was able to accurately predict whether or not a shot was made, on a fresh dataset containing the same variables.
Q. How did you, Abhi, Jack and Spencer work on this as a team? Did you divvy up specific tasks?
A. For the most part, we decided to code together. Whoever wasn’t sharing their screen was on their computer coming up with more ideas and/or parsing through class notes to help the teammate actively coding. Although we found group coding to be efficient, the allocated meeting time for the hackathon was insufficient for adequate data cleaning and model building. As a result, we met once together outside the allotted time to test our model and tune our parameters.
Q. Were there any things that surprised you during the course of the project?
A. We were surprised to find that the NBA shot log data was not as clean and intuitive as we initially believed. More time during the hackathon was spent understanding where the data comes from and creating new variables from old ones than we had anticipated.
For example, we had to rely on metadata to understand where on the court the “x” and “y” location coordinates were referring to – and used them to create a distance to the basket variable. In many ways, cleaning the data was like a treasure hunt; having four pairs of eyes to catch mistakes was very beneficial! Discovering flaws and wrangling raw data is a very common data science problem that emphasizes why the hackathon was a great educational experience.
Q. What were the team’s conclusions?
A. A main takeaway of the project was that modeling shot outcomes is not as straightforward as we expected – at least with this limited dataset. There are many variables that were not incorporated into our model that likely have a significant impact on making or missing a shot. As a result, we were unable to achieve the high accuracy we were expecting and hoping for – no groups could. Perhaps using a deep-learning technique that utilizes player tracking data could lead to superior performance.
Q. What becomes of this model now? Will you try and improve on it or maybe see if any professional teams would like to do anything with it?
A. Throughout our time in the program – and since the hackathon – we are always being introduced to new, more complex machine learning models and data science tools. As a result, I have found myself brainstorming how alternative models or strategies could be used to improve the performance of our shot prediction model.
In fact, we had a couple members of the club use the hackathon dataset for their final project in the Bayesian machine learning course. With that, they found that a more complex, hierarchical model that accounts for differences between players yielded higher accuracy in shot predictions, which was exciting to see!
Q. Is there anything else you’d like to add?
A. Gaining experience working with messy sports data and producing a model that you are proud to talk about during interviews is extremely valuable when trying to break into the sports analytics industry. Due to the work performed during the Sports Analytics Club meetings, I have been able to have more confidence and experience going into these interviews.
Furthermore, the hackathon elevated my interest in using granular data to construct models that can effectively predict sports outcomes. I am excited to pursue this interest after graduation working as a data scientist at FanDuel Sportsbook. I know that many members of the [Master of Science in Data Science] Sports Analytics Club – including myself – really enjoyed the NBA hackathon and look forward to showcasing our developing skills to a different sports-related dataset during the next hackathon later this spring semester.