Researchers Investigating Climate Change Impact on Shenandoah forests
To get a tree core sample, Jake Malcomb, a University of Virginia doctoral student in environmental science, uses an increment borer – a big drill bit that’s hollow in the middle – and drives it into a tree, extracting a piece of wood roughly the thickness of a pencil and half the length of the tree’s diameter.
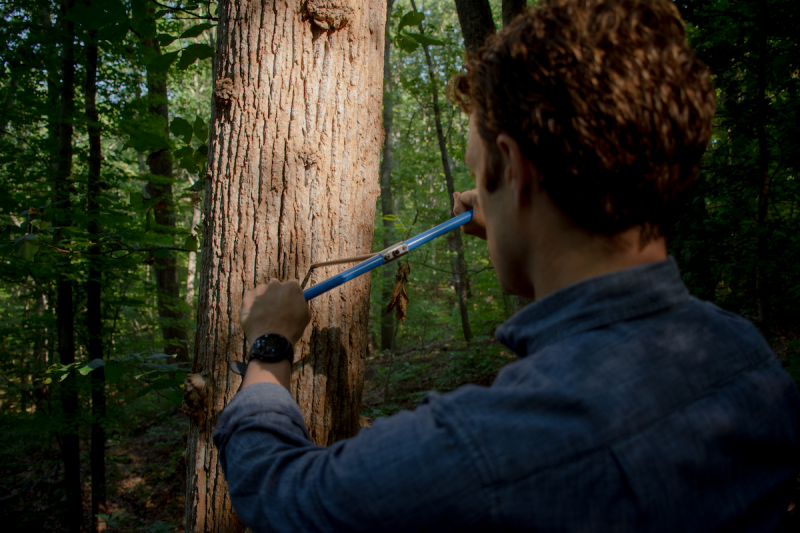
So far, Malcomb has collected cores from 60 trees of three species in Shenandoah National Park, aiming to see how they are responding to climate change. It’s a time-consuming process, though, and he would need to drill a lot of holes to get a meaningful sample size in a park with more than 35 million trees.
Luckily, Malcomb is about to get an assist from the International Space Station.
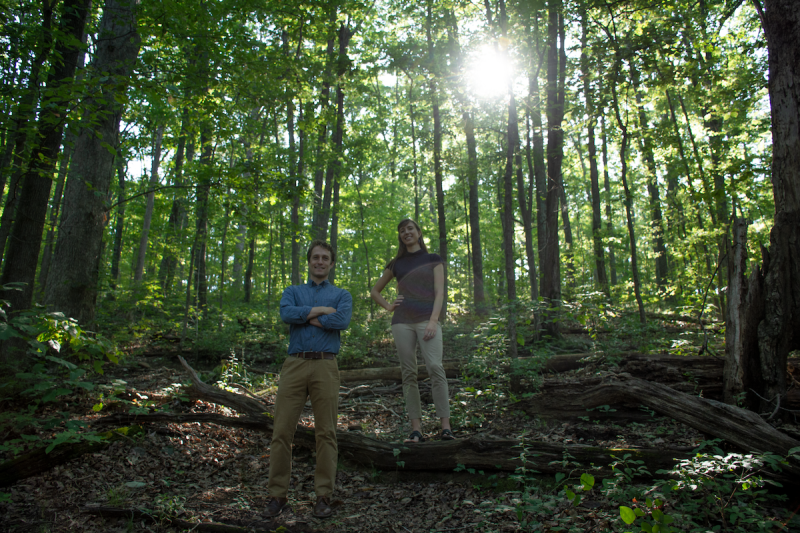
Malcomb and his research partner, Linnea Saby, a doctoral candidate in UVA’s Department of Engineering Systems and Environment, plan to analyze a massive geospatial data set collected over a two-year period from the International Space Station, and then parsed by an “extreme machine learning” tool that aims to mimic the human brain.
The project is part of the Presidential Fellows in Data Science program at the UVA Data Science Institute, which provides funding to Ph.D. candidates partnering on collaborative, multi-disciplinary research projects that address real-world problems using traditional research methods alongside cutting-edge data science tools and techniques.
Malcomb uses an increment borer to test how a tree is responding to climate change. (Photo by Cody Huff, DSI Multimedia Producer)
“The tree core samples provide valuable temporal information about long-term tree growth and physiology,” Malcomb said. “And machine learning will allow us to use geospatial data to understand forest ecosystems on an unprecedented scale.”
Taken together, the data will provide a more complete picture of the effects of climate change on the world’s forests.
Here’s how it works.
Data Collection
In the same way that humans sweat, plants regulate their temperature by releasing water through tiny pores on their leaves, a process called “transpiration.” If they have sufficient water, they can maintain their temperature; but if there is insufficient water, the plant’s temperature rises, placing stress on the plants, the entire forest ecosystem and human society in turn.
It is this temperature rise that the space station aims to track, helping scientists and forest managers understand the varying environmental responses to climate change and how to best manage ecosystems. What tree species are most resilient? What areas are most important to preserve and which forests are going to be more or less adaptable to a changing climate? How are changes in carbon storage and water filtration performed by forests affecting our air quality and water supply?
Two new programs from NASA – the ECOsystem Spaceborne Thermal Radiometer Experiment on Space Station, or ECOSTRESS, and the Global Ecosystem Dynamics Investigation, or GEDI – will provide Malcomb, Saby and other scientists with an extraordinarily large geospatial dataset to answer those questions, collected over a two-year period by equipment attached to the International Space Station.
The ECOSTRESS tool is a multispectral thermal infrared radiometer that has been orbiting the Earth aboard the space station for two years, measuring and documenting surface temperatures to aid scientists in understanding global ecosystem response to climate change. It will acquire the most detailed temperature images of the surface ever taken from space and will measure the temperature at the scale of an individual farmer’s field, helping scientists understand how changing temperatures are affecting plants’ water filtration, storage and management.
GEDI “will provide answers to how deforestation has contributed to atmospheric CO2 concentrations, how much carbon forests will absorb in the future, and how habitat degradation will affect global biodiversity,” according to NASA.
Malcomb and Saby applied to be part of the ECOSTRESS Early Adopters program, which includes more than 234 Early Adopters from more than 21 countries, across academic, government (U.S. and non-U.S.), private and NGO sectors.
“We rely a lot on theoretical models to measure evapotranspiration and biomass,” Saby said. “The data from ECOSTRESS and GEDI give us the opportunity to get a real-life, big-picture understanding of the effects of climate change on entire forest ecosystems.”
The Dataset
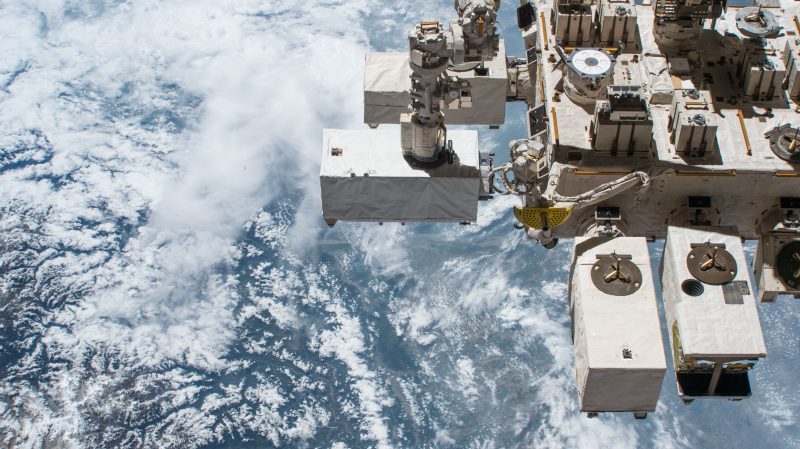
The ECOSTRESS instrument passes over the same point on Earth every three days, so Malcomb and Saby will have 121 potential days of data collected per year. The measured area of Shenandoah National Park is 806 square kilometers, which converts to approximately 164,500 70-meter-by-70-meter observations per day. Over the course of a year, that equals a potential of more than 20 million data points. (In reality, the team can expect to have one-half to two-thirds of that because the instrument can’t collect data on cloudy days.)
“The size of this dataset will allow us to answer questions at spatial scales that are not attainable using traditional field work,” Malcomb said.
“It will give us information about how entire forests are composed and how they’re functioning,” Saby added.
Data Management & Analysis
To analyze the data, Saby and Malcomb will use a data science tool called an extreme learning machine, or ELM, combined with more traditional data science methods. ELMs are a type of artificial neural network – an algorithm based upon the structure of the human brain. Neural networks “learn” from real-world data to recognize patterns or predict outcomes. For this project, Saby and Malcomb selected ELMs because they needed a machine-learning algorithm that could work well with a very large dataset.
“It is a constant battle in working with geospatial data to find computationally efficient ways of handling it, because these are very, very large datasets,” Saby said. “Extreme learning machines are a fairly simple neural network that has shown a lot of promise in handling this type of data.”
The team will use data collected previously on the ground in the forest to test their machine learning model’s effectiveness. They hope that in addition to investigating the effects of climate change in Shenandoah National Park, they can create a model that can be used to make predictions in areas where there is no preexisting data or where data collection and management have been impossible or underfunded.
“I am excited about this work because of the great environmental problems we are facing,” Saby said. “And the enormous opportunity we have to apply data science and engineering to solve these problems.”