Professors Baek and Gates Win $900K Award from NNSA to Advance Physics-Informed Machine Learning
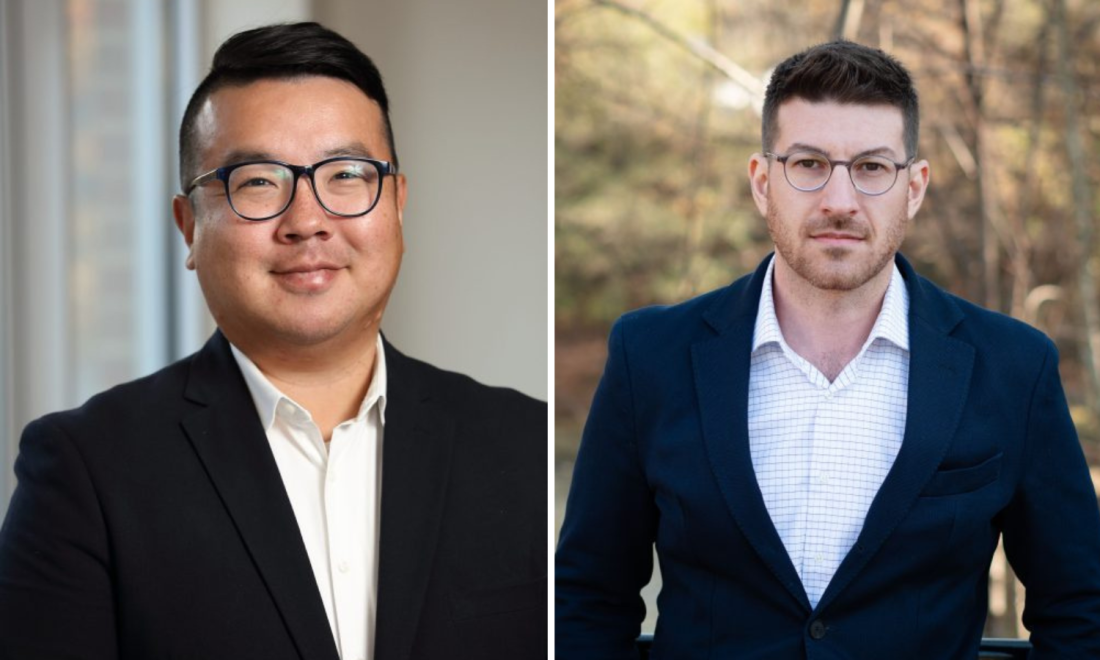
The National Nuclear Security Administration (NNSA) within the U.S. Department of Energy has announced that it is awarding a $900,000 research and workforce development grant under the Stewardship Science Academic Alliances (SSAA) Program to a University of Virginia School of Data Science team led by Stephen Baek, Quantitative Foundation Associate Professor of Data Science, and Alex Gates, Assistant Professor of Data Science.
In the project entitled “Advancing Physics-Informed Machine Learning for the Discovery of Structure-Property-Performance Linkages in Multiphase Heterogeneous Energetic Compounds,” Professors Baek and Gates will collaborate with a University of Iowa team, led by Professor H.S. Udaykumar, to develop machine learning theories and methods for modeling extreme, high-energy-density physics phenomena, which can contribute to the safe storage, monitoring, and handling of energetic materials.
Baek, the lead investigator of the project and an expert in physics-aware deep learning, said that energetic materials are “highly interesting, not only from the physics and chemistry perspective, but also from the data science perspective.”
“The high-energy-density physics of energetic materials generates extreme data features and patterns that emerge and vanish rapidly, which are the characteristics that conventional machine learning models, from a purely statistical perspective, are designed to disregard in order to avoid overfitting,” Baek explained. However, he added that “these extreme and fast transient patterns are the key phenomena of interest, from a physics standpoint, and thus must be accurately modeled.”
According to Baek, such a difference in world views between the physics modeling and the data science communities is where lots of research opportunities emerge.
Augmenting the physics-aware deep learning expertise of Baek will be Gates, who is a co-investigator of the project and a rising star in network sciences. “Our work builds off the idea that explosive reactions don’t follow a neat, orderly grid — they spread through a web of microstructures, pressure waves, and shock fronts,” Gates said. “A network model lets us flexibly map out these relationships, ensuring we don’t miss the critical pathways that turn a spark into a blast.”
In this three-year project, Baek and Gates will explore mathematical foundations and computational algorithms to incorporate domain-specific physics principles and constraints into machine learning models, such as graph-based representations, multiresolution techniques, etc. The findings of this project will have direct benefits not only to the modeling of energetic materials, but also other extreme physics applications such as hypersonic vehicles, extreme weather modeling, and many others.