Dean’s Blog: Toward a Maturity Model for Research
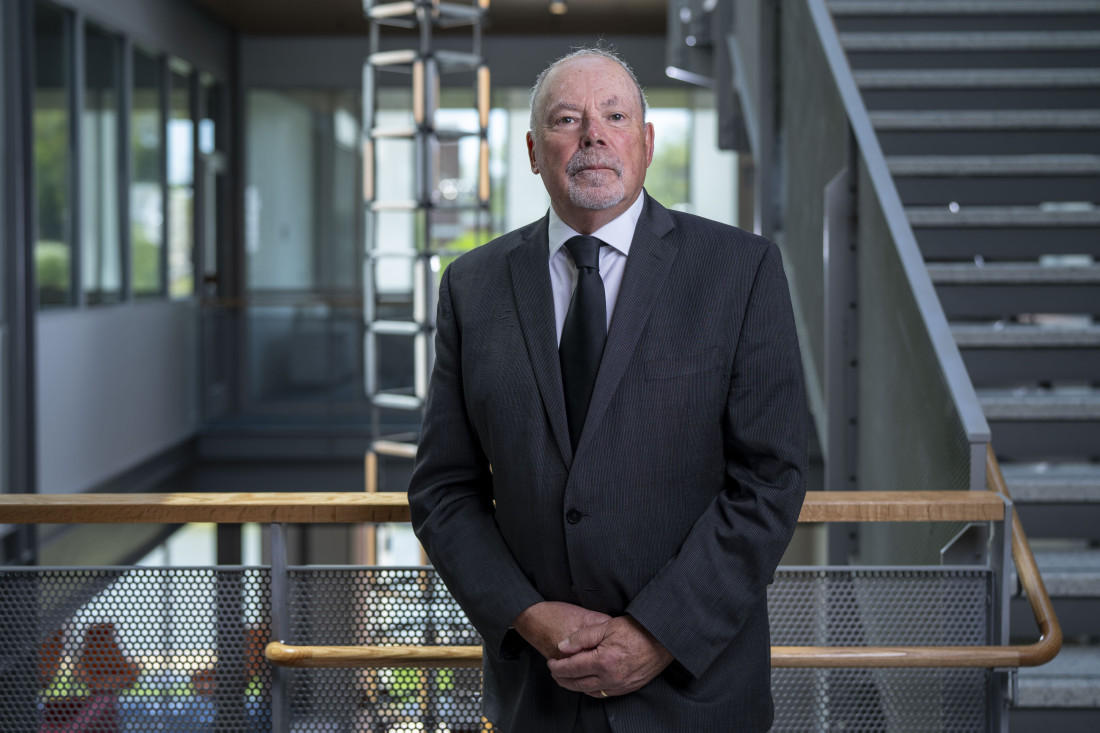
Periodically, I consider what I did today at work and map it to what I was actually trained to do. Typically about 25% has the benefit of prior training.
Recently wrapped up in the other 75% was thinking about how our School of Data Science at the University of Virginia is maturing. Don't get me wrong, we are far from having all the parts of a well-oiled machine working in unison, yet we are no longer in that frantic startup phase where the loss of one person could have meant we failed to deliver our programs, submit a grant, etc.
We are in a better place than where we were even a year ago. We have depth amongst our team, policies and procedures, a common view of data science, a shared culture, and a full complement of educational programs.
We are also following the mission to be “Great and Good” as a contributor to the University of Virginia through education, research, and service.
But here is the thing: I had at least a little training in launching a startup, just a couple of small companies, but have no training or experience taking an organization towards greater maturity. My typical approach when faced with a lack of experience is to read and then consult with those who are the experts.
I am not sure what comprises a successful maturity model, nor how to align it with our organizational goals, but it seems wise to look at prior success stories. Trolling through the literature with the help of ChatGPT, I came across Bell Labs as a model for how we might think about our research. No surprise there.
I had read Jon Gertner’s "The Idea Factory Bell Labs and the Great Age of American Innovation" long ago. What was new was the way ChatGPT summarized the success of Bell Labs and how it resonated with what we at the School of Data Science are trying to achieve.
I took the ChatGPT interpretation of why Bell Labs was successful and spoke to that success in terms (briefly) of what the School has accomplished and what we hope to accomplish. What follows are nine key points which begin to form a framework for what a School of Data Science maturity model for research might encompass. What needs to follow are maturity models for education and service, but let's start with research:
1. Culture of Innovation and Freedom: I would like to think this is what the School aspires to, but it is hard when a faculty member has to teach courses every semester. Yes, we often talk about academic freedom, but that implies the ability to freely express yourself, but only as much as time allows given your other duties. Freedom, as implied here, is confined to faculty research activities, which is only a part of their time and even then, for many, it is subject to the quirks of federal funding calls. We have created small internal seed funding to encourage researchers to collaborate around innovative ideas but it does not involve much freedom. Such is the nature of the academic enterprise. One approach would be to have a mixture of research staff and faculty, where the former are dedicated 100% to research innovation. But how to sustain that? Public-private partnership is worthy of discussion as we mature.
2. Interdisciplinary Collaboration: We are proud of our efforts here in terms of the cadre of faculty we have hired, the organizational structures we have created, the culture we have promoted, and the successes we have begun to see. We are a university within a university, and this matters. We have even secured funds to better understand our collaborative culture. Amplification comes next based on actions to our findings.
3. Long-term Investment: Typical federal funding cycles work so much against research, which is a long -term endeavor, it's enough to make a principal investigator weep. We are lucky that the School of Data Science has a significant endowment which assures (as far as we can tell) long-term sustainability of the organization. Currently, such endowments are mostly tied to endowed chairs. One option is to explore philanthropy and corporate partnerships that support the development of ideas rather than just people.
4. Attract Top Talent: We are doing our best here, and our amazing hires have bought into our collective vision of what higher education should be. Still, data science is a competitive market and we can't match private sector offers. The Bell Labs model hired new talent to work alongside Nobel Laureates so that everyone gave their best. We may not have Nobel Laureates, but we have a mix of senior, mid-career, and young investigators who can inspire one another. We need to establish a mentoring model that leverages this demographic spread.
5. Commitment to Research: This is a work in progress and will always be so. We have hired faculty not just for their ability to undertake groundbreaking research but also to teach the materials needed for undergraduate and graduate degrees in data science. Consequently, rather than specific research foci we have more of a spread. As the number of researchers grows, foci are starting to appear around specific societal needs —
6. Focus on Problem-solving: This follows from the argument for groundbreaking research and extends that notion to achieve a practical outcome. The School of Data Science already has a culture of support for translation and values practical application. We need to establish ways to reward translational research to provide encouragement when the reward system is dominated by traditional scholarly thinking.
7. Supportive Infrastructure: The School has minimal experimental research, and this is not likely to change. We will remain very much a computational enterprise and therefore need state-of-the-art research computing. This is more than hardware and software. It is people and the recognition of data as part of the complete research lifecycle, which includes, for example, consideration of data acquisition, visualization and dissemination, We do not have that presently, but by supporting our UVA leadership in a quest to improve the institution’s position through hires and appropriate hardware and software, the situation is improving. It is also an opportunity to further develop relationships with cloud providers through corporate partnership and that is on-going.
8. Recognition and Rewards: Internal to the school and to UVA we continue to celebrate the success of our team and its individuals, for example through teaching and research awards and endowed chairs. We have yet to turn our attention to outward-facing achievements such as faculty becoming fellows of societies, members of academies, and so on. Part of me sees these as a dated “old boy’s network” that is strewn with easily measured bias. Having said that, it is what I aspired to for a long time and it is a form of recognition our faculty seek. We need to be more intentional and systematic about how we move forward. Awards beget awards, and we are behind the curve.
9. Adaptability: Last and certainly not least must be our ability to change to meet new scientific and technological landscapes. Never has this been more evident than with the rise of large language models. They have changed how society thinks and works in unprecedented ways that we are only just beginning to grasp. We have engaged in a Futures Initiative to try and get out in front of what breakthroughs are coming, as hard and unpredictable as that is. Attempting to be proactive rather than reactive is a first step. As we adapt we need honest and effective evaluation methods, which themselves will change over time, to reward what is most meaningful for a robust research enterprise. These nine points are certainly not a maturity model for research, but I am hopeful they prompt a discussion on a model for the School of Data Science and how we would implement it. Once some consensus arises around a maturity model for research similar models need to be developed for education and service (expect further blogs), as the three pillars of higher education organization. With these in hand we need to revisit our strategic plan to determine what changes are needed.
Acknowledgements: Thanks to Don Brown, senior associate dean for research at the School of Data Science, for valuable input and leading our research efforts.