Beyond Moneyball: Phillies Data Scientist Give Students a Real-World Look at How Today’s MLB Teams Use Data
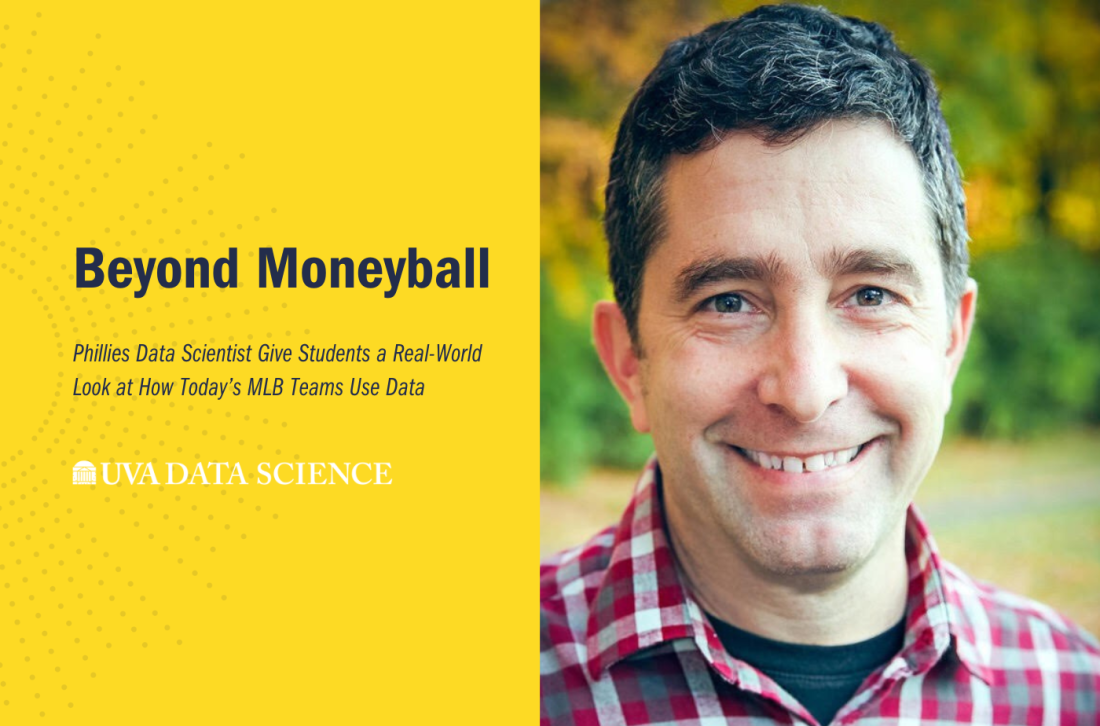
You’ve probably heard of Moneyball, the 2011 film starring Brad Pitt that made baseball analytics famous. But do you know how much the field has evolved since then?
Last month, Chris Fonnesbeck, principal quantitative analyst in Baseball Research & Development for the Philadelphia Phillies, walked UVA School of Data Science students through some of the latest advances in baseball analytics, referred to as sabermetrics. His presentation illustrated how data science informs decisions in nearly every facet of the Phillies and other Major League Baseball organizations, from draft decisions and player development to in-game calls.
As pervasive as it is, Fonnesbeck, who has also worked for the Brewers and Yankees and is an adjunct professor at Vanderbilt University, noted that jobs like his can be “a little bit opaque” since most clubs don’t want to share their secrets. Philadelphia, however, authorized Fonnesbeck to give his talk at UVA.
“I won’t give away all of our trade secrets,” he said. “But I want to give you a useful, high-level overview of what goes on inside a baseball team in 2023.”
Fonnesbeck went through a detailed review of some of the metrics that his team focuses on. For example, the team runs models to predict expected runs in a specific set of circumstances, such as a man on first with two outs, or a runner on second with no outs. The models are based on a sample size of more than 40,000, and can help teams predict what might happen in an inning and how to best score or avoid runs, depending if they are batting or fielding.
This model is why many teams have moved away from sacrifice bunts, which Fonnesbeck said are almost always a bad idea statistically. When looking at run values by event, a home run is worth 1.397 runs on average, while and a sacrifice bunt has a -0.096 run value.
“We don’t see that as much in baseball anymore,” he said, “because being on first with no outs is better than being on second with no outs most of the time.”
There are also metrics tracking each player’s value to the team. These measures are more precise than traditional batting averages, which track the number of hits someone gets but excludes things like walks, which still put a man on base. Now, organizations track player’s weighted on-base average, which assigns a value to each possible event when a player is at the plate, from a home run to an out and everything in between, and then divides that by the number of opportunities a player has. It is considered a good measure of a player’s overall offensive value.
Another metric, the on-base percentage plus slugging percentage (OPS) – weights home runs more than singles or doubles. That measure can be put on a percentage scale, where 100 is the league average. A player like Aaron Judge, who was the home-run leader last year, has a 200 OPS score, meaning that he was a full 100% above the average player.
“That doesn’t happen very often, only for MVP candidates, All-Star types,” Fonnesbeck said. “But you can get a good sense of what a player’s production is relative to somebody else in the league.”
On the flip side, Fonnesbeck also discussed analyzing pitchers with a metric called fielding-independent pitching (FIP). Traditional measures of pitching performance, such as earned run average (ERA) do not differentiate between pitching and plays made in the field. A runner might score after a fielding error, but that run would still count against the pitcher’s ERA. Data scientists developed the FIP equation to focus more closely on pitching.
Fonnesbeck talked students through the specifics of these and other metrics, even discussing how his team accounts for external factors such as the size of different ballparks, which can make home runs more or less likely.
Then, he moved on to player value. One metric is wins above replacement (WAR), which isolates how much a player’s production has contributed to wins for that particular team when compared with a replacement player. Fonnesbeck defined a replacement level player as the expected production from a player who could be freely acquired and signed for the league minimum salary.
“WAR is not a summary statistic so much as it is a model output,” he said. “This is where you have a much more complex model that summarizes the entirety of a player’s contribution – hitting, pitching, defense, everything.”
Citing some examples, Fonnesbeck said that an MVP candidate like Manny Machado, who plays for the San Diego Padres, has a 6+ WAR. A superstar, such as Houston Astros third baseman Alex Bregman, has a 5-6 WAR; an All-Star might have a 4-5 WAR. Good players or “solid starters,” he said, might range from 2-4 wins above replacement, while role players or replacement players are less than 2.
Among the lifetime leaders in WAR are Babe Ruth, Walter Johnson, Cy Young, Barry Bonds, Willie Mays, Ty Cobb and Hank Aaron.
Metrics like WAR and other predictive metrics, such as surplus value, help baseball organizations predict a player’s value to the organization with more accuracy than previously possible. This has contributed to players locking in longer contracts earlier in their careers, Fonnesbeck said, when their predicted value is high even if they do not have a long record of excellence yet.
He pointed out Atlanta Braves outfielder Ronald Acuña, Jr. as one example. Acuña, who is in his early twenties, signed an eight-year, $100 million contract in 2019.
“Teams are using this approach to lock these players up for large amounts of money, kind of placing a bet on the player that they could turn out to be a star,” he said. “It’s a lot of money now, but it is not as much as they might spend if they waited for those players to establish themselves as stars and then had to sign them.”
“In the past, you would sign players based mostly on what they had done, rather than what they were expected to do,” he said.
Fonnesbeck also cautioned students to be wary of some of the stats broadcasters flash on the screen during televised games. Just because we now have the machines to measure and predict many different factors doesn’t mean those tools are always applied accurately. As an example, he cited Apple TV’s in-game probability feature, which purports to show the probability of a player reaching a base throughout his at-bat. An article on Fangraphs walked readers through one at-bat, questioning Apple TV’s predictions.
“Be a little bit apprehensive, when you see those machine learning tools applied,” Fonnesbeck said. “These tools can be misapplied in baseball, and I am sure there are teams misapplying data as well.”
He closed by reminding students that the Phillies are always looking for talented data scientists.
“I was allowed to come here today partly as outreach from the Philadelphia Phillies,” he said. “There are a variety of opportunities within the Phillies, both within baseball operations and elsewhere… we are always looking for talented quantitative folks.”