Accurate forecasts of U.S. Presidential elections are not only central to political journalism, but are used by campaigns to formulate strategy, impact financial markets, and aid businesses planning for the future.
However, evidenced by the 2016 and 2020 elections, forecasting the election remains a challenging endeavor. Our review of methodologies revealed three discrete approaches: polling-based, demographic and economic fundamentals-based, and sentiment-based. We sought to identify which advantages each approach offers. We built on past research to adopt a novel forecast model that combines a weighted average of a hierarchical Bayesian fundamentals model and a Bayesian polling model.
Forecasting election results has grown in popularity with each election cycle, and particularly after the 2012 election when models such as Nate Silver’s were very accurate. However, after the 2016 and 2020 presidential elections, problems with the accuracy of polls have called the soundness of these forecasts into question. We sought to explore how well some of these forecast models would do in the 2020 election and which approach has the most promise for future forecasting. In particular, we compared forecasts based entirely or mainly on polls with others that rely on “fundamentals”: economic or demographic data that may predict how a state or region will vote.
Additionally, we created our own model that combines both polling and fundamentals with the goal of finding out whether these approaches can shed light on how voters decide elections. Much of the research that will discover why the polls were overconfident in a Biden victory has yet to be done, but it’s apparent that if the polls cannot be consistently accurate in the future, the popularity of polling-based models may fade and be replaced by something else. Our own model combined a Bayesian polling-based model with a Bayesian hierarchical model using only fundamentals, and we found that the fundamentals model did better on its own due to inaccurate polls. While fundamentals-based models have their own flaws, our research demonstrates that forecasters may need to find novel ways of using this data to improve the accuracy of their predictions.
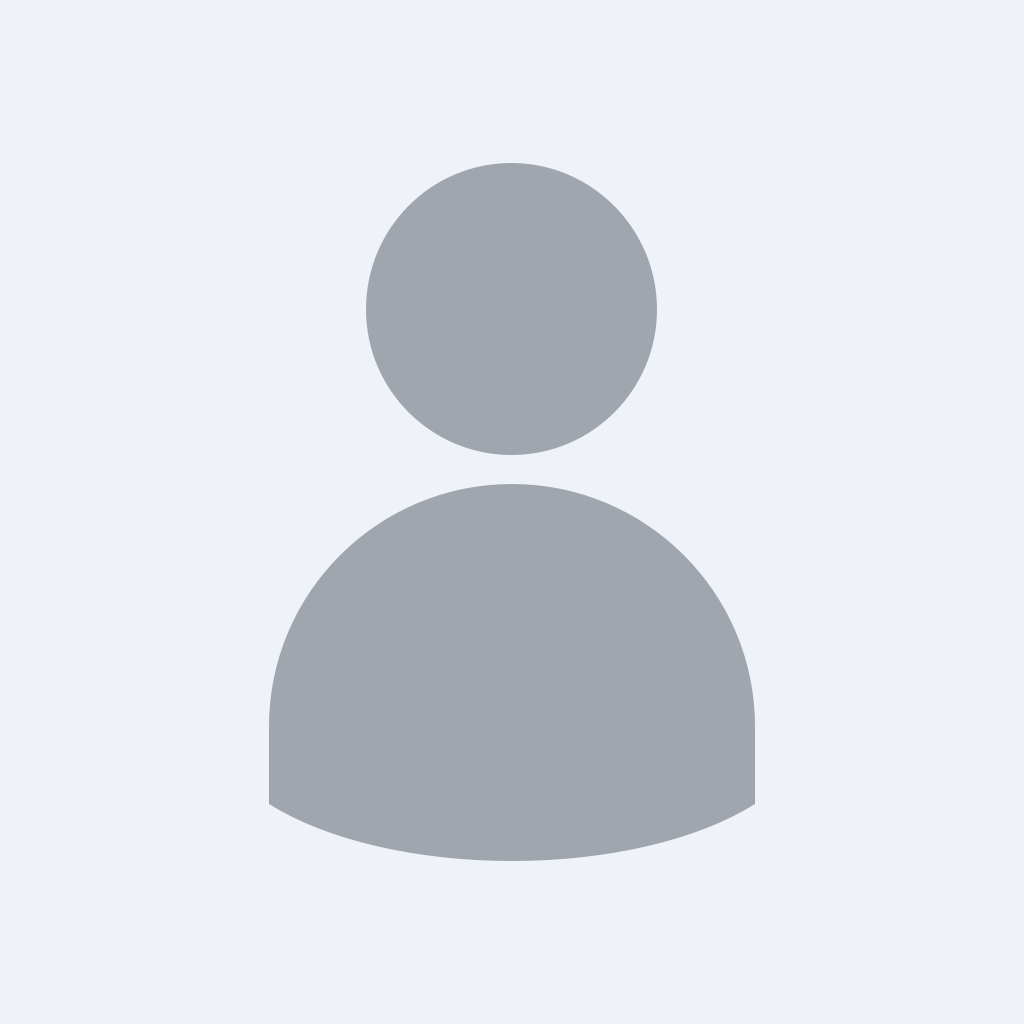
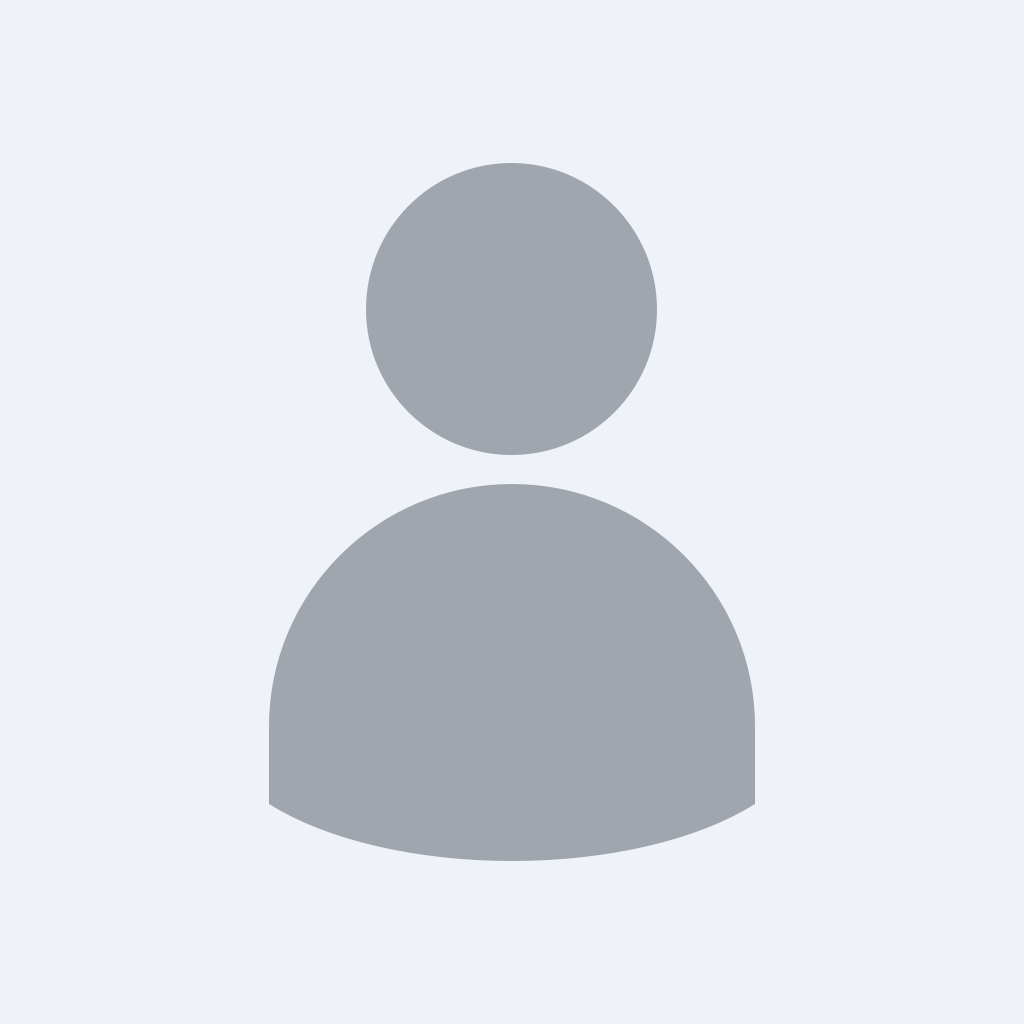
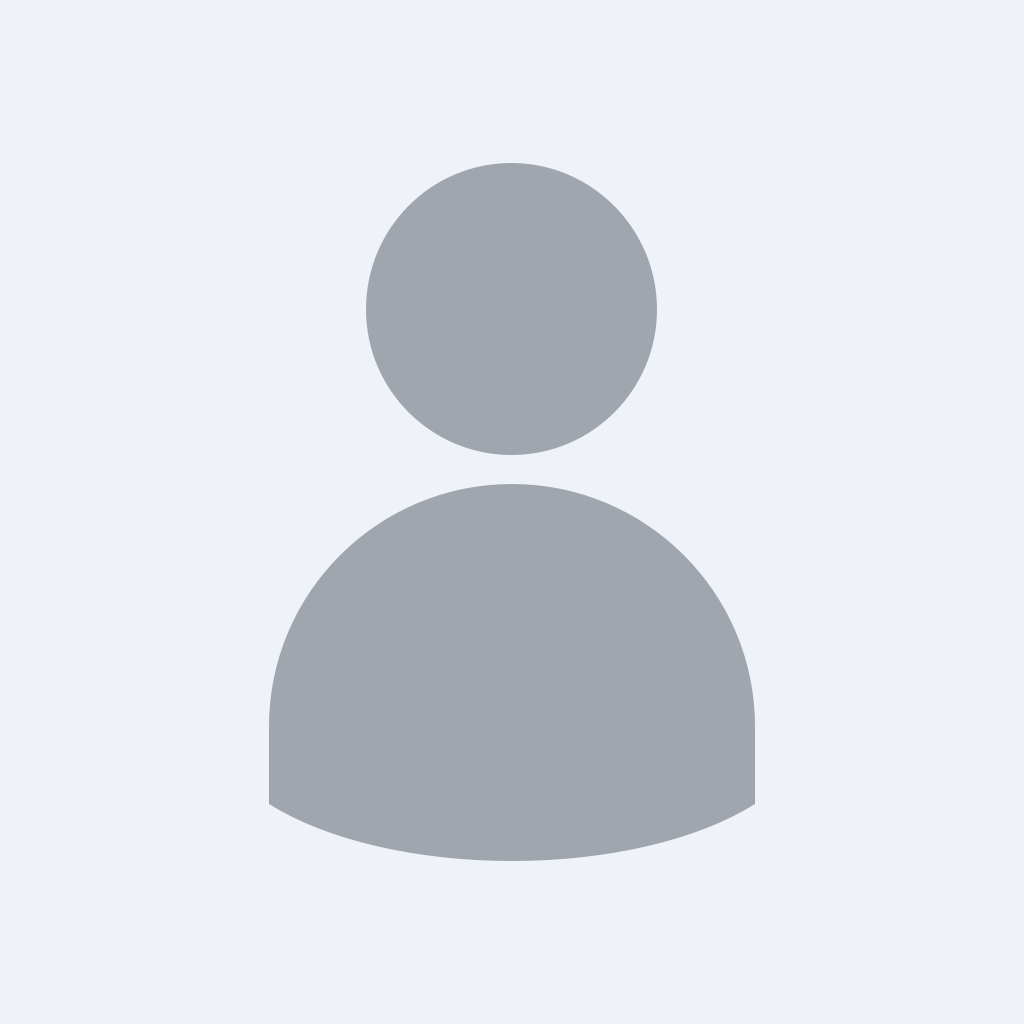
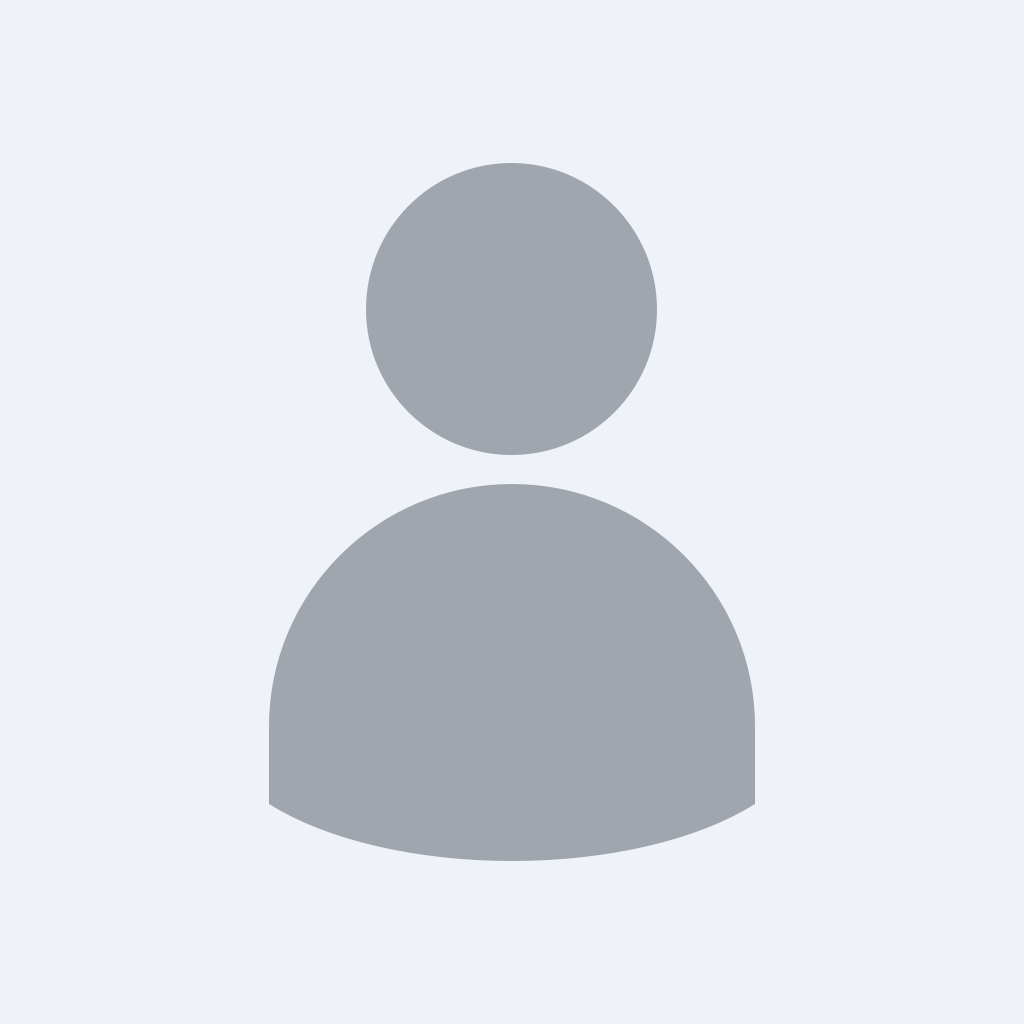