This project will seek to provide accurate ecological forecasts by using different approaches for predicting algal blooms.
Algal blooms are a widespread problem that impact drinking water, recreation, and industry around the world, and locally in Virginia. By using data from high-frequency water quality sensors and comparing process-based and machine learning models, researchers Cal Buelo (Environmental Sciences) and Neda Nazemi (Engineering Systems and Environment) will study which methods are most accurate in individual aquatic ecosystems and look for patterns across different systems.
Hopefully, near-term ecological forecasting knowledge combined with increasingly affordable and easy-to-use automated sensors will allow water resource managers to adopt proactive strategies to minimize negative impacts from algal blooms.
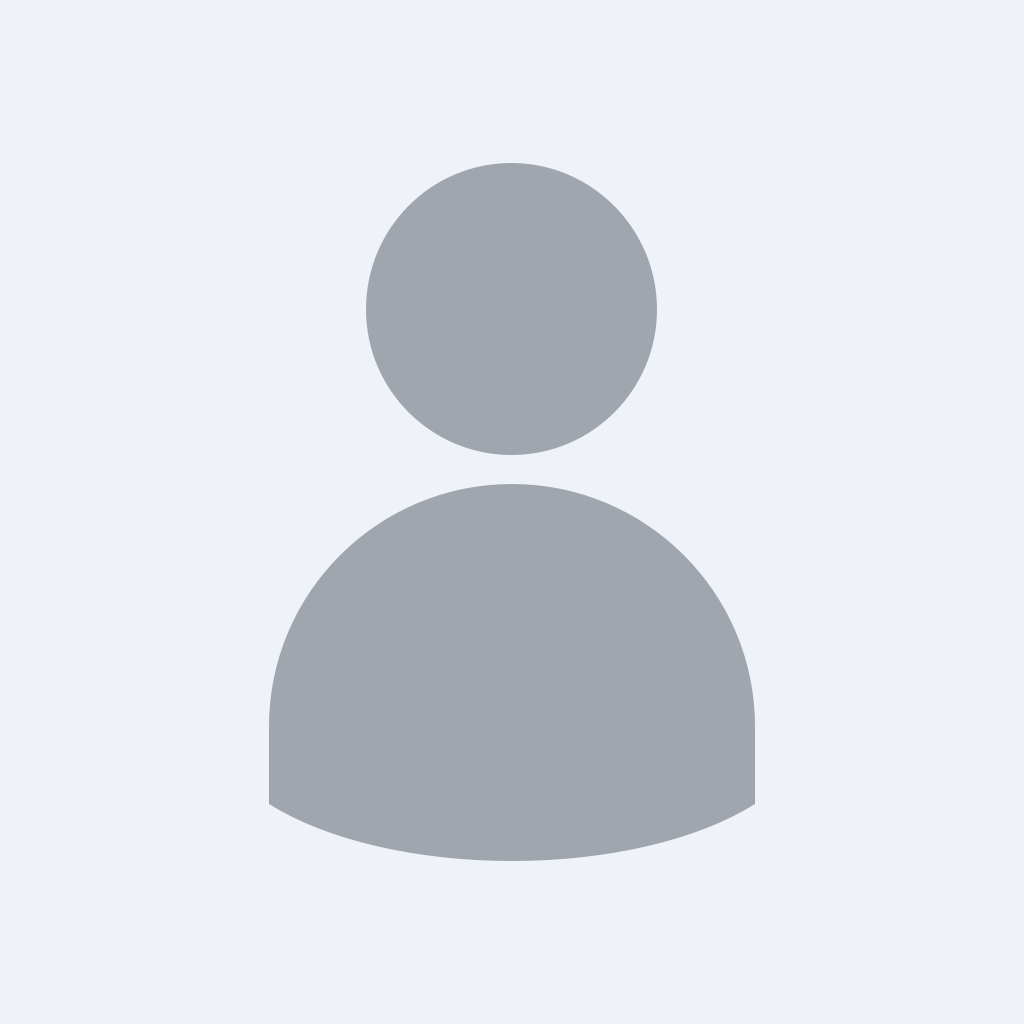
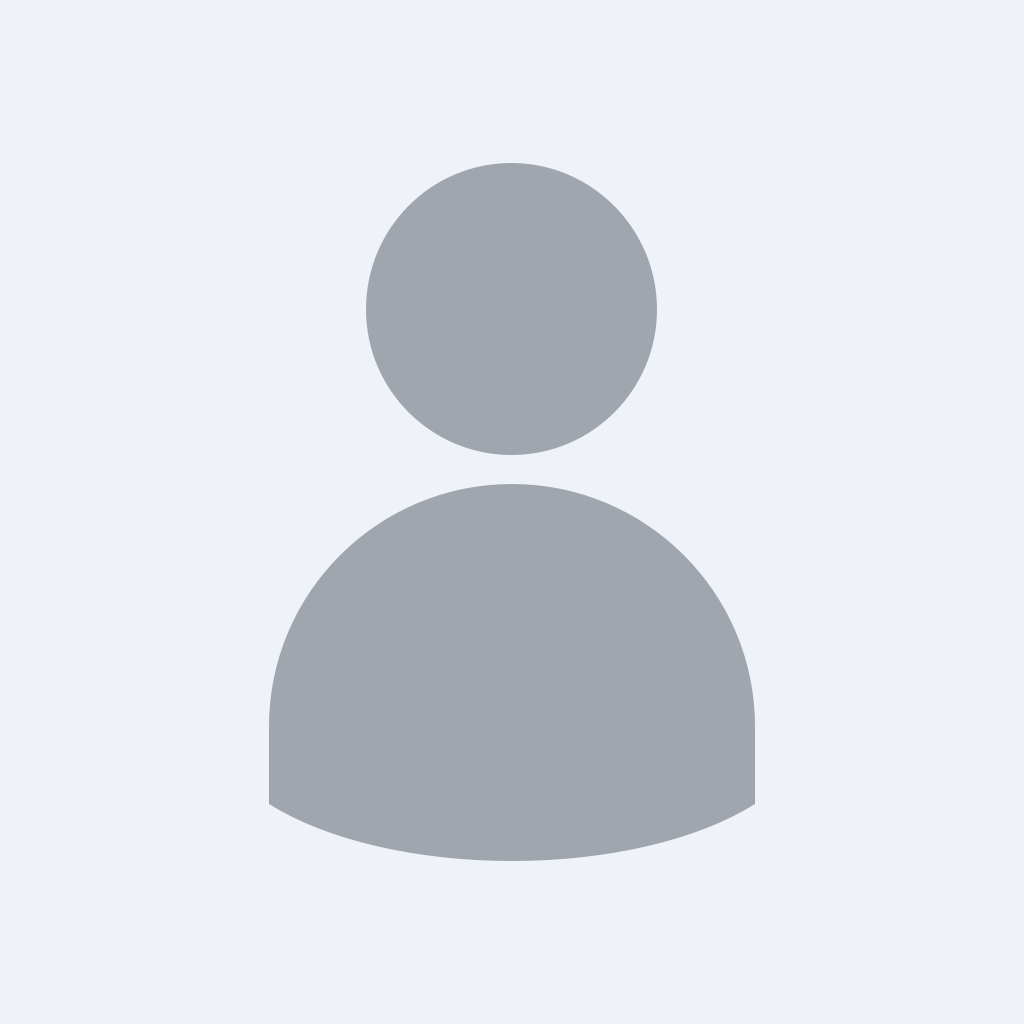