Motor vehicle crashes cause loss of life, property and finances. Vehicle accidents are a focus of traffic safety research, uncovering useful information that can be directly applied to reduce these losses.
Traditionally, modeling crash fatalities has been done using machine learning techniques, considering crash level variables, such as roadway characteristics, lighting conditions, weather conditions and the prevalence of drugs or alcohol.
This research, conducted by MSDS students Lulu Ge, Tyler Hutcherson and Qi Tang, moves in a new direction by incorporating heterogeneous data sources, including both police crash report data and demographic data, potentially leading to a heightened understanding of the relationship between crash location and the likelihood of a fatality.
In order to utilize multiple data sources, they used a mixed linear modeling technique that enabled data fusion in a principled way to build a better predictive model. They integrated these data sources to analyze the natural clustering of events in space by different geographic levels.
Their findings indicate that using mixed effect logistic regression, incorporating both fixed and random effects, outperforms a traditional classification model in its ability to predict crash fatalities across the Commonwealth of Virginia.
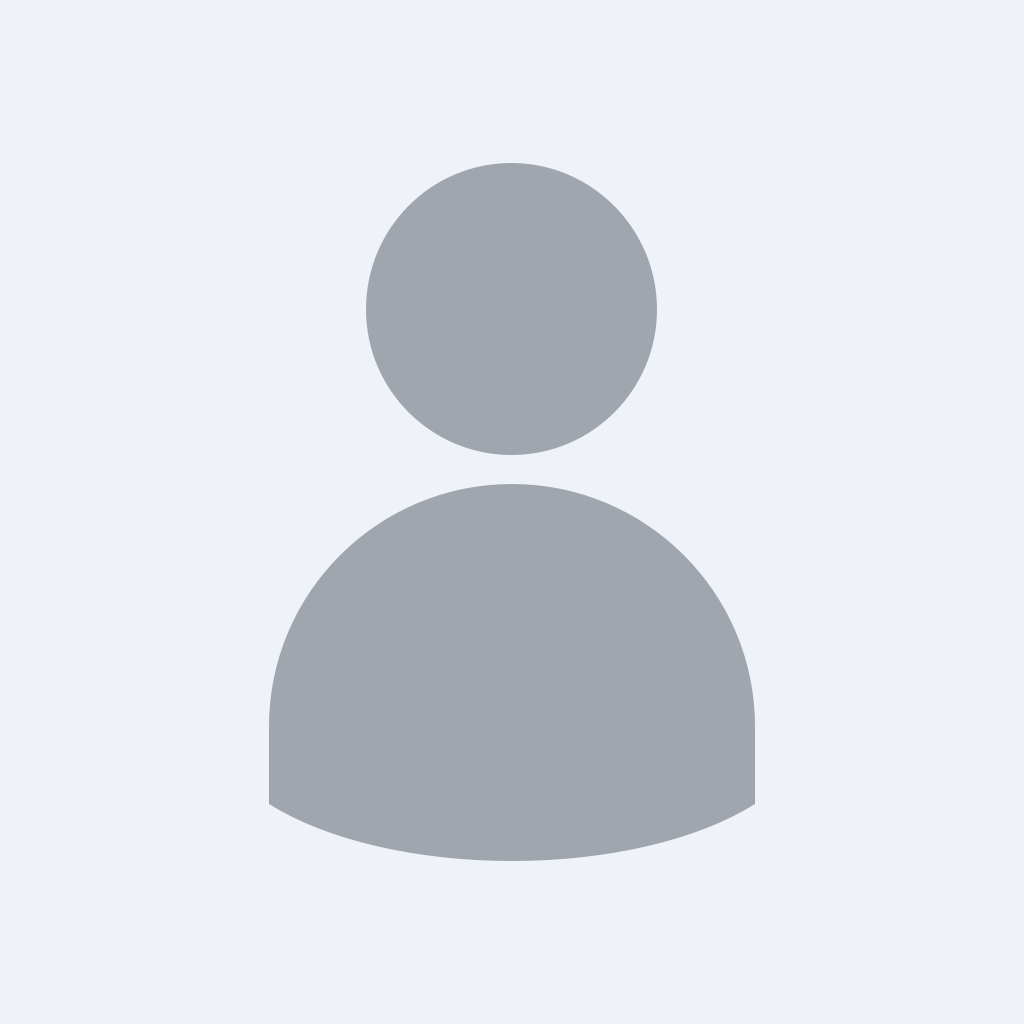
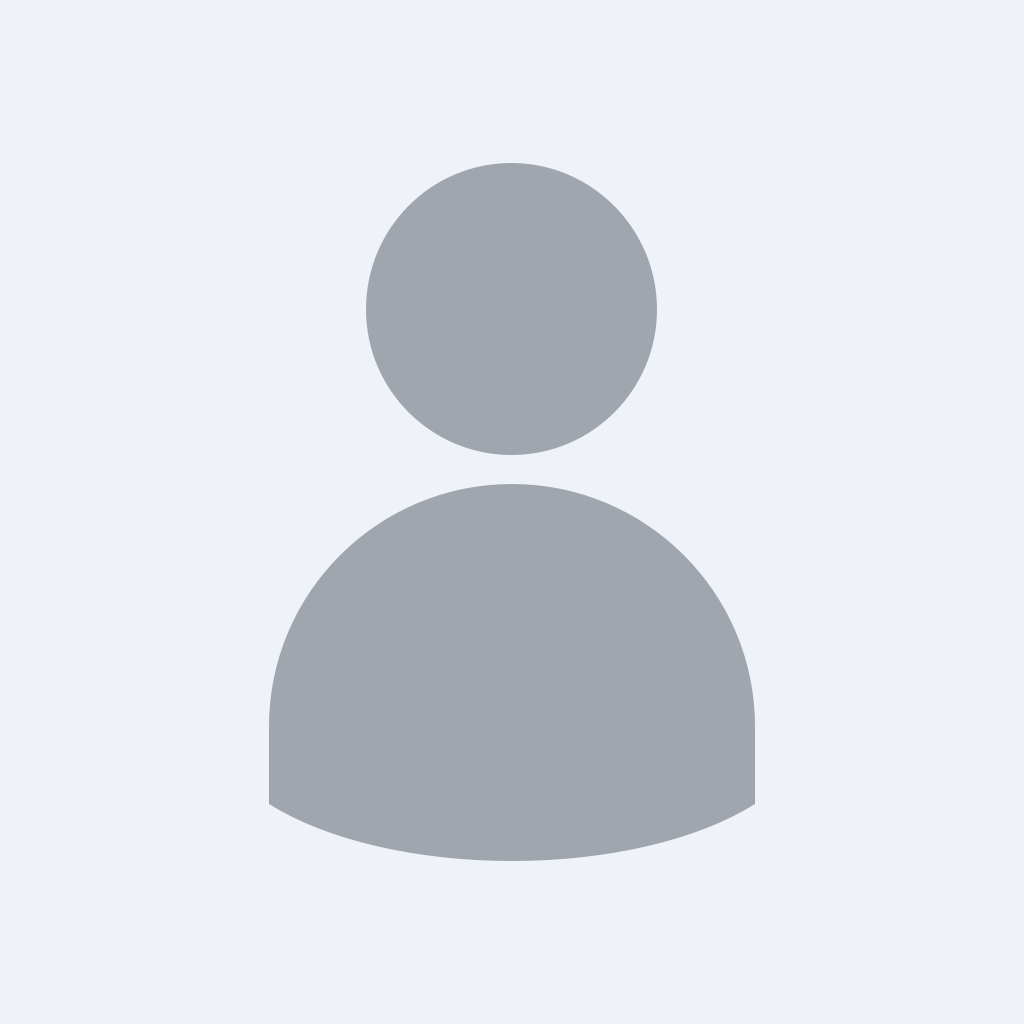
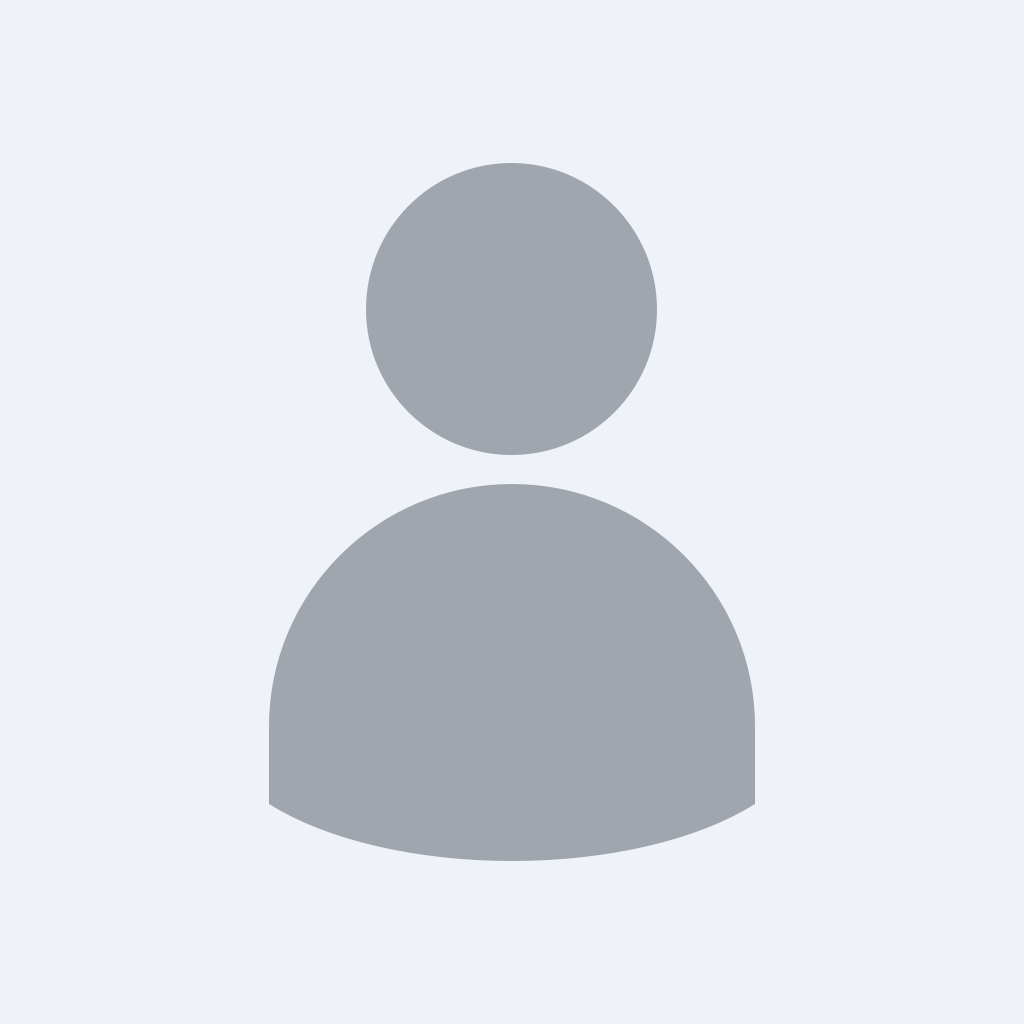