Why Gianluca Guadagni Is So Excited for the Future of Data Science
Gianluca Guadagni likens the current moment in data science and mathematics to the excitement around the invention of the steam engine in the 1700s.
“The steam engine was wonderful, and it seemed that you could do anything with it, but nobody understood the best way to do that,” he said. That curiosity – all of those unanswered questions about how to make transportation faster and more efficient – led to a revolution in the field of thermodynamics.
Today, we are on the cusp of that revolution in machine learning and mathematics – at the dawn of a massive technological shift and searching for ways to harness its power for good.
“We need to capture it, we need to create a framework where we can talk about it, we need to understand the limits,” Guadagni said. “We need to understand efficiency and we need to share that knowledge.”
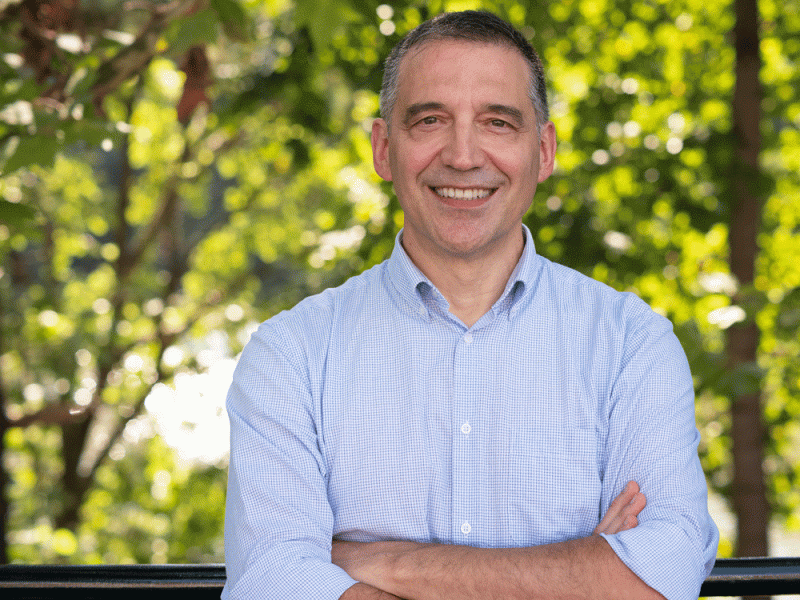
Guadagni studied physics and mathematics earned a doctorate in mathematics from UVA in 2004 before returning to Italy to teach in the School of Engineering at the University of Rome “Tor Vergata”. By 2006, he was back on Grounds teaching first in the Department of Mathematics, then in School of Engineering and Applied Sciences and now in the School of Data Science.
Much of that journey, Guadagni said, was driven by his desire to understand complex systems that we often take for granted, specifically thermodynamic systems.
“You can think of a magnet, basically, as a crystal, and a crystal is made of a lattice. At each intersection of this lattice, we think there are atoms or molecules, which interact with each other,” Guadagni said. “And so, these are huge systems, very complex, and almost impossible to know.”
Temperature is a good example, he said. Temperature is not determined by any one molecule; individual molecules do not have a temperature. Instead, temperature is a measure of the combined vibrations of all of the molecules in a system, such as the human body. Those vibrations generate energy, which we feel as heat. Magnets are another one – when a magnet is drawn to something, every molecule in that magnet aligns in the same direction.
“Then, when you change some external condition, such as the external temperature or pressure, these systems can flick to a completely different state,” Guadagni said. Water freezes or boils. Magnets are drawn to metal.
“While it’s natural to us, what is really happening is something incredible,” Guadagni said. “Those are problems I have always been fascinated with, thinking about how we can really see and understand extremely complicated systems like this, with huge numbers of atoms and molecules that somehow have this coordinate behavior.”
Data science and machine learning offer new ways for Guadagni to peer into these age-old systems – the next evolution in centuries of mathematics and physics knowledge. It can create more complex, and more accurate, digital models than ever before, getting us ever closer to being able to model, for example, how a protein is formed or how the neural networks in our brain fire.
“We are far from understanding it all,” he said. “There is a lot that needs to be done, and models that need to be changed.”
That humility is something that Guadagni works to instill in his students at UVA, where he received a UVA Student Council Teaching Award in 2022.
We might have more powerful machines than ever, he notes, but we still have to take the time to get the work right – to not just plug values in and watch the algorithm chug along, but to understand what we are doing and why. Big companies – the Googles of the world – want to see results and to have the AI tools that make the news. And, they want to do in a few hours what could be done, more painstakingly, over several months.
“Eventually it is going to become too expensive,” Guadagni said, citing a talk he recently attended about the energy used by massive machine learning models – projected to reach in few years roughly the same amount of energy needed to power a small country.
“What are the ethical complications of doing it that way,” he asks. “Efficiency is not just something for mathematics. Inefficiency has a social cost. If you are not efficient, you are wasting resources that could be used for something else.”
The 1700s offer another lesson for this dilemma. As engineers, physicists and scientists began to understand the steam engine, they didn’t just improve their knowledge of thermodynamics. They shared that knowledge widely.
“That kind of knowledge was aristocratic at that time – only those who could afford it could get it – but they did share it,” Guadagni said.
Today, teaching and research can help to move that knowledge out into the public sphere, rather than keeping it with one company or organization. That is why Guadagni does what he does. Every day, he can learn more about the systems that have always fascinated him and share that knowledge with students and peers. Together, they can create a network with just as much power, moving toward a more efficient and equitable future.