Can You Trust Artificial Intelligence? See How Sheng Li is Building Trustworthy Technology
If you were to look out a window right now, your brain would answer a series of questions pretty quickly. Let’s say you are at home looking out at your street. Does everything look as it usually does? Is anything out of place? If there are two people walking a dog nearby, your brain would catalogue them too. Do you know them? What do they look like? Do they look friendly?
We make these assessments on a daily basis, almost without thinking – and often with our biases or assumptions baked in. Data scientists like Sheng Li imagine a world where artificial intelligence can make the same connections with the same ease – and crucially – without the same bias and potential for error.
Li, an artificial intelligence researcher and assistant professor in the University of Virginia School of Data Science, directs the Reasoning and Knowledge Discovery Laboratory. There, he and his students investigate how to improve deep learning to make AI not only accurate, but trustworthy.
“Deep learning models are usually implemented by deep neural networks with multiple layers, which are able to get latent features from large scale data,” Li said. “Those features could benefit many downstream applications, such as computer vision, natural language processing, recommender systems, or bioinformatics.”
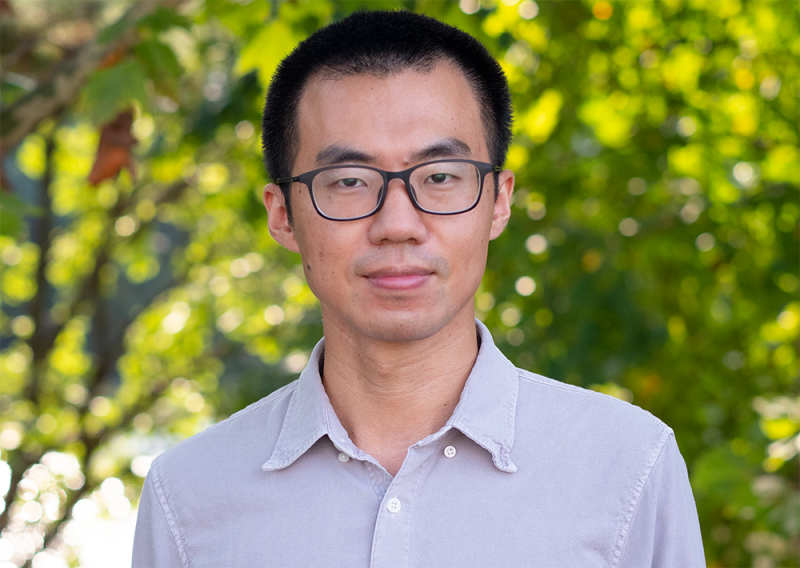
Li first became interested in artificial intelligence when he was selecting university programs in China, where he earned a bachelor’s degree in computer science and a master’s degree in information security from Nanjing University of Posts and Telecommunications before pursuing a Ph.D. in computer engineering at Northeastern University. One of his undergraduate professors was working on image processing and facial recognition. Li began studying under him, eventually publishing his first research paper in 2008.
“We were working on facial recognition and palmprint recognition. We designed mathematical models to extract features from images to recognize the identity of the person,” Li said. “Now, when I see the paper, our model seems very simple, but at that time it was very cool to me.”
As he pursued his Ph.D. and went to work with Adobe Research on deep learning, Li, his advisor and his colleagues learned more and more about how to solve problems with facial recognition, such as occlusion or “noise” in images that might prevent an algorithm from accurately recognizing something or someone. Li’s first book, “Robust Representations for Data Analytics,” tackled that topic and others with his Ph.D. advisor Yun Fu.
Ultimately, Li came to focus not just on the accuracy of artificial intelligence models, but on their trustworthiness, such as fairness, robustness, explainability, and transferability.
“When we apply machine learning in reality, we must take those things into account as well,” he said. “Is it fair? Is it robust? Is it accountable?”
Several recent studies, for example, have shown that many artificial intelligence algorithms do not perform as well when faced with non-white subjects. One recent study found that facial recognition technology misidentified Black or Asian faces 10 to 100 times more often than white faces and falsely identified women more often than men. This could have very serious real-world consequences if security software falsely identifies someone in a criminal investigation or if verification software repeatedly failed to recognize someone.
To account for that, “we can mitigate the facial recognition bias by incorporating fairness constraints,” Li said. “In addition to looking at overall accuracy across the population, we can also look at accuracy for individual groups, defined by sensitive attributes such as age, gender or some racial groups.”
Li and his lab are also focused on another potential minefield: how to teach AI models that “correlation does not imply causation,” as the saying goes. Let’s say the dog outside your home started barking. You might quickly realize that the dog is barking at a squirrel that darted across its path. An AI algorithm would not know if the dog was barking at the squirrel, at you, or at another, unseen threat.
“As humans, we can reason through something and think about what the true cause of a phenomena is,” discarding factors that are merely correlations, Li said. “To teach a model a similar thing is very, very challenging.”
Statisticians have studied a similar problem for decades, Li said, but machine learning research has turned its attention to causal inference over the last ten years. Li’s group has been studying the problem for about eight years, with several publications and tutorials done and a book, which Li co-edited, publishing next year.
“We are working on teaching the machine to not only look at and recognize the different objects but infer pairwise relationships and detect anomalies in a trustworthy way,” he said. He named several use cases, such as increased accuracy and trustworthiness in surveillance systems.
It is a challenging goal, but an exciting one as well, if scientists can in fact equip AI to make better decisions.
“Trustworthiness is the major focus of my lab,” Li said, because “these algorithms could ultimately help us make better decisions in an open and dynamic environment."